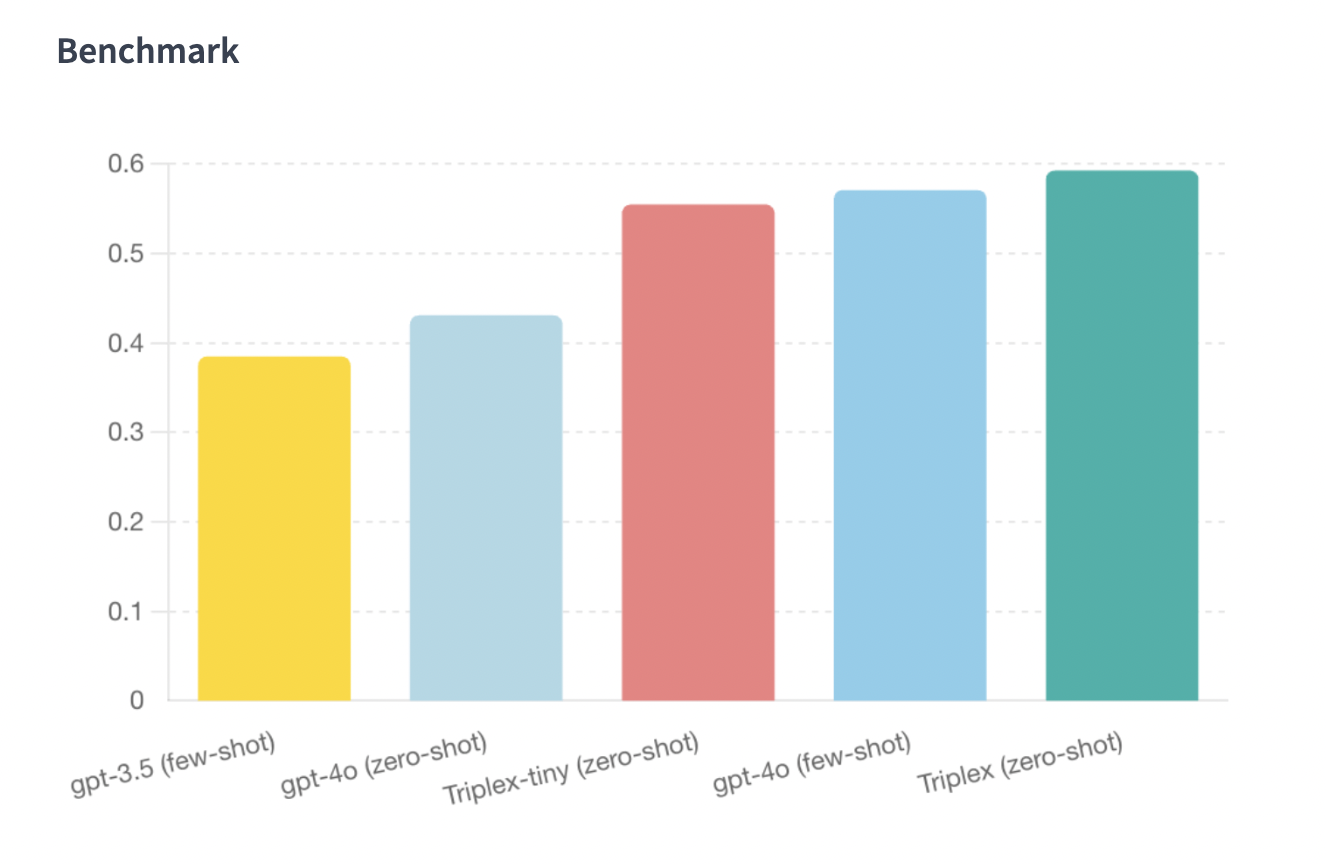
SciPhi Open Sourced Triplex: A SOTA LLM for Knowledge Graph Construction Provides Data Structuring with Cost-Effective and Efficient Solutions
Introduction
Recent release of Triplex, a cutting-edge language model designed for knowledge graph construction, promises to revolutionize the conversion of unstructured data into structured formats. This open-source innovation significantly reduces the cost and complexity traditionally associated with this process and is set to become a key tool for data scientists and analysts seeking efficient, cost-effective solutions.
Practical Solutions and Value
Triplex efficiently constructs knowledge graphs, providing a tenfold reduction in cost compared to traditional methods. It achieves this by converting unstructured text into foundational elements called “semantic triples.” The model also demonstrates superior performance in cost and accuracy compared to advanced models like GPT-4o, making it a practical and efficient solution for various applications.
Performance and Training
Triplex’s exceptional performance is attributed to its smaller model size, capability to function without extensive few-shot context, and additional training using Dynamic Programming Optimization and Knowledge Triplet Optimization. This extensive training on diverse datasets ensures the model’s versatility and robustness across various applications.
Immediate Applications
One immediate application of Triplex is local knowledge graph construction using the R2R RAG engine in conjunction with Neo4J, which is now more accessible thanks to the efficiencies introduced by Triplex.
Conclusion
SciPhi’s release of Triplex dramatically reduces the cost and complexity of converting unstructured data into structured formats, promising to enhance the efficiency of existing processes and make advanced data representation techniques accessible to various applications and industries.