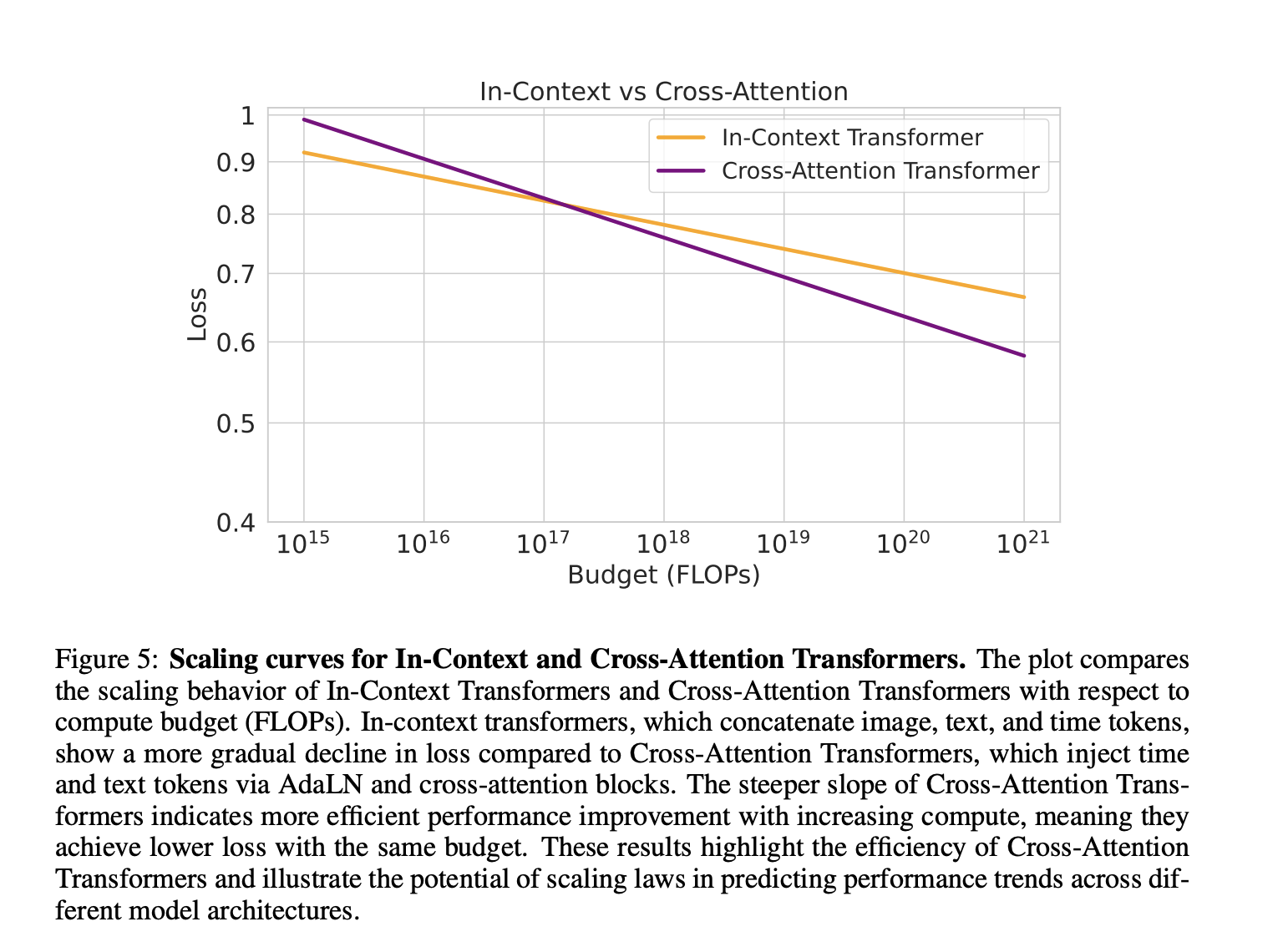
Understanding Scaling Laws in Diffusion Transformers
Large language models (LLMs) show a clear relationship between performance and the resources used during training. This helps optimize how we allocate our computing power. Unfortunately, diffusion models, especially diffusion transformers (DiT), lack similar guidelines. This makes it hard to predict outcomes and find the best sizes for models and data, leading researchers to use inefficient methods that can be costly.
Current Challenges
While scaling laws are well-studied in language models, diffusion models haven’t been analyzed in the same depth. Though larger diffusion models generally perform better, specific guidelines for optimizing resources and predicting performance are missing. This limitation impacts the progress researchers can make in this area.
New Research Breakthrough
Researchers from several prestigious institutions have now established scaling laws for diffusion models used in text-to-image synthesis. They examined a range of compute budgets and model sizes, finding optimal configurations that clearly relate compute resources to model size, data quantity, and loss during training.
Key Findings
The study revealed:
- Scaling laws exist between compute budgets and optimal configurations for diffusion models.
- Larger budgets generally lead to better performance in image generation.
- Metrics like Frechet Inception Distance (FID) align with these scaling laws, helping predict output quality.
Practical Applications
These findings not only help define the best model sizes and data requirements but also enable accurate predictions of performance across different datasets. By using these established scaling laws, organizations can:
- Optimize their resource allocation effectively.
- Improve their model designs by choosing the right architectures.
- Enhance the quality of their image generation processes.
Next Steps for Utilizing AI
For organizations looking to harness AI, consider the following:
- Identify Automation Opportunities: Find customer interaction points where AI can make an impact.
- Define KPIs: Set measurable goals for your AI projects.
- Select the Right AI Solution: Choose tools that match your specific needs.
- Implement Gradually: Start small, gather data, and expand wisely.
Stay Connected
For more insights and continuous updates on leveraging AI, connect with us on Telegram and follow us on @itinaicom. Explore how AI can transform your operations at itinai.com.
Upcoming Event
Don’t miss our live webinar on October 29, 2024, showcasing the best platform for serving fine-tuned models: Predibase Inference Engine.
For any inquiries or AI management advice, reach out at hello@itinai.com.