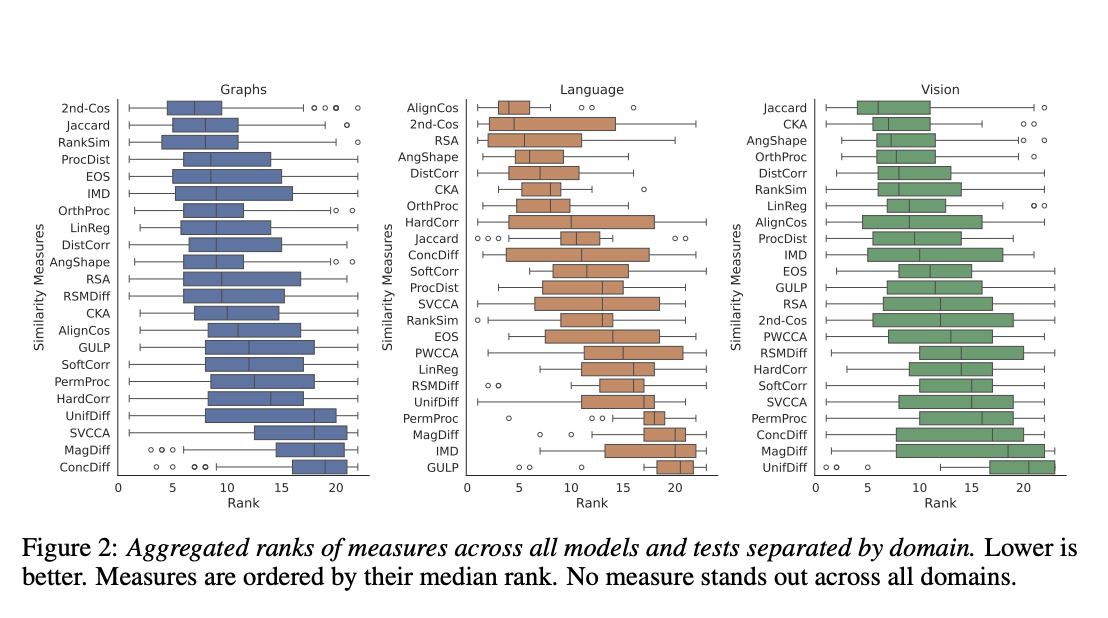
Practical AI Solutions for Evaluating Representational Similarity
Overview
Representational similarity measures play a crucial role in machine learning, aiding in the comparison of internal neural network representations. They offer insights into learning dynamics, model behaviors, and performance across various tasks and architectures. Quantifying representation similarity is vital for model evaluation, transfer learning, and understanding the impact of different training methods.
Challenges and Solutions
Challenges in this field include the lack of comprehensive benchmarks for evaluating similarity measures. To address this, the Representational Similarity (ReSi) benchmark was introduced. This framework provides a systematic and robust platform for evaluating similarity measures, covering a wide range of tests, architectures, and datasets. The benchmark aims to help researchers make informed decisions when selecting similarity measures for specific applications.
Key Findings
Evaluation of the ReSi benchmark revealed that no single similarity measure consistently outperformed others across all domains. It highlighted the strengths and weaknesses of different measures, guiding researchers in choosing the most appropriate measure for their specific needs. Detailed results from the benchmark demonstrated the complexity and variability in evaluating representational similarity, emphasizing the importance of using a comprehensive benchmark like ReSi.
Advantages of ReSi Benchmark
The ReSi benchmark significantly advances machine learning by providing a systematic and robust platform for evaluating representational similarity measures. It fills a critical gap in evaluating representational similarity measures and facilitates more rigorous and consistent evaluations, catalyzing future research by providing a solid foundation for developing and testing new similarity measures.
AI Solutions for Business Transformation
To evolve your company with AI and stay competitive, the ReSi benchmark can be used to identify automation opportunities, define KPIs for AI endeavors, select suitable AI solutions, and implement AI gradually. It enables companies to redefine their way of work and leverage AI for sales processes and customer engagement, providing guidance on leveraging AI for business outcomes.
Conclusion
The ReSi benchmark offers a comprehensive, systematic framework that enhances the understanding of how different similarity measures perform across various neural network architectures and tasks. It promotes more rigorous and consistent evaluations, driving AI-driven business transformation and providing insights into leveraging AI for sales processes and customer engagement.