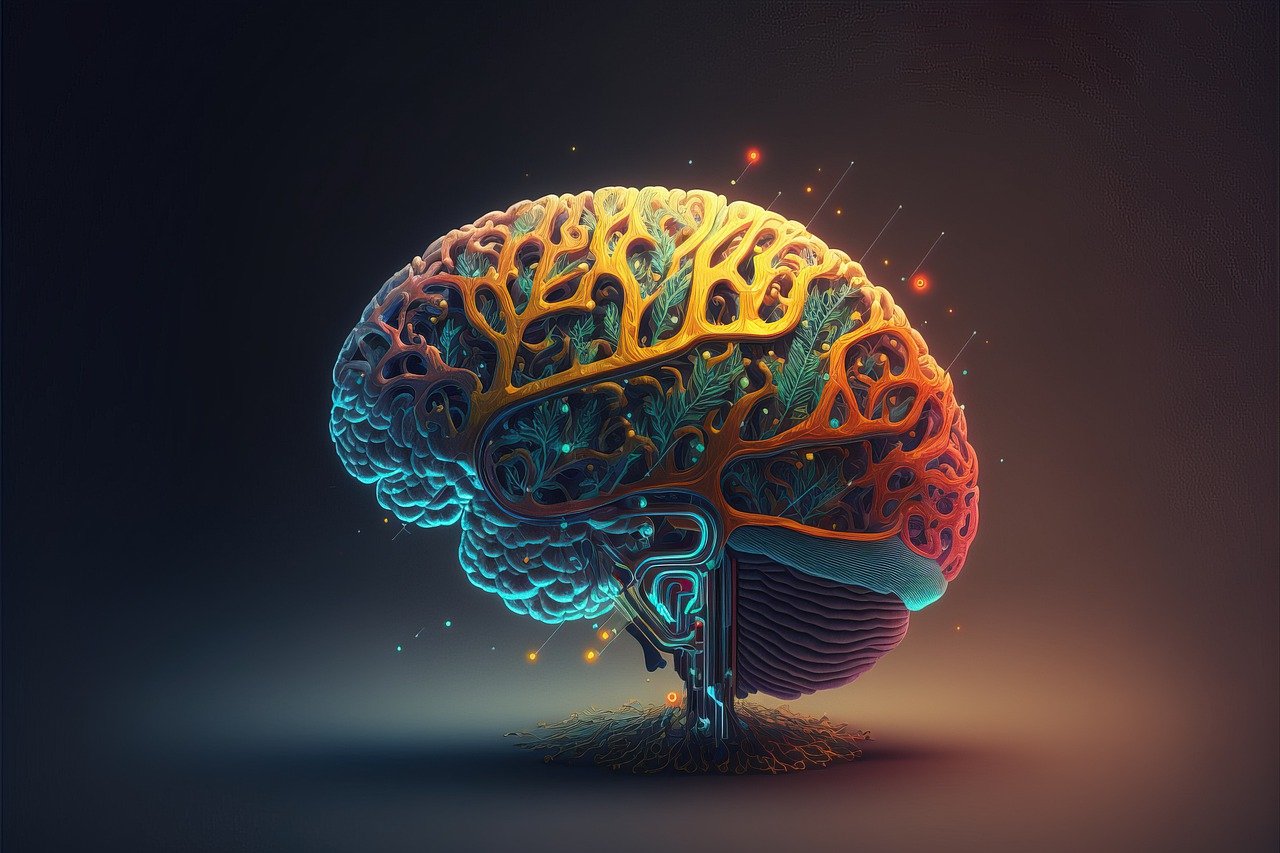
Understanding Retrieval-Augmented Generation (RAG)
Retrieval-augmented generation (RAG) combines information retrieval with generative AI to improve accuracy and relevance. This approach helps meet specific user needs effectively. Here’s a look at different RAG architectures and their practical applications.
Corrective RAG
Corrective RAG acts as a real-time fact-checker, ensuring responses are accurate by validating against trusted sources. For example, a healthcare chatbot can verify medication dosages with medical guidelines, making it vital in sectors like healthcare and finance.
Speculative RAG
Speculative RAG predicts user needs by analyzing behavior and preparing responses in advance. A news app might show trending articles on climate change based on a user’s search history, enhancing the experience in fast-paced environments like e-commerce and customer service.
Agenetic RAG
Agenetic RAG adapts over time, learning user preferences through interactions. A streaming service can prioritize thriller movie recommendations as it learns a user’s likes, making it effective in retail and entertainment.
Self-RAG
Self-RAG focuses on continuous improvement by evaluating its accuracy. A financial analysis tool can adjust predictions based on real-time data and user feedback, ensuring relevance in dynamic industries like finance and logistics.
Adaptive RAG
Adaptive RAG adjusts responses based on real-time user context. An airline booking system can suggest alternatives based on seat availability, making it suitable for ticketing and event management.
Refeed Feedback RAG
Refeed Feedback RAG learns from user corrections, refining its responses. A telecom chatbot can improve accuracy over time by incorporating user feedback, ideal for customer service.
Realm RAG
Realm RAG combines retrieval with deep contextual understanding, delivering precise answers in technical fields. A legal assistant can find relevant case precedents, saving time in research.
Raptor RAG
Raptor RAG organizes data hierarchically, allowing quick access to relevant information. A hospital can categorize patient symptoms efficiently, crucial for healthcare and e-commerce.
Replug RAG
Replug RAG connects with external data sources for real-time insights. A financial platform can fetch the latest market trends, enhancing relevance in data-intensive industries.
Memo RAG
Memo RAG retains context across interactions, improving continuity. A virtual assistant can recall previous customer issues, enhancing user satisfaction in support and education.
Attention-Based RAG
Attention-Based RAG filters out irrelevant details to provide focused responses. A research assistant can identify key studies on “AI in healthcare,” making it valuable in academia and legal research.
RETRO RAG
RETRO RAG uses historical context for informed responses. A corporate knowledge system can recall past project decisions, essential for continuity in organizations.
Auto RAG
Auto RAG automates data retrieval with minimal human oversight. A news aggregator can compile headlines automatically, ideal for real-time content platforms.
Cost-Constrained RAG
Cost-Constrained RAG optimizes data retrieval within budget limits, balancing efficiency and cost. Non-profits can access essential data while minimizing expenses.
ECO RAG
ECO RAG minimizes energy consumption during data retrieval, supporting sustainable practices. An environmental monitoring platform can optimize energy use effectively.
Rule-Based RAG
Rule-Based RAG ensures compliance with regulations, vital in regulated industries. A financial advisory platform can provide compliant investment recommendations.
Conversational RAG
Conversational RAG enables natural dialogue, enhancing user engagement. A retail chatbot can adapt responses based on customer queries, improving satisfaction in e-commerce.
Iterative RAG
Iterative RAG refines responses through multiple interactions. A tech support bot can offer more specific solutions based on past feedback, ideal for troubleshooting.
HybridAI RAG
HybridAI RAG combines multiple models for comprehensive analysis. Predictive maintenance platforms can analyze various data sources to anticipate equipment failures.
Generative AI RAG
Generative AI RAG merges retrieval with creative content generation. A marketing assistant can create new ad copy by analyzing past trends, supporting innovative campaigns.
XAI RAG
XAI RAG emphasizes explainability, ensuring users understand responses. In healthcare, it can recommend treatments with clear reasoning, fostering trust in regulated industries.
Context Cache RAG
Context Cache RAG maintains relevant data across interactions, enhancing continuity. A virtual tutor can recall previous lessons for tailored guidance, improving educational outcomes.
Grokking RAG
Grokking RAG synthesizes complex data for intuitive explanations. A research assistant can simplify intricate concepts, making them accessible to broader audiences.
Replug Retrieval Feedback RAG
Replug Retrieval Feedback RAG enhances connections to data sources through feedback, ensuring precision. A market insights platform can adjust its algorithms for accurate data retrieval.
Conclusion
These 25 RAG architectures showcase the potential of AI to transform various industries. By selecting the right RAG type, organizations can optimize processes, enhance user experiences, and drive innovation. Explore how AI can redefine your operations and identify opportunities for automation to stay competitive.
For more insights and updates, follow us on social media and join our community.