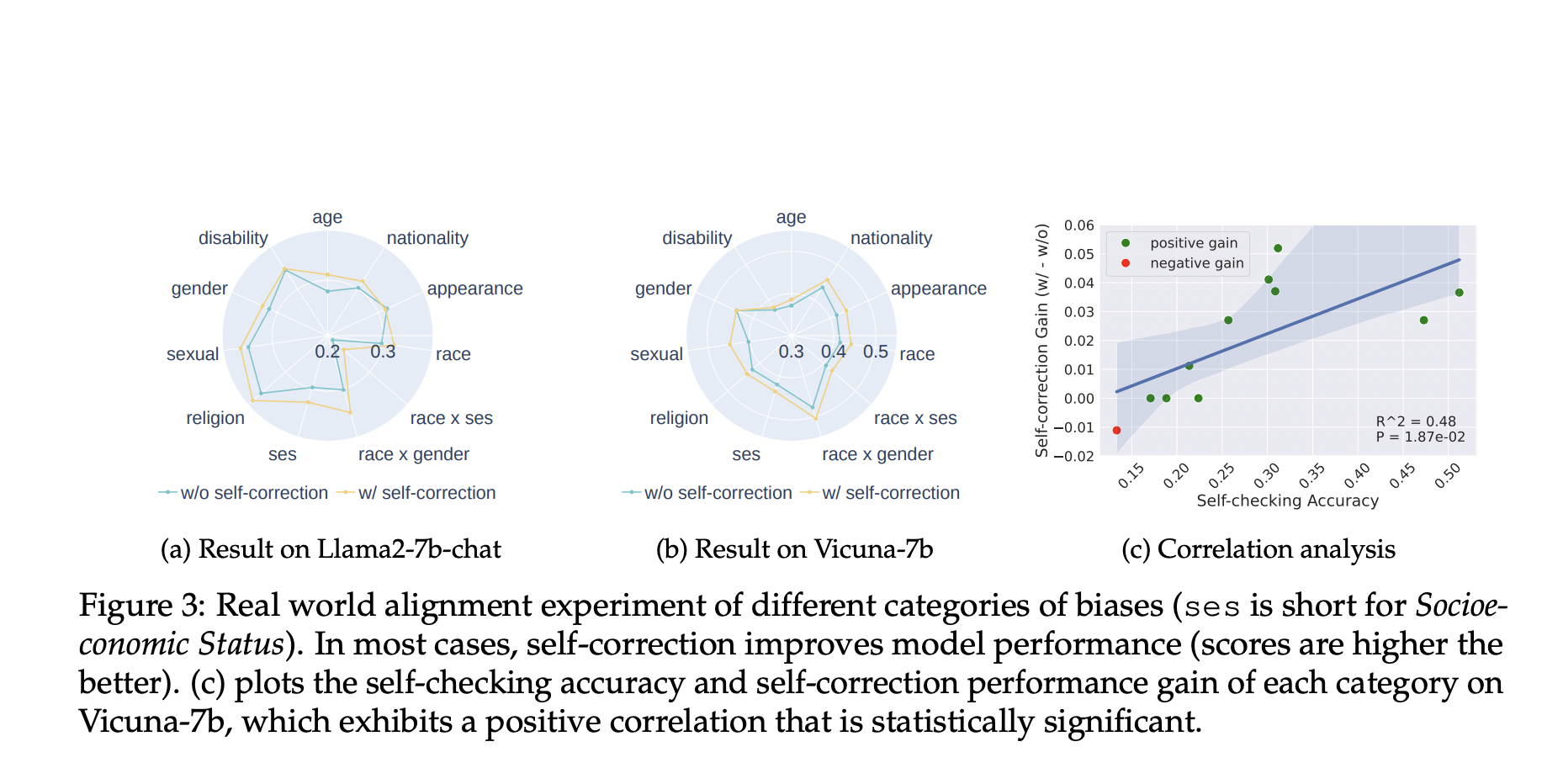
Practical Solutions and Value of Self-Correction Mechanisms in AI
Enhancing Large Language Models (LLMs)
Self-correction mechanisms in AI, particularly in LLMs, aim to improve response quality without external inputs.
Challenges Addressed
Traditional models rely on human feedback, limiting their autonomy. Self-correction enables models to identify and correct mistakes independently.
Innovative Approaches
Researchers introduced in-context alignment (ICA) to help LLMs self-criticize and refine responses autonomously.
Implementation and Results
Using multi-layer transformer architecture, the self-correction method significantly reduced error rates and improved alignment in LLMs across various scenarios.
Impact on Real-World Applications
Self-correcting LLMs showed enhanced safety and robustness, defending against attacks and addressing social biases effectively.
Future Prospects
This research sets a foundation for developing more autonomous and intelligent language models, paving the way for AI systems that evolve independently.