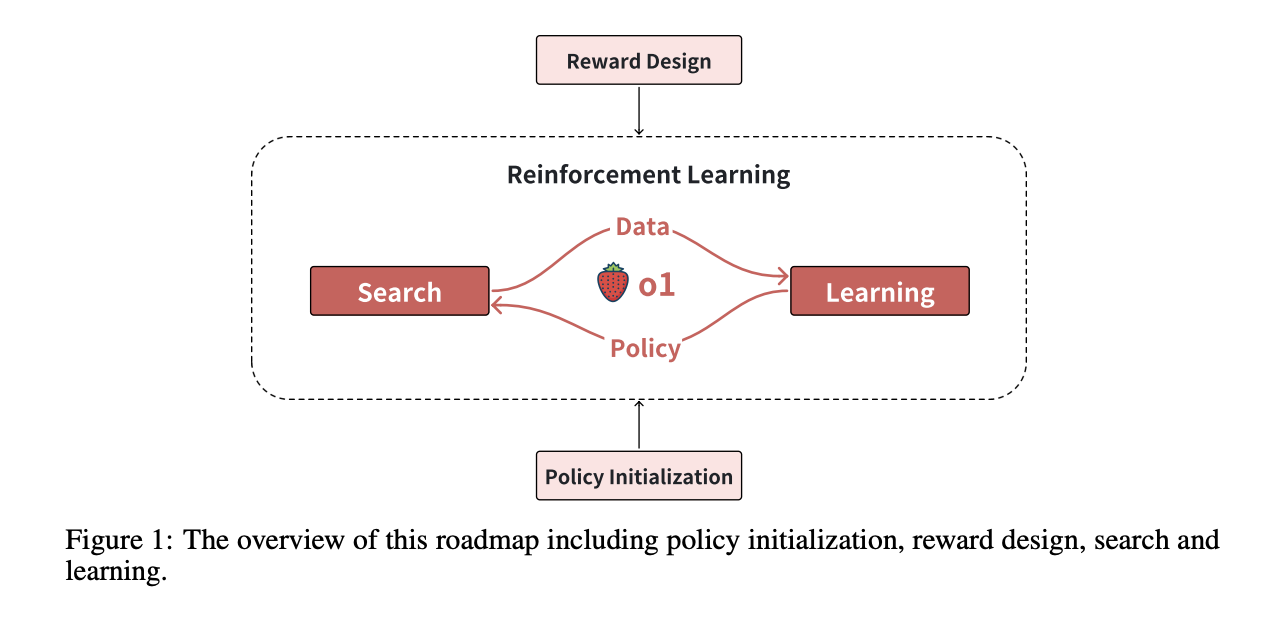
Challenges in AI Reasoning
Achieving expert-level performance in complex reasoning tasks is tough for artificial intelligence (AI). Models like OpenAI’s o1 show advanced reasoning similar to trained experts. However, creating such models involves overcoming significant challenges, such as:
- Managing a vast action space during training
- Designing effective reward signals
- Scaling search and learning processes
Current methods, like knowledge distillation, have limitations based on the teacher model’s performance. This emphasizes the need for a structured roadmap focusing on:
- Policy initialization
- Reward design
- Search
- Learning
The Roadmap Framework
A team from Fudan University and Shanghai AI Laboratory has created a roadmap for reproducing o1 using reinforcement learning. This framework highlights four essential components:
1. Policy Initialization
This involves pre-training and fine-tuning models to perform critical tasks like:
- Decomposition
- Generating alternatives
- Self-correction
2. Reward Design
Providing detailed feedback to guide the learning process, using techniques like process rewards to validate steps.
3. Search Strategies
Methods like Monte Carlo Tree Search (MCTS) and beam search help in generating high-quality solutions.
4. Learning
This involves refining the model’s policies using data generated from searches.
By combining these elements, the framework enhances reasoning capabilities through proven methodologies.
Technical Details and Benefits
The roadmap tackles key technical challenges in reinforcement learning with innovative strategies:
- Policy Initialization: Large-scale pre-training builds strong language representations aligned with human reasoning.
- Reward Design: Incorporates process rewards to guide decision-making effectively.
- Search Methods: Balances exploration and exploitation using internal and external feedback.
These strategies reduce dependence on manually curated data, making the approach scalable and resource-efficient while enhancing reasoning capabilities.
Results and Insights
Implementing this roadmap has led to impressive results:
- Models trained with this framework show over 20% improvement in reasoning accuracy on challenging benchmarks.
- MCTS has proven effective in producing high-quality solutions.
- Iterative learning with search-generated data allows models to achieve advanced reasoning with fewer parameters.
These findings highlight the potential of reinforcement learning to replicate the performance of models like o1, offering insights for broader reasoning tasks.
Conclusion
The roadmap from Fudan University and Shanghai AI Laboratory presents a strategic approach to enhance AI reasoning abilities. By integrating policy initialization, reward design, search, and learning, it provides a comprehensive strategy for replicating o1’s capabilities. This framework addresses existing limitations and paves the way for scalable AI systems capable of tackling complex reasoning tasks.
Check out the Paper. All credit for this research goes to the researchers of this project. Also, don’t forget to follow us on Twitter and join our Telegram Channel and LinkedIn Group. Don’t Forget to join our 60k+ ML SubReddit.
Join this webinar to gain actionable insights into boosting LLM model performance and accuracy while safeguarding data privacy.
Transform Your Business with AI
To stay competitive and leverage AI effectively, consider the following steps:
- Identify Automation Opportunities: Find key customer interaction points that can benefit from AI.
- Define KPIs: Ensure measurable impacts on business outcomes.
- Select an AI Solution: Choose tools that fit your needs and allow customization.
- Implement Gradually: Start with a pilot, gather data, and expand AI usage wisely.
For AI KPI management advice, connect with us at hello@itinai.com. For continuous insights into leveraging AI, stay tuned on our Telegram t.me/itinainews or Twitter @itinaicom.
Discover how AI can redefine your sales processes and customer engagement. Explore solutions at itinai.com.