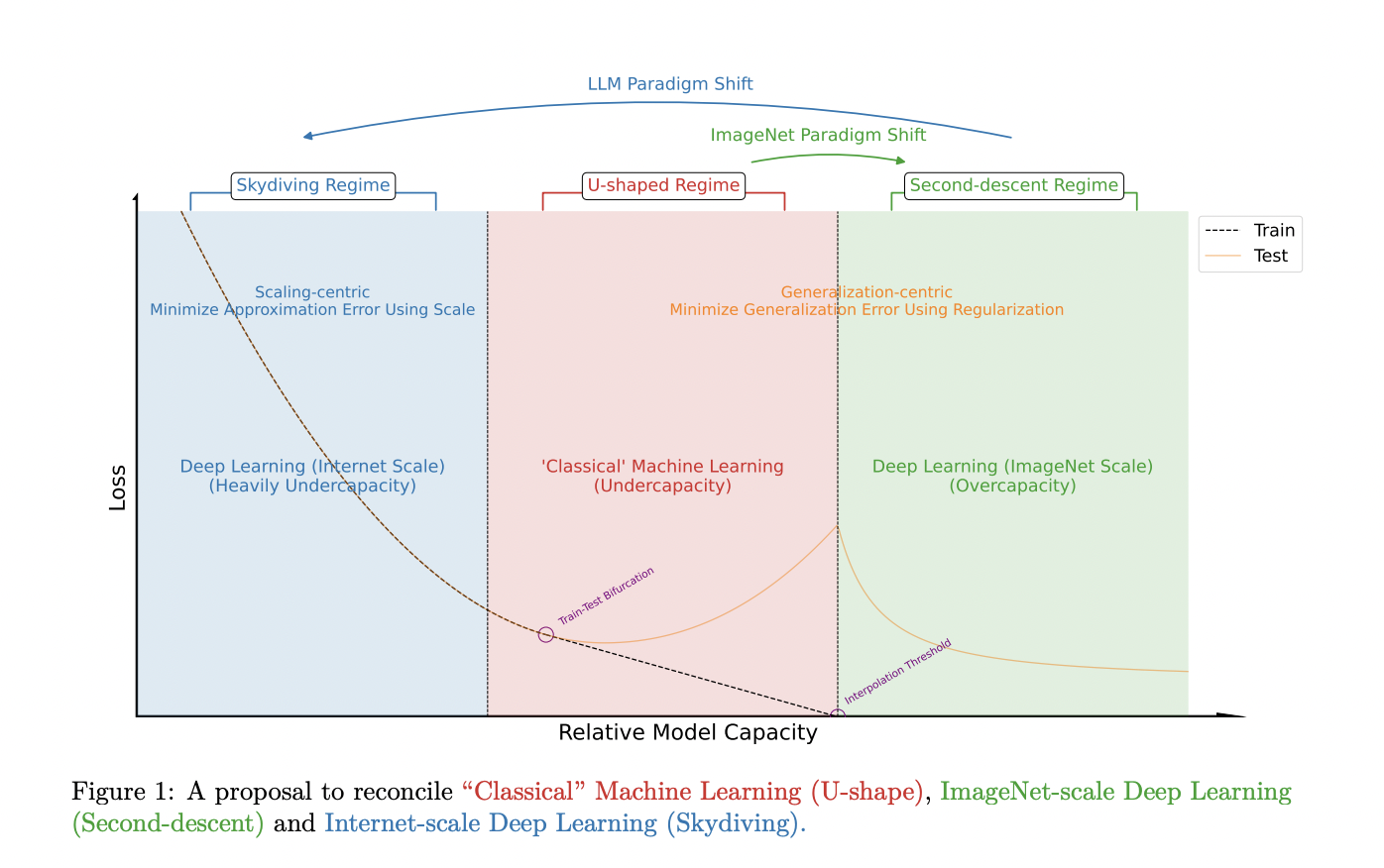
Practical Solutions and Value in AI
Paradigm Shift in Machine Learning
Researchers are now focusing on scaling up models to handle vast amounts of data, rather than just preventing overfitting. This shift requires new strategies to balance computational constraints with improved performance on tasks.
Distinct Machine Learning Paradigms
Two paradigms have emerged: generalization-centric and scaling-centric. Each requires different approaches to optimize model performance based on data and model scales.
Model Architecture and Training
A decoder-only transformer architecture trained on the C4 dataset is used, incorporating features like Rotary Positional Embedding and QK-Norm. The model aims to enhance performance in the scaling-centric paradigm.
Regularization Techniques Reevaluation
In the context of large language models, traditional regularization methods are being reevaluated for their effectiveness. Researchers are exploring new approaches suitable for the scaling-centric paradigm.
Challenges in Model Comparison
Comparing models at massive scales poses challenges, as traditional validation set approaches become impractical. Researchers need to find effective ways to compare models trained at scale.
AI Implementation Strategies
Implementing AI involves identifying automation opportunities, defining KPIs, selecting suitable AI solutions, and gradually expanding AI usage. Connect with us for AI KPI management advice and insights into leveraging AI.