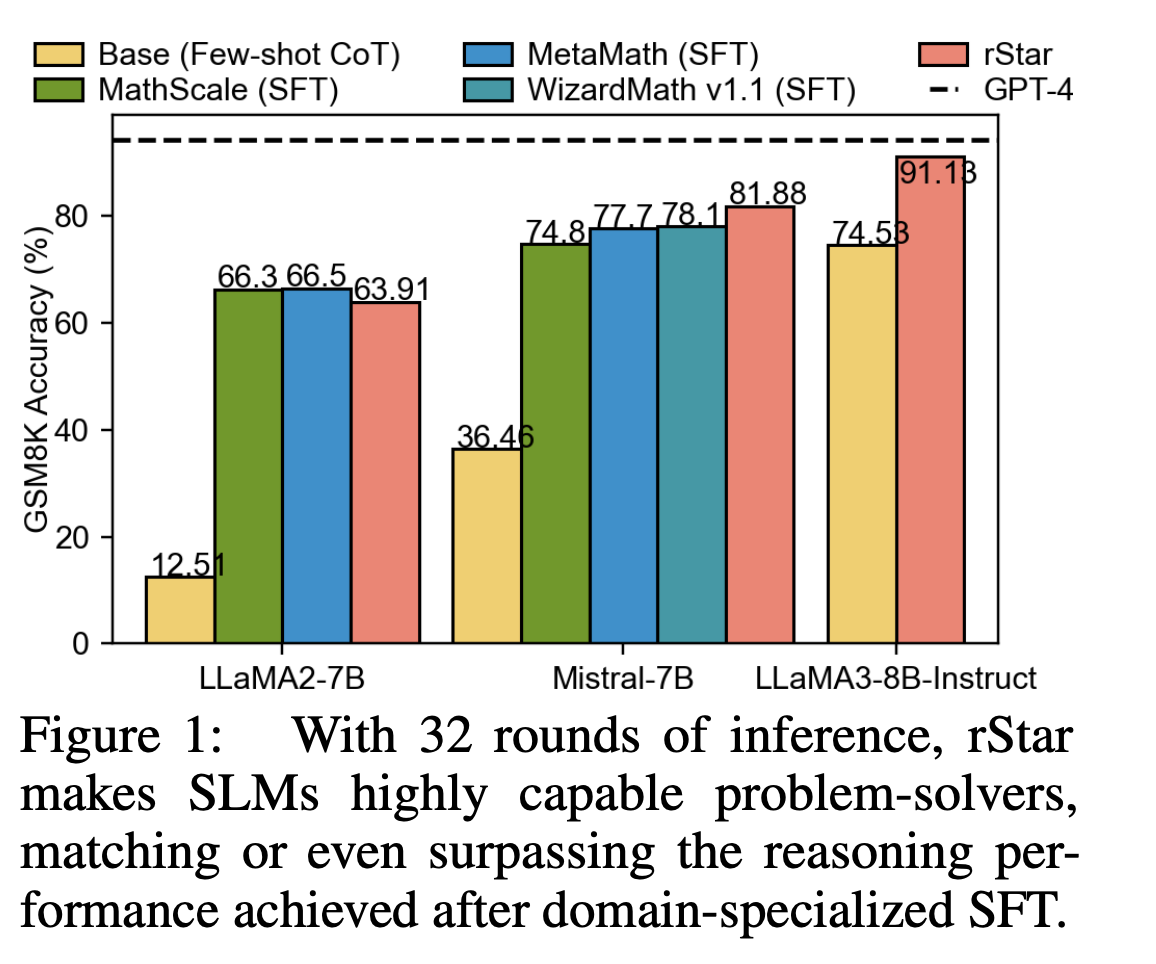
Practical AI Solutions for Enhancing Small Language Models’ Reasoning Capabilities
Introduction
Large language models (LLMs) face challenges in complex reasoning tasks, but practical solutions are being developed to enhance the reasoning capabilities of smaller language models (SLMs) without relying on fine-tuning or superior models.
rStar Approach
Researchers have introduced the Self-play muTuAl Reasoning (rStar) approach, which employs a unique self-play mutual generation-discrimination process to enhance SLMs’ reasoning capabilities during inference.
Key Features of rStar
rStar uses a conventional Monte Carlo Tree Search (MCTS) algorithm to self-generate multi-step reasoning solutions and introduces a rich set of human-like reasoning actions. It also implements a carefully designed reward function and a discrimination process called mutual consistency using a second SLM as a discriminator.
Effectiveness of rStar
rStar has demonstrated significant improvements in reasoning accuracy across various tasks and models, outperforming existing methods in both accuracy and efficiency. It has also shown state-of-the-art performance in diverse reasoning benchmarks and language models.
Practical Implementation of AI in Business
AI solutions can redefine business processes and customer engagement by identifying automation opportunities, defining measurable KPIs, selecting suitable AI tools, and implementing AI usage gradually.
Connect with Us
To explore AI solutions for your company or receive AI KPI management advice, connect with us at hello@itinai.com. Stay updated on leveraging AI by following us on Telegram and Twitter.