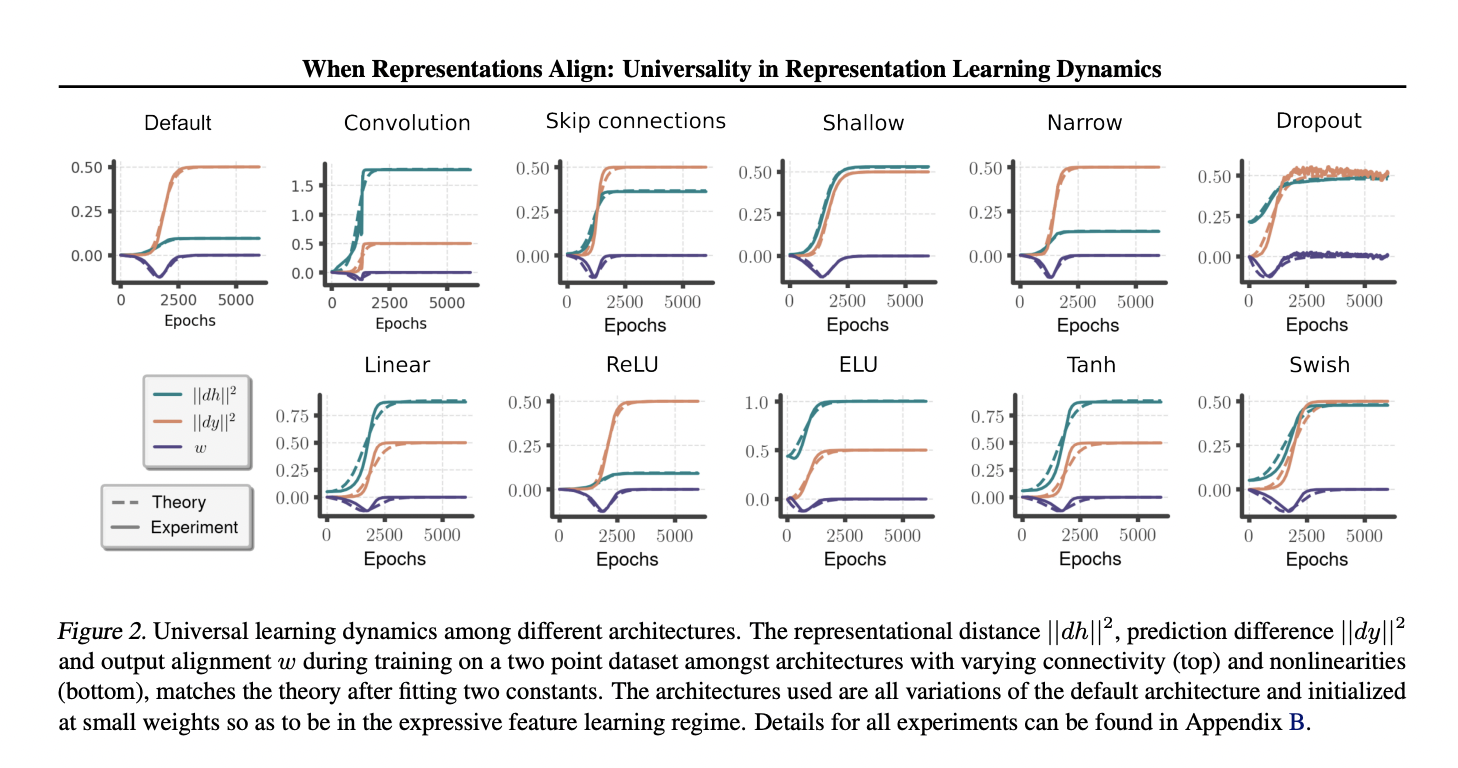
Universal Dynamics of Representation Learning in Deep Neural Networks
Practical Solutions and Value
Deep neural networks (DNNs) have various sizes and structures which influence the neural patterns learned. However, the issue of scalability is a major challenge in deep learning theory. Researchers at the University College London have proposed a method for modeling universal representation learning to explain common phenomena observed in learning systems.
Key Research Findings
The proposed theory looks at the representation dynamics at an intermediate layer, showing that networks naturally learn structured representations, especially when they start with small weights. The effective learning rates vary at different hidden layers, impacting the learning patterns across different architectures.
Practical Applications
This research offers insights into how neural networks learn and can redefine sales processes and customer engagement. It emphasizes the importance of identifying automation opportunities, defining KPIs, selecting suitable AI solutions, and implementing AI usage judiciously.
AI Solutions for Your Company
If you want to evolve your company with AI, stay competitive, and leverage the findings from the University College London research, connect with us at hello@itinai.com for AI KPI management advice and continuous insights into leveraging AI.