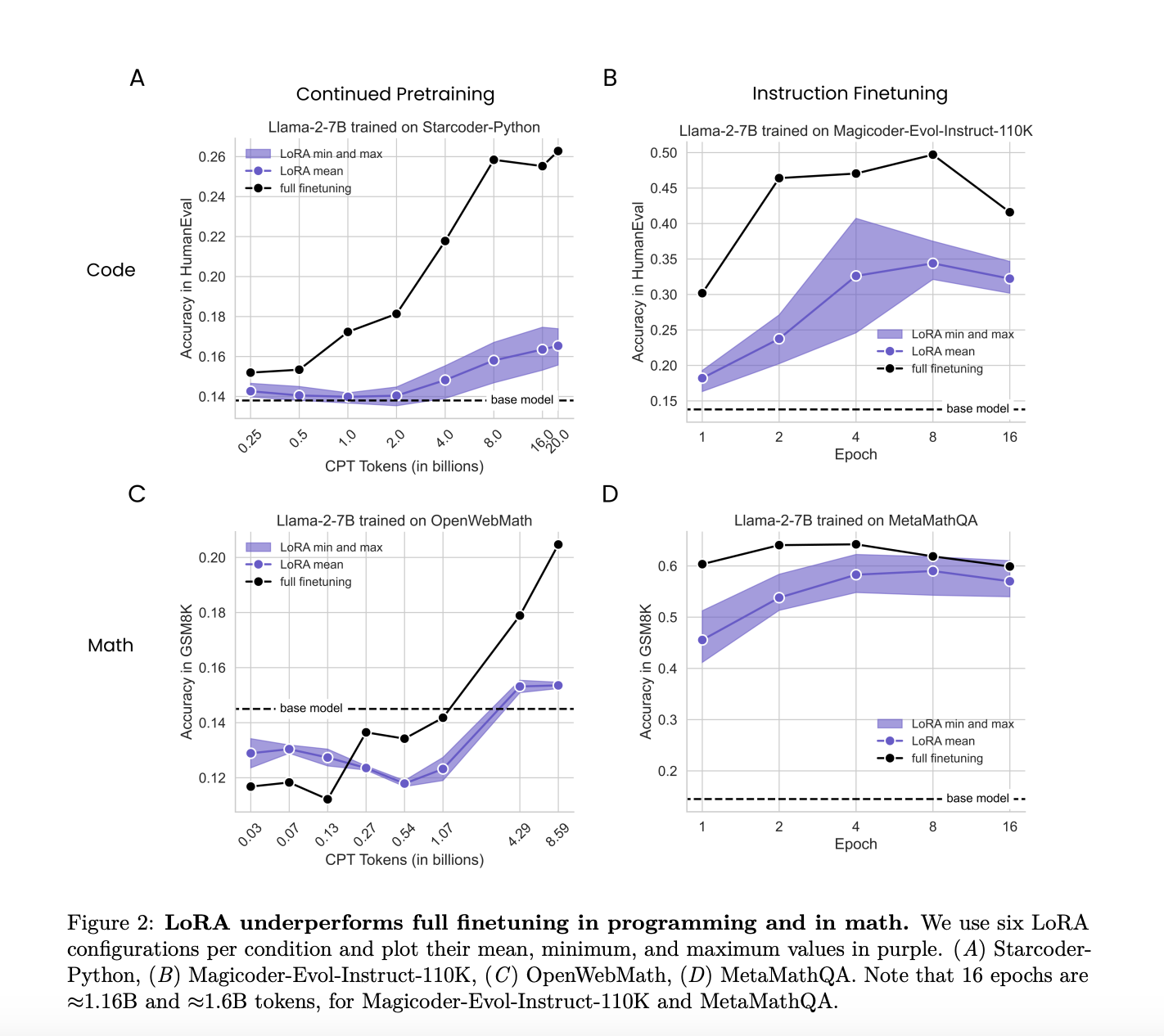
Practical AI Solutions for Large Language Models
Machine learning models with billions of parameters need efficient methods for performance tuning. Enhancing accuracy while minimizing computational resources is crucial for practical applications in natural language processing and artificial intelligence. Efficient resource utilization significantly impacts overall performance and feasibility.
Innovative Approaches
Researchers have explored methods to address the challenge of fine-tuning Large Language Models (LLMs). This includes techniques like Low-Rank Adaptation (LoRA) to reduce computational load and memory usage while maintaining model performance. The study compared the performance of LoRA and full finetuning across programming and mathematics tasks, providing valuable insights into their strengths and weaknesses under different conditions.
Implications and Benefits
The research revealed that while full finetuning generally outperformed LoRA in accuracy and efficiency, LoRA offers advantages in regularization and memory efficiency. It also maintains the base model’s capabilities and generates diverse outputs, making it valuable in certain contexts. This study provides essential insights into balancing performance and computational efficiency in finetuning LLMs, offering a pathway for more sustainable and versatile AI development.
AI for Business Evolution
To evolve your company with AI, it is essential to understand automation opportunities, define KPIs, select appropriate AI solutions, and implement them gradually. Consider practical AI solutions like the AI Sales Bot designed to automate customer engagement 24/7 and manage interactions across all customer journey stages.
Get In Touch
For AI KPI management advice and continuous insights into leveraging AI, connect with us at hello@itinai.com or follow us on Telegram and Twitter.
Check out our practical AI solutions at itinai.com/aisalesbot to redefine your sales processes and customer engagement.