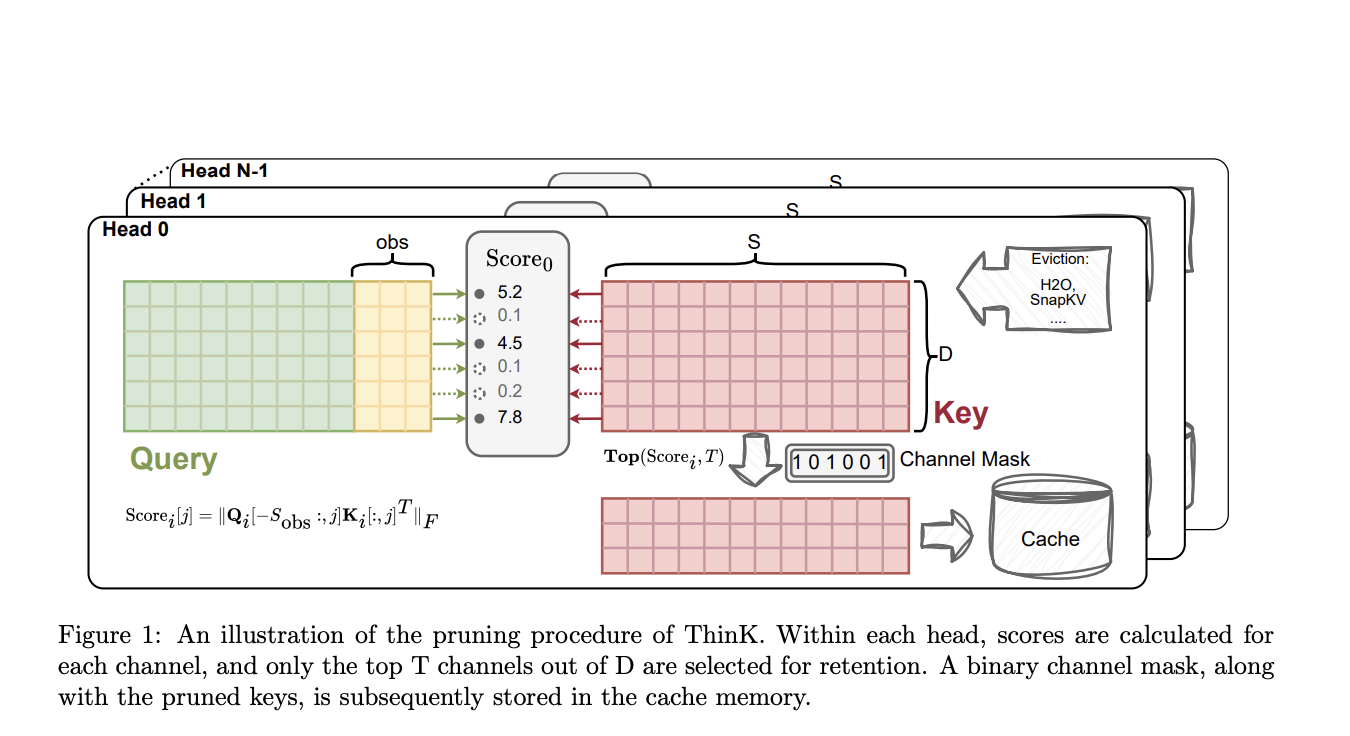
Practical Solutions and Value of ThinK: Optimizing Large Language Models
Revolutionizing Natural Language Processing
Large Language Models (LLMs) have transformed natural language processing, enhancing context understanding and enabling applications like document summarization, code generation, and conversational AI.
Challenges and Solutions
LLMs face cost and efficiency challenges due to increasing model size and sequence length. Researchers have developed ThinK, a unique method for optimizing the KV cache in LLMs by pruning the channel dimension of the key cache, reducing memory consumption while preserving model performance.
Key Features of ThinK
ThinK introduces a query-driven pruning criterion to evaluate channel importance, uses a greedy algorithm to select important channels, and focuses on long-context scenarios with an observation window to reduce computational costs.
Experimental Results
Experimental results demonstrate that ThinK successfully prunes key cache channels, outperforming existing compression methods and maintaining or improving performance across different benchmarks and pruning ratios.
Advancements and Future Implications
ThinK emerges as a promising advancement in optimizing Large Language Models for long-context scenarios, offering improved memory efficiency with minimal performance trade-offs. It paves the way for more efficient and powerful AI systems in the future, potentially revolutionizing long-context processing in language models.
Evolve Your Company with AI
If you want to evolve your company with AI, stay competitive, and use Salesforce AI Introduces ‘ThinK’ to redefine your way of work, connect with us for AI KPI management advice and continuous insights into leveraging AI.
Discover AI Solutions
Discover how AI can redefine your sales processes and customer engagement. Explore solutions at itinai.com.