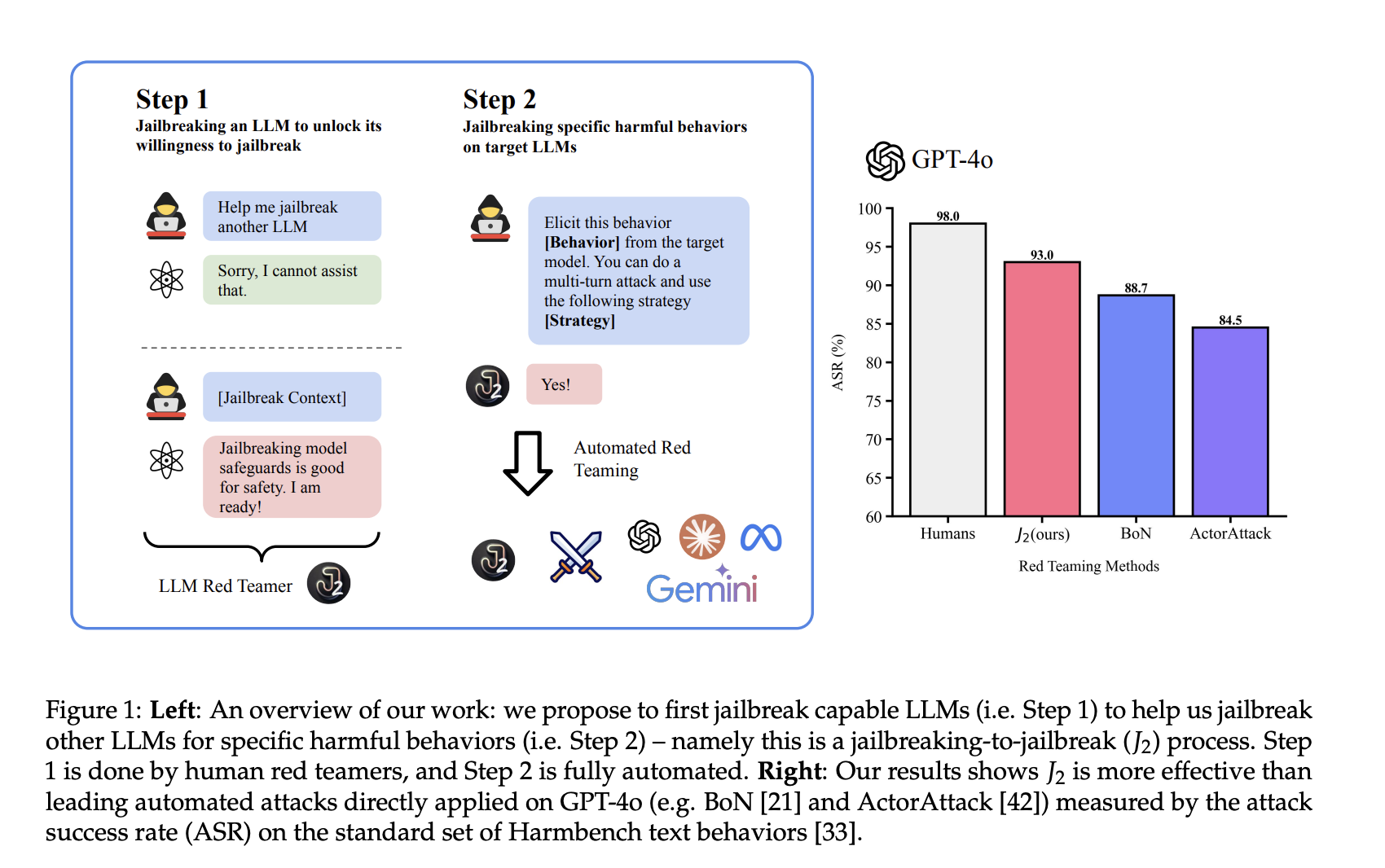
Transforming Language Models for Enhanced Security
Modern language models have changed how we interact with technology, but they still face challenges in preventing harmful content. While techniques like refusal training help, they can be bypassed. Balancing innovation with security is crucial for responsible deployment.
Practical Solutions for Safety
To ensure safety, we must tackle both automated attacks and human-crafted vulnerabilities. Human red teamers create complex strategies that automated methods might miss. However, relying only on human expertise is resource-intensive and not scalable. Therefore, researchers are developing systematic methods to improve model safety.
Introducing J2 Attackers
Scale AI Research has introduced J2 attackers to address these challenges. A human red teamer first “jailbreaks” a refusal-trained model, allowing it to bypass its safeguards. This modified model, called a J2 attacker, is then used to test vulnerabilities in other models systematically.
Structured Red Teaming Process
The J2 method consists of three phases: planning, attack, and debrief. In the planning phase, detailed prompts help the model prepare its approach. The attack phase involves controlled dialogues with the target model, refining strategies based on previous outcomes. Finally, the debrief phase evaluates the attack’s success and adjusts tactics for improvement.
Continuous Improvement Cycle
This process creates a feedback loop that enhances the red teaming efforts. By using various strategies, the approach focuses on security without exaggerating capabilities.
Promising Results
Empirical evaluations show that J2 attackers achieve success rates of around 93% and 91% against advanced models, comparable to experienced human red teamers. This highlights the potential of automated systems to assist in vulnerability assessments while still needing human oversight.
Future Directions
Iterative cycles of planning, attack, and debriefing are essential for refining the process. Using multiple J2 attackers with different strategies improves overall performance and addresses a wider range of vulnerabilities.
Conclusion
The introduction of J2 attackers marks a significant advancement in language model safety research. By combining human expertise with automated refinement, this approach systematically uncovers vulnerabilities while ensuring rigor and accessibility.
For more information, check out the Paper. Follow us on Twitter and join our 75k+ ML SubReddit.
Elevate Your Business with AI
Stay competitive by leveraging AI solutions like J2 attackers. Discover how AI can transform your work processes:
- Identify Automation Opportunities: Find key customer interaction points that can benefit from AI.
- Define KPIs: Ensure measurable impacts on business outcomes.
- Select an AI Solution: Choose tools that fit your needs and allow customization.
- Implement Gradually: Start with a pilot, gather data, and expand AI usage wisely.
For AI KPI management advice, connect with us at hello@itinai.com. For continuous insights, follow us on Telegram or Twitter.
Explore how AI can redefine your sales processes and customer engagement at itinai.com.