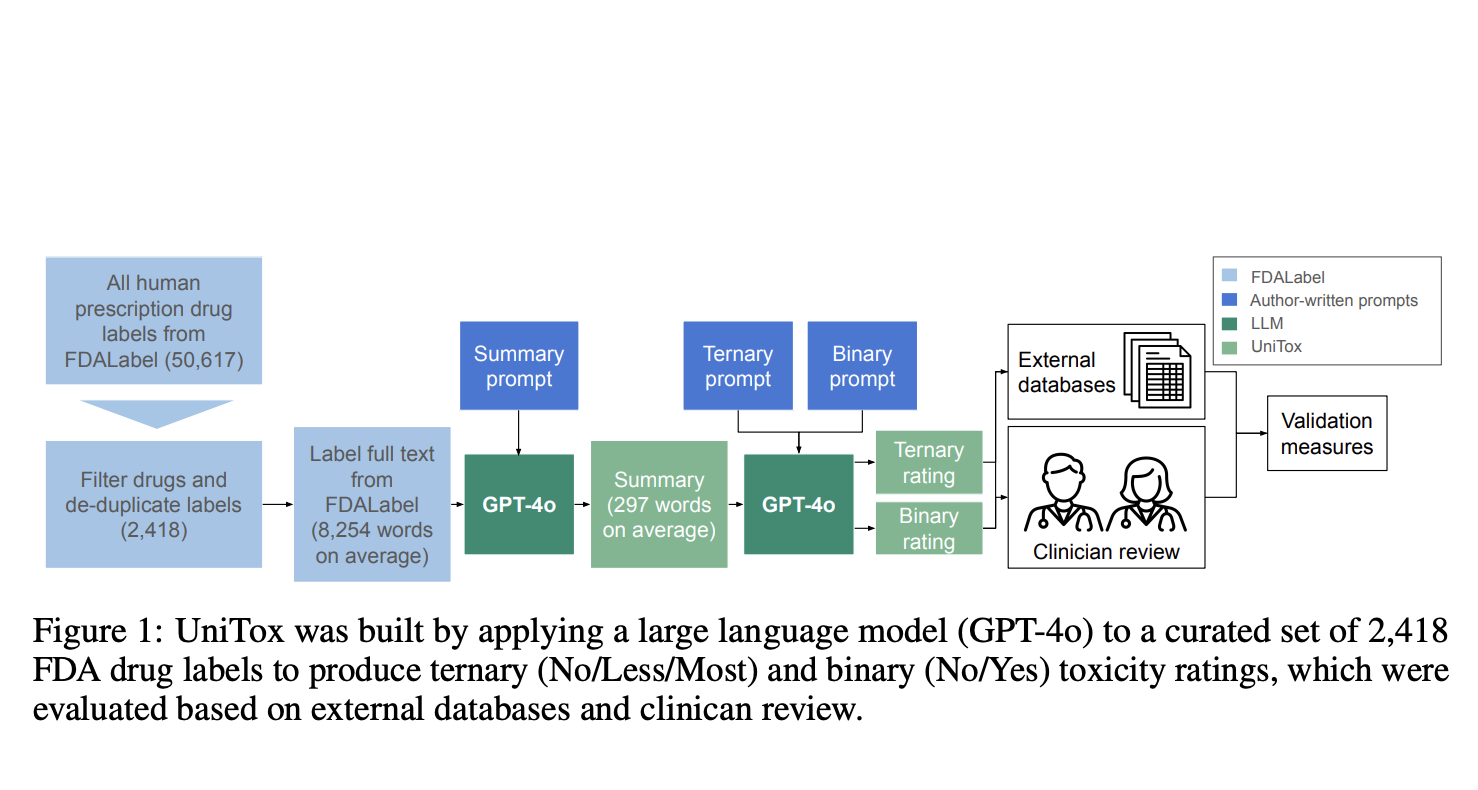
Understanding Drug-Induced Toxicity in Drug Development
Key Challenge in Clinical Trials
Drug-induced toxicity is a significant issue in drug development, leading to many clinical trial failures. While effectiveness is the main reason for these failures, safety concerns account for 24%. Toxicity can impact vital organs like the heart, liver, kidneys, and lungs. Even approved drugs can be withdrawn due to unexpected toxic effects discovered after they hit the market. There is an urgent need for predictive models to identify safer drug candidates early in development.
Limitations of Current Toxicity Datasets
Existing toxicity databases, such as SIDER and LiverTox, often focus on specific organs or rely on laboratory tests that may not reflect real-life effects. Compiling these datasets is labor-intensive and can vary widely in methodologies, leading to inconsistencies. For example, the FDA’s renal toxicity database has over 30% disagreement on certain drugs. Large language models (LLMs) like askFDALabel show promise in improving data extraction from FDA labels, achieving good agreement with human evaluations for cardiotoxicity. However, challenges like scalability and consistency still limit the effectiveness of machine learning models.
Introducing UniTox: A Comprehensive Solution
Researchers from Stanford University and Genmab developed **UniTox**, a thorough dataset containing information on **2,418 FDA-approved drugs**. This dataset summarizes and rates drug-induced toxicities using **GPT-4o** to analyze FDA drug labels. UniTox covers eight types of toxicity, including cardiotoxicity and liver toxicity, making it the largest systematic in vivo database for these issues. Clinicians confirmed the accuracy of the GPT-4o annotations, with concordance rates of **85-96%**.
How UniTox Works
To create UniTox, researchers filtered and cleaned drug labels from the FDALabel database. Using GPT-4o, they produced toxicity summaries and ratings for eight types of toxicity, categorizing them in simple terms. The validation process involved comparing with existing FDA datasets and clinician reviews, achieving strong agreement. Clinicians assessed the model’s outputs for accuracy and alignment with expert knowledge.
Benefits of the UniTox Dataset
The UniTox dataset offers a robust resource for analyzing toxicity. It includes summaries generated by GPT-4o, with classifications in easy-to-understand formats. The average summary condenses lengthy drug labels into **297 words**, facilitating quick comprehension. This dataset reveals important toxicity correlations and patterns across different drug classes.
Conclusion: Advancing Drug Toxicity Prediction
The study showcases the efficiency of GPT-4o in summarizing complex drug labels and producing accurate toxicity ratings. The UniTox dataset, which includes **2,418 drugs**, fills important gaps in toxicity evaluation across various organ systems. Despite some challenges, UniTox demonstrates the potential of LLMs in enhancing drug toxicity prediction and supporting ongoing research.
Get Involved and Stay Updated
For more information, check out the paper and dataset. Follow us on Twitter and join our Telegram Channel and LinkedIn Group. Don’t forget to join our **60k+ ML SubReddit** for continuous updates.
Transform Your Company with AI
Discover how AI can enhance your business operations. Here are some practical steps:
– **Identify Automation Opportunities**: Find key areas that can benefit from AI.
– **Define KPIs**: Ensure measurable impacts from your AI initiatives.
– **Select an AI Solution**: Choose tools that fit your needs and allow customization.
– **Implement Gradually**: Start small, gather data, and expand AI usage thoughtfully.
For AI KPI management advice, connect with us at **hello@itinai.com**. Stay tuned for more insights on leveraging AI through our Telegram channel **t.me/itinainews** or Twitter **@itinaicom**.