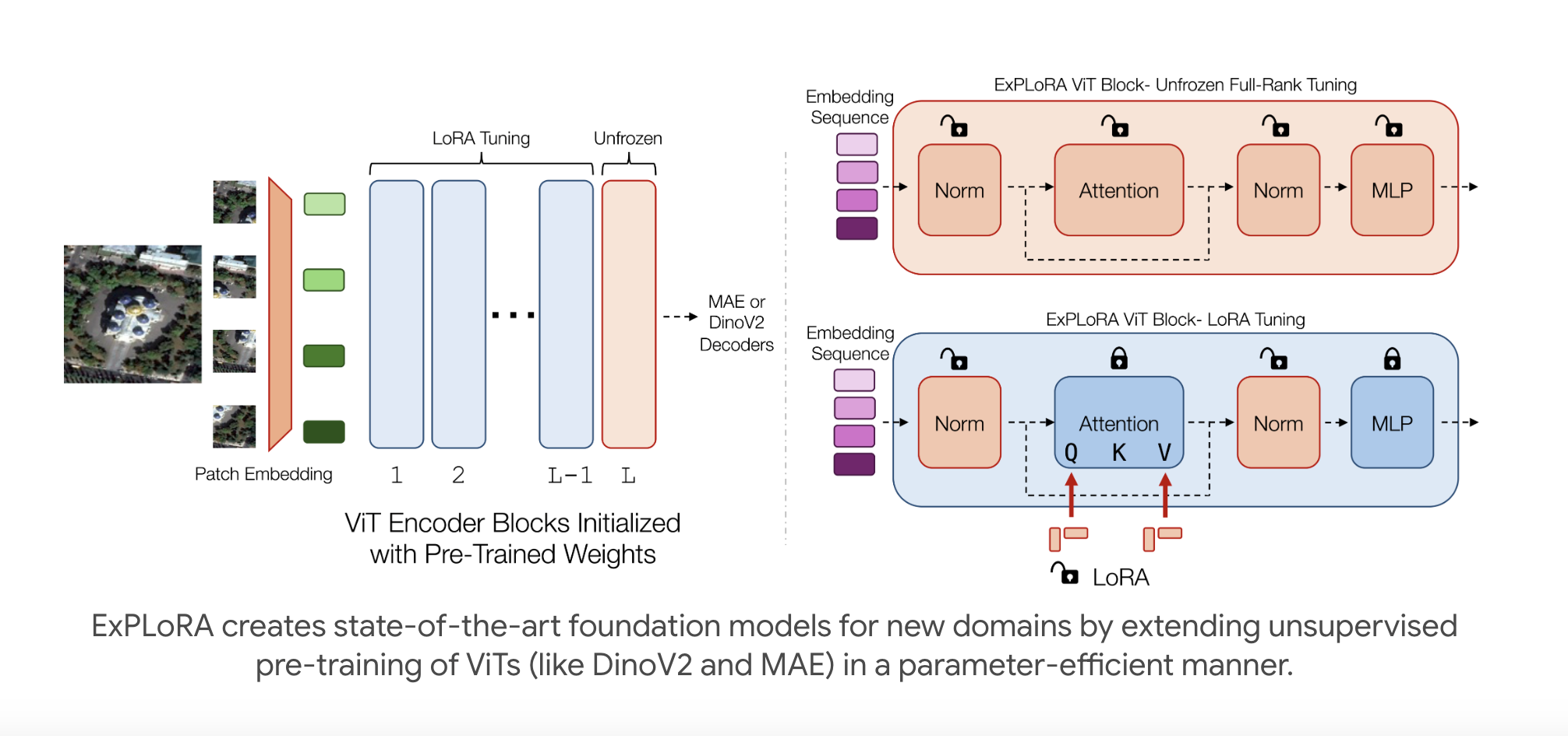
Understanding Parameter-Efficient Fine-Tuning (PEFT)
PEFT methods, such as Low-Rank Adaptation (LoRA), allow large pre-trained models to be adapted for specific tasks using only a small portion (0.1%-10%) of their original weights. This approach is cost-effective and efficient, making it easier to apply these models to new domains without extensive resources.
Advancements in Vision Foundation Models (VFMs)
Models like DinoV2 and Masked Autoencoders (MAE) excel in tasks like classification and segmentation through self-supervised learning (SSL). New domain-specific models, such as SatMAE, are designed for analyzing satellite images. PEFT methods help adapt these large models by updating only a small number of parameters, enhancing their performance across various domains.
Introducing ExPLoRA
Researchers from Stanford University and CZ Biohub have created ExPLoRA, a technique that improves transfer learning for vision transformers (ViTs) when facing domain shifts. By starting with weights from large datasets, ExPLoRA continues unsupervised pre-training in new domains, selectively adjusting only a few layers while using LoRA for the rest. This method boosts satellite image classification accuracy by 8%, using just 6-10% of the parameters compared to traditional models.
Efficiency of MAE and DinoV2
MAE requires full fine-tuning for downstream tasks, which can be resource-heavy. In contrast, DinoV2 offers strong performance without full fine-tuning. ExPLoRA combines pre-trained weights with low-rank adaptations, making it efficient in adapting ViTs to new domains while reducing storage needs and maintaining strong feature extraction capabilities.
Results and Impact
In experiments with satellite imagery, ExPLoRA achieved a top accuracy of 79.2% on the fMoW-RGB dataset, outperforming traditional methods while using only 6% of the parameters. Additional tests on multi-spectral images further demonstrate ExPLoRA’s effectiveness in closing domain gaps and achieving competitive results.
Conclusion
ExPLoRA is a groundbreaking strategy for adapting pre-trained ViT models to various visual domains, including satellite and medical imagery. It overcomes the challenges of costly pre-training by enabling efficient knowledge transfer, achieving superior performance with minimal adjustments. The method significantly enhances transfer learning, showing state-of-the-art results in satellite imagery while using less than 10% of the parameters of previous approaches.
Stay Connected
Check out the Paper and Project. All credit goes to the researchers involved. Follow us on Twitter, join our Telegram Channel, and connect with our LinkedIn Group. If you appreciate our work, subscribe to our newsletter and join our 50k+ ML SubReddit.
Upcoming Event
RetrieveX – The GenAI Data Retrieval Conference on Oct 17, 2023.
Transform Your Business with AI
Stay competitive by leveraging AI solutions. Here’s how:
- Identify Automation Opportunities: Find key areas for AI integration.
- Define KPIs: Measure the impact of AI on business outcomes.
- Select an AI Solution: Choose tools that fit your needs.
- Implement Gradually: Start small, gather data, and expand.
For AI KPI management advice, contact us at hello@itinai.com. For ongoing insights, follow us on Telegram or Twitter.
Enhance Sales and Customer Engagement with AI
Explore solutions at itinai.com.