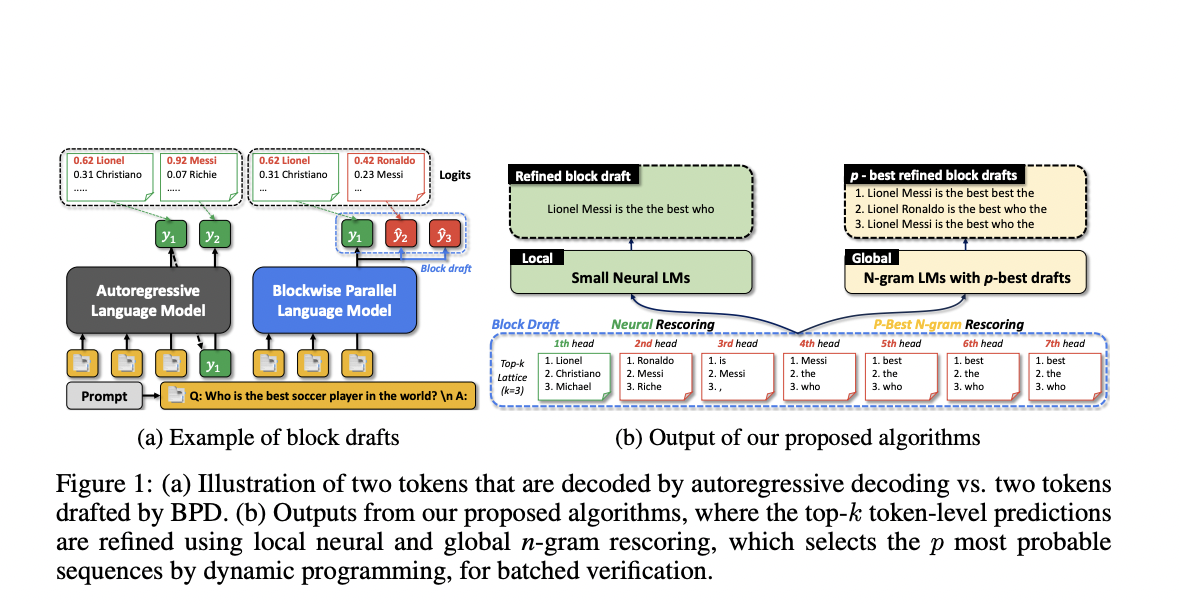
Practical Solutions and Value of Blockwise Parallel Decoding (BCD) in AI Language Models
Overview
Recent advancements in autoregressive language models like GPT have revolutionized Natural Language Processing (NLP) by excelling in text creation tasks. However, their slow inference speed hinders real-time deployment.
Blockwise Parallel Decoding (BCD)
BCD accelerates inference by predicting multiple tokens simultaneously, reducing latency and computing demand. It enhances model efficiency by optimizing token predictions for fluency and accuracy.
Improvements
The team enhanced block drafts by analyzing token distributions and implementing algorithms using neural language models and n-gram models. This led to a 5-21% increase in block efficiency across various datasets.
Key Contributions
- Studied prediction heads in BCD models, identifying issues like falling confidence in predictions and token repetition.
- Introduced Oracle top-k block efficiency concept to improve block efficiency by reducing repetition and uncertainty.
- Implemented Global and Local rescoring algorithms to refine block drafts, increasing efficiency by up to 21.3%.
AI Implementation Tips
- Identify Automation Opportunities
- Define KPIs for measurable impacts
- Select AI Solutions aligned with needs
- Implement Gradually starting with a pilot
Connect with Us
For AI KPI management advice, contact us at hello@itinai.com. Stay updated on AI insights via Telegram @itinainews or Twitter @itinaicom.
Discover how AI can transform your sales processes and customer engagement at itinai.com.