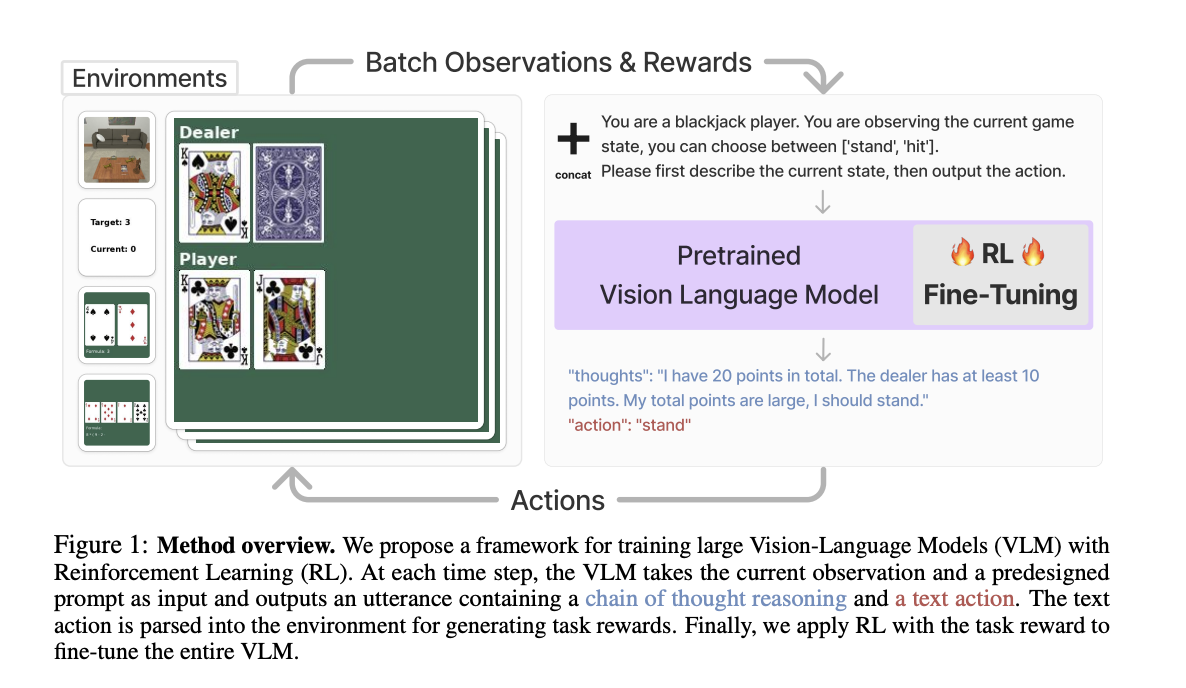
Practical Solutions for Vision-Language Models (VLMs)
Enhancing VLM Performance
Large Vision-Language Models (VLMs) can be fine-tuned with specific visual instruction-following data to greatly enhance their performance in solving a wide range of tasks.
Overcoming Drawbacks with Reinforcement Learning
Reinforcement Learning (RL) offers a way to fully develop the decision-making capabilities of VLM agents in multi-step interactive environments, addressing the limitations of supervised learning from pre-gathered information.
Algorithmic Framework for Optimization
A team of researchers has developed an algorithmic framework that uses Reinforcement Learning to optimize VLMs, enabling them to excel in decision-making tasks by incorporating Chain-Of-Thought (CoT) reasoning.
Performance Enhancements
The empirical findings demonstrate that this paradigm significantly enhances VLM agents’ performance in decision-making tasks, outperforming popular commercial models and showcasing the significance of CoT reasoning in the RL training framework.
Evolve Your Company with AI
Researchers from UC Berkeley, UIUC, and NYU have developed an Algorithmic Framework that Uses Reinforcement Learning (RL) to Optimize Vision-Language Models (VLMs), offering practical solutions for companies looking to leverage AI for competitive advantage.
AI Redefining Work Processes
Discover how AI can redefine your way of work by identifying automation opportunities, defining KPIs, selecting AI solutions, and implementing AI gradually to drive measurable impacts on business outcomes.
Practical AI Solutions
Consider the AI Sales Bot from itinai.com/aisalesbot designed to automate customer engagement 24/7 and manage interactions across all customer journey stages.