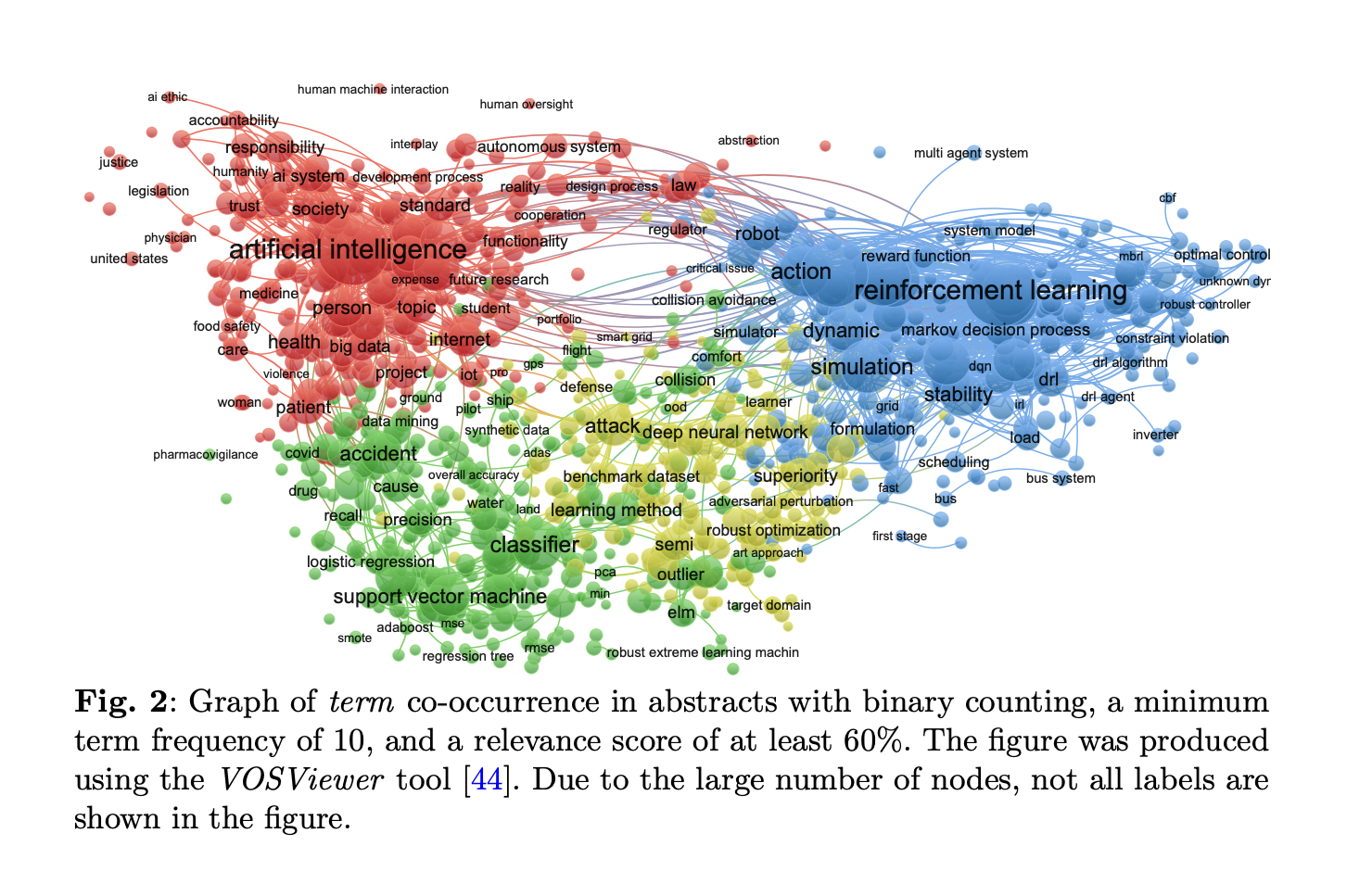
Rethinking AI Safety: Balancing Existential Risks and Practical Challenges
Understanding AI Safety
Recent discussions about AI safety often focus on the extreme risks posed by advanced AI. This narrow view can overlook valuable research and mislead the public into thinking AI safety is only about catastrophic threats. To address this, policymakers need to create regulations and safety standards for AI. Learning from past technologies like aviation and cybersecurity can help us develop effective safety practices for AI deployment.
Key Findings from Research
Researchers from the University of Edinburgh and Carnegie Mellon University emphasize that AI safety discussions should include a wider range of perspectives. Their review of existing research shows various safety concerns, such as:
- Adversarial robustness
- Interpretability
They recommend combining short-term and long-term risk assessments instead of only focusing on existential threats. This broader approach can help tackle both immediate and future AI risks effectively.
Research Methodology
The researchers reviewed AI safety literature using a structured method, analyzing 2,666 papers from databases like Web of Science and Scopus. They focused on identifying risks throughout the AI system lifecycle and evaluating strategies to mitigate these risks. Ultimately, they selected 383 papers for detailed analysis.
Trends in AI Safety Research
Since 2016, AI safety research has grown steadily, driven by deep learning advancements. Key themes identified include:
- Safe reinforcement learning
- Adversarial robustness
- Domain adaptation
This research aligns with traditional safety engineering principles, ensuring AI systems are both effective and secure.
Types of Risks in AI Safety
AI safety research identifies eight types of risks, including:
- Noise
- Lack of monitoring
- System misspecification
- Adversarial attacks
Most studies focus on noise and monitoring failures, which impact model reliability. Recent research emphasizes safety in reinforcement learning and adversarial robustness, paralleling traditional engineering safety practices.
Conclusion and Future Directions
The study highlights the importance of diverse motivations in AI safety research. It addresses risks like design flaws and inadequate monitoring, advocating for a broader view of AI safety. Future research should explore sociotechnical aspects and include non-peer-reviewed sources for a more comprehensive understanding.
Explore AI Solutions for Your Business
If you want to enhance your company with AI, consider the following steps:
- Identify Automation Opportunities: Find areas in customer interactions that can benefit from AI.
- Define KPIs: Ensure your AI initiatives have measurable impacts.
- Select an AI Solution: Choose tools that meet your needs and allow for customization.
- Implement Gradually: Start with a pilot project, gather data, and expand usage wisely.
Get in Touch
For AI KPI management advice, contact us at hello@itinai.com. For ongoing insights into leveraging AI, follow us on Telegram or Twitter @itinaicom.
Discover how AI can transform your sales processes and customer engagement. Visit itinai.com for more information.