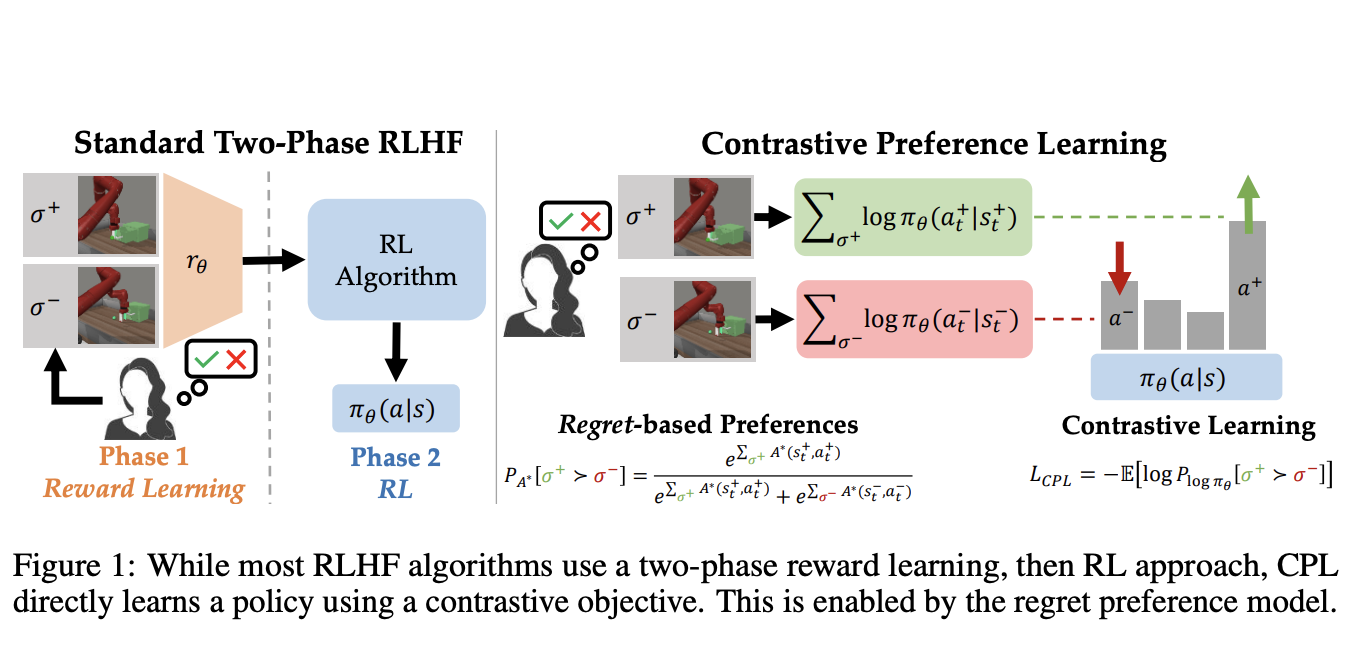
Addressing Challenges in AI Research with Contrastive Preference Learning (CPL)
Practical Solutions and Value
Aligning AI models with human preferences in high-dimensional tasks is complex. Traditional methods like Reinforcement Learning from Human Feedback (RLHF) face challenges due to computational complexity and limitations in real-world applications.
A novel algorithm, Contrastive Preference Learning (CPL), directly optimizes behavior from human feedback, bypassing the need for learning a reward function. This approach simplifies the learning process, making it applicable to high-dimensional and sequential decision-making problems.
CPL offers a more scalable and computationally efficient solution compared to traditional RLHF methods, broadening the scope of tasks that can be effectively tackled using human feedback.
Evaluation and Impact
CPL demonstrates effectiveness in learning policies from high-dimensional and sequential data, often surpassing traditional RL-based methods. It achieves higher success rates in various tasks and shows significant improvements in computational efficiency.
By directly optimizing policies through a contrastive objective based on a regret preference model, CPL offers a more efficient and scalable solution for aligning models with human preferences, particularly impactful for high-dimensional and sequential tasks.
AI Implementation and Business Impact
For companies looking to evolve with AI, CPL provides a framework for leveraging human feedback to improve AI models. It offers practical steps for identifying automation opportunities, defining KPIs, selecting AI solutions, and implementing AI usage gradually.
For AI KPI management advice and insights into leveraging AI, connect with us at hello@itinai.com or stay tuned on our Telegram t.me/itinainews or Twitter @itinaicom.