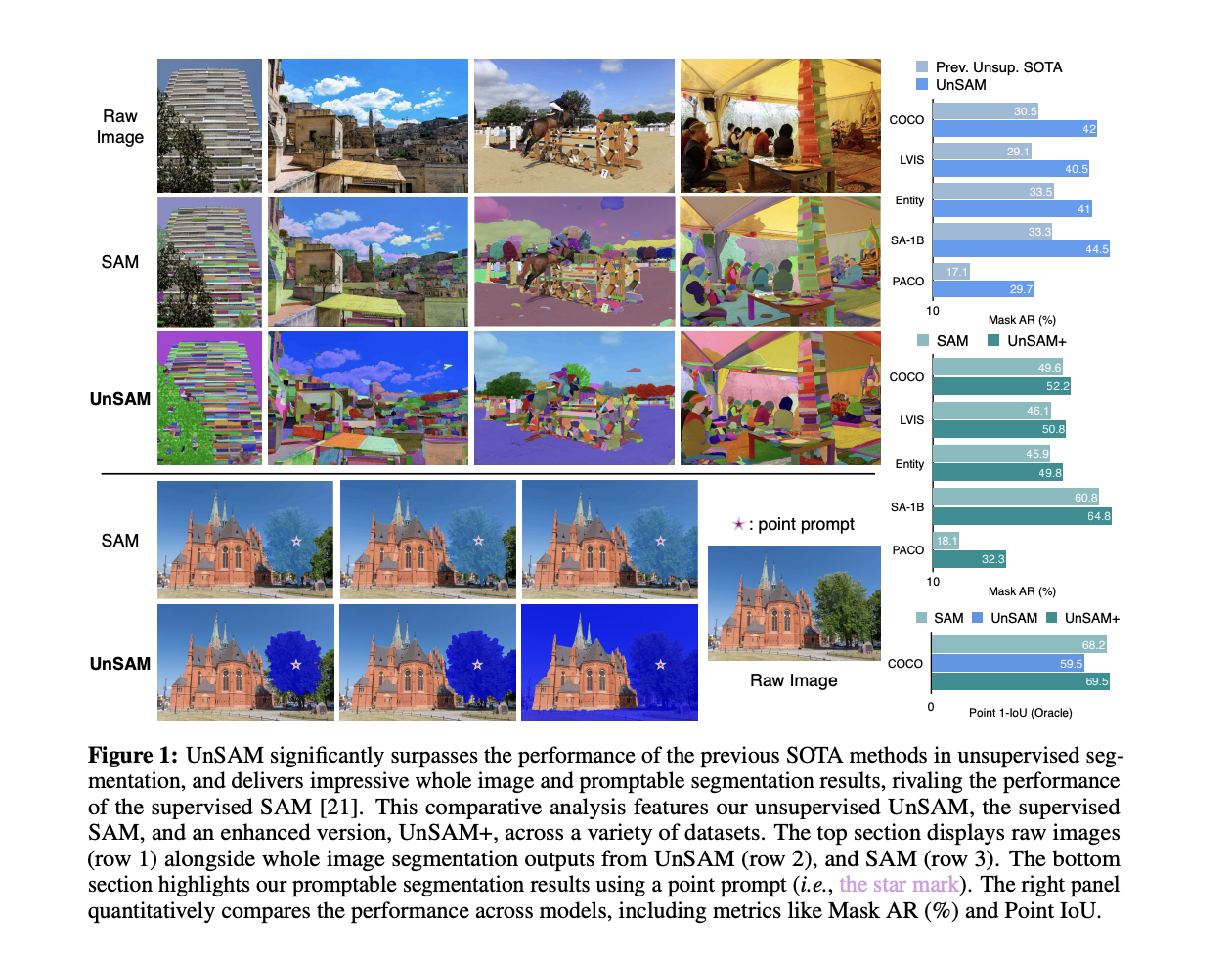
Practical Solutions and Value of Unsupervised SAM in Computer Vision
Introduction
Unsupervised SAM (UnSAM) offers a groundbreaking approach to segmentation tasks in Computer Vision, providing high-quality results without the need for extensive manual labeling. It outperforms traditional methods like SAM, offering significant advancements in accuracy and efficiency.
Key Features and Innovations
UnSAM utilizes a divide-and-conquer strategy to create segmentation masks with varying levels of granularity, capturing intricate details in visual scenes. Its Top-Down and Bottom-Up clustering strategies ensure parallel performance with human-labeled datasets like SA-1B.
Performance and Achievements
UnSAM surpasses SAM by significant margins in metrics like Average Recall (AR) and Average Precision (AP) on datasets such as SA-1B, PartImageNet, and PACO. Even with minimal training data, UnSAM achieves state-of-the-art results, showcasing its superiority in unsupervised vision learning.
Conclusion
UnSAM demonstrates that exceptional segmentation outcomes can be achieved without large annotated datasets, making it a valuable tool for fields like medicine and science. Its efficiency and accuracy highlight a new era in unsupervised vision learning, offering immense potential for AI applications.