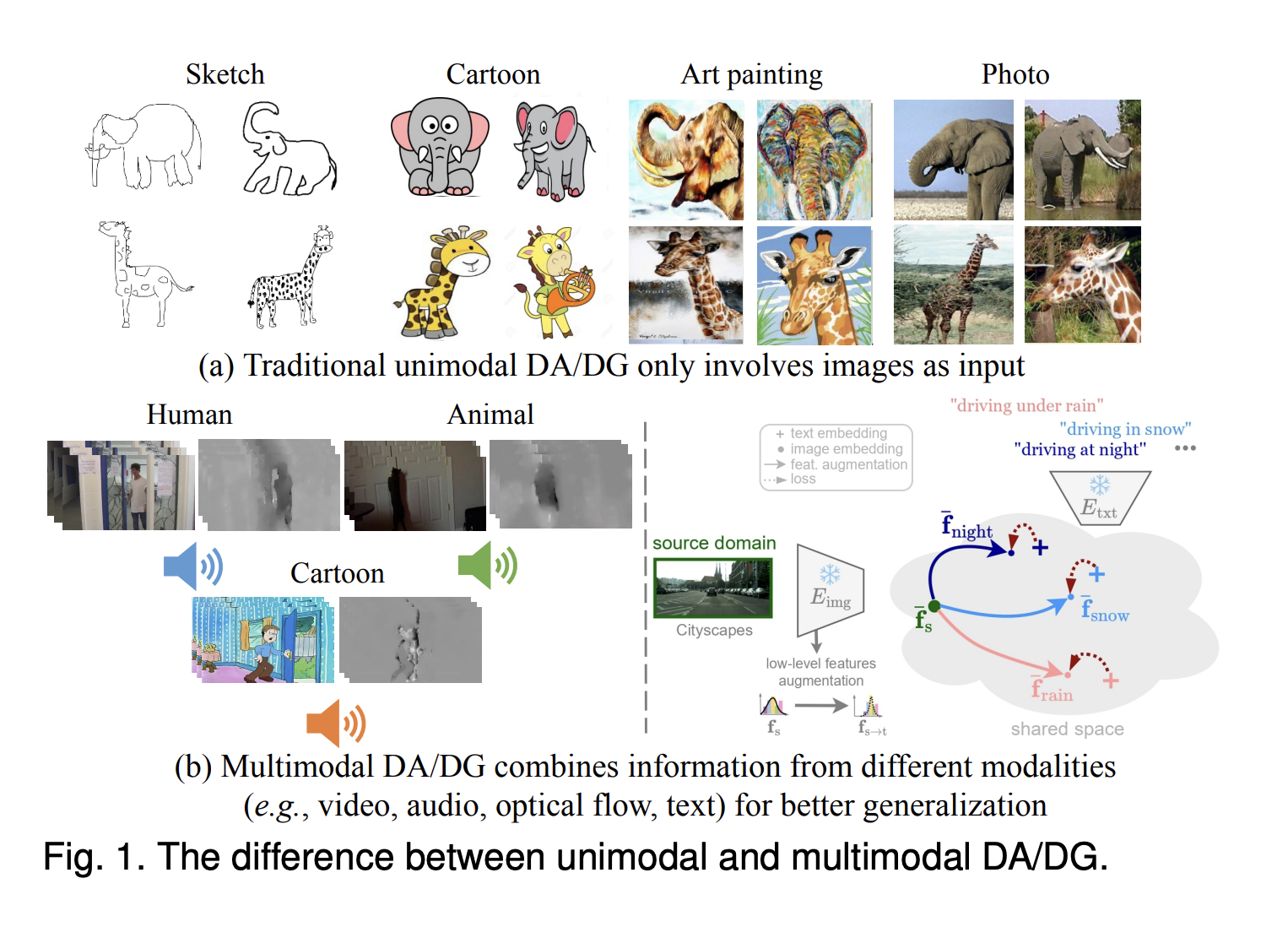
Understanding Multimodal AI Adaptation and Generalization
Artificial intelligence (AI) has made significant progress in many areas. However, to truly assess its development, we must look at how well AI models can adapt and generalize across different fields. This is where Domain Adaptation (DA) and Domain Generalization (DG) come into play, attracting attention from researchers worldwide.
Key Challenges and Solutions
Training AI models is resource-intensive, and there is a shortage of high-quality data. Therefore, models trained on limited data must perform well in new situations. Research in DA and DG has largely focused on single data types, like images or time series. With the rise of large multimodal datasets, researchers are now tackling Multimodal Domain Adaptation (MMDA) and Multimodal Domain Generalization (MMDG), which present unique challenges due to the differences in data types.
Recent Advances in MMDA and MMDG
Researchers from ETH Zurich and TUM, Germany, have conducted an extensive survey on advancements in Multimodal Adaptation and Generalization. Their work covers:
- Multimodal Domain Adaptation: This aims to enhance knowledge transfer between different domains. The challenge lies in the varying characteristics of data types and missing inputs. Solutions include adversarial learning and cross-modal interaction techniques, with frameworks like MM-SADA and xMUDA leading the way.
- Multimodal Test-Time Adaptation: Unlike MMDA, this focuses on models adjusting during use without needing labeled data. Key challenges include limited source data and continuous data shifts. Techniques like self-supervised learning and uncertainty estimation have been developed, with contributions like READ and Adaptive Entropy Optimization.
- Multimodal Domain Generalization: This seeks to train models that can handle entirely new domains without prior data. Challenges include the lack of target domain data and inconsistent feature distributions. Research has focused on Feature Disentanglement and Cross-Modal Knowledge Transfer, with algorithms like SimMMDG and MOOSA.
- Using Multimodal Foundation Models: Foundation models like CLIP have shown promise in improving DA and DG. However, they require significant computational resources. Researchers are exploring methods like feature-space augmentation and synthetic data generation to address these challenges.
- Fine-Tuning Multimodal Foundation Models: This involves adapting foundation models for specific tasks. Techniques like Prompt-Based Learning and Adapter-Based Tuning have been proposed to manage computational costs and data limitations, with notable works including CoOp and CLIP-Adapter.
Conclusion
This article highlights the importance of generalizability and adaptability in multimodal AI applications. It reviews various research areas and approaches, from basic methods to advanced foundation models. The survey provides valuable insights and outlines future directions for developing more effective AI frameworks.
For more information, check out the Paper and GitHub Page. Follow us on Twitter, join our Telegram Channel, and connect with our LinkedIn Group. Don’t forget to join our 75k+ ML SubReddit.
Transform Your Business with AI
To stay competitive and leverage AI effectively, consider the following steps:
- Identify Automation Opportunities: Find key areas in customer interactions that can benefit from AI.
- Define KPIs: Ensure your AI initiatives have measurable impacts on business outcomes.
- Select an AI Solution: Choose tools that fit your needs and allow for customization.
- Implement Gradually: Start with a pilot project, gather data, and expand AI usage carefully.
For AI KPI management advice, contact us at hello@itinai.com. For ongoing insights into AI, follow us on Telegram or @itinaicom.
Discover how AI can enhance your sales processes and customer engagement at itinai.com.