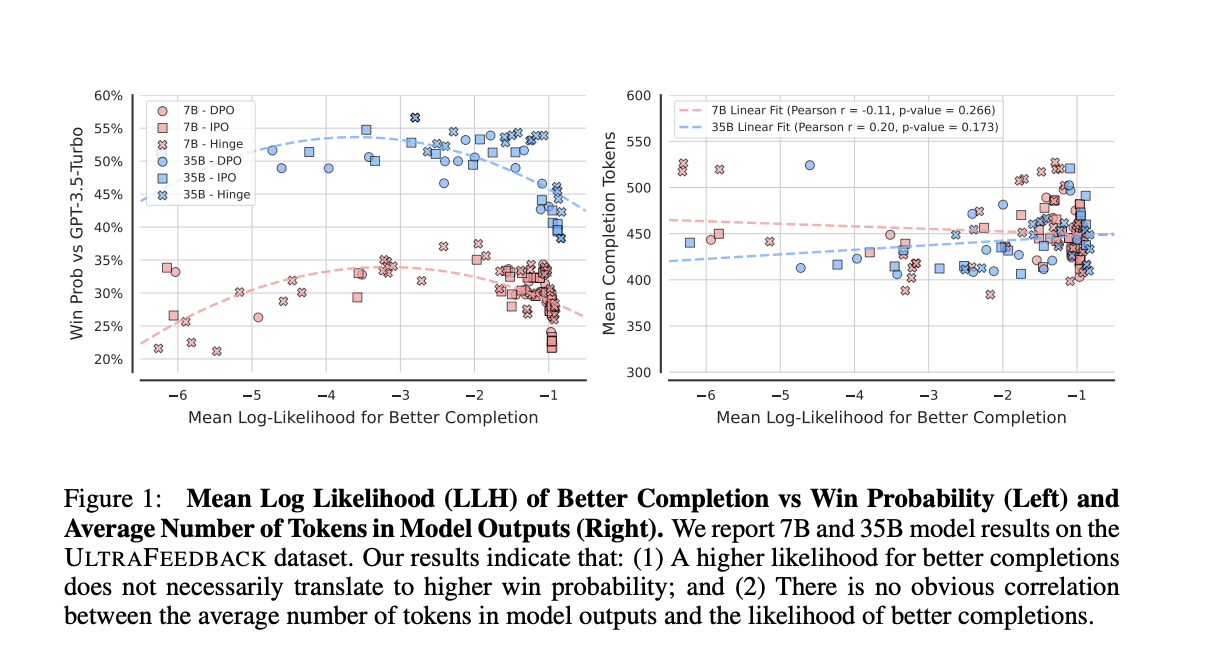
Understanding the Challenges of Direct Alignment Algorithms
The issue of over-optimization in Direct Alignment Algorithms (DAAs) like Direct Preference Optimization (DPO) and Identity Preference Optimization (IPO) is significant. These methods aim to align language models with human preferences but often fail to enhance model performance despite increasing the likelihood of preferred outcomes. This indicates a flaw in relying solely on likelihood as the alignment goal.
Research Insights from University College London and Cohere
Researchers investigated whether boosting the likelihood of preferred completions improves performance. They found that higher likelihood does not always lead to better alignment with human preferences. In fact, slightly lowering the likelihood can enhance output diversity, which helps models generalize better to new data. Two key indicators of over-optimization were identified: decreasing entropy over Top-k Tokens and diminishing Top-k Probability Mass.
Research Methodology
The study involved analyzing the relationship between completion likelihood and performance metrics across various DAAs. Researchers used two instruction-tuned models (7B and 35B parameters) trained on the ULTRAFEEDBACK dataset. They experimented with different hyperparameters for DPO, IPO, and a Hinge loss function while monitoring the log-likelihood of preferred completions. Regularization techniques like Negative Log-Likelihood (NLL) were also applied to prevent over-optimization.
Key Findings
Results showed that higher likelihoods of preferred completions do not guarantee improved performance compared to models like GPT-3.5 Turbo. Both models exhibited weak correlations between completion likelihood and win probability. Interestingly, models with slightly reduced likelihoods demonstrated greater output diversity, positively impacting generalization, especially in early training stages. However, excessive diversity could lead to overly random outputs that harm performance.
Conclusion and Recommendations
The research highlights the need for a balance between increasing the likelihood of preferred completions and promoting diversity for better model performance. Monitoring entropy and probability mass can serve as early indicators of over-optimization. Adaptive regularization techniques are recommended during training to maintain this balance.
Practical AI Solutions for Businesses
To leverage AI effectively, consider the following strategies:
- Identify Automation Opportunities: Find key customer interaction points that can benefit from AI.
- Define KPIs: Ensure your AI initiatives have measurable impacts on business outcomes.
- Select an AI Solution: Choose tools that align with your needs and offer customization.
- Implement Gradually: Start with a pilot project, gather data, and expand AI usage wisely.
For AI KPI management advice, connect with us at hello@itinai.com. For ongoing insights into leveraging AI, follow us on Telegram or @itinaicom.
Discover how AI can transform your sales processes and customer engagement. Explore solutions at itinai.com.
Check out the research paper for more details. Follow us on Twitter, join our Telegram Channel, and LinkedIn Group for updates. If you enjoy our work, subscribe to our newsletter and join our 50k+ ML SubReddit community.
Upcoming Live Webinar – Oct 29, 2024
Join us for a session on the best platform for serving fine-tuned models: Predibase Inference Engine.