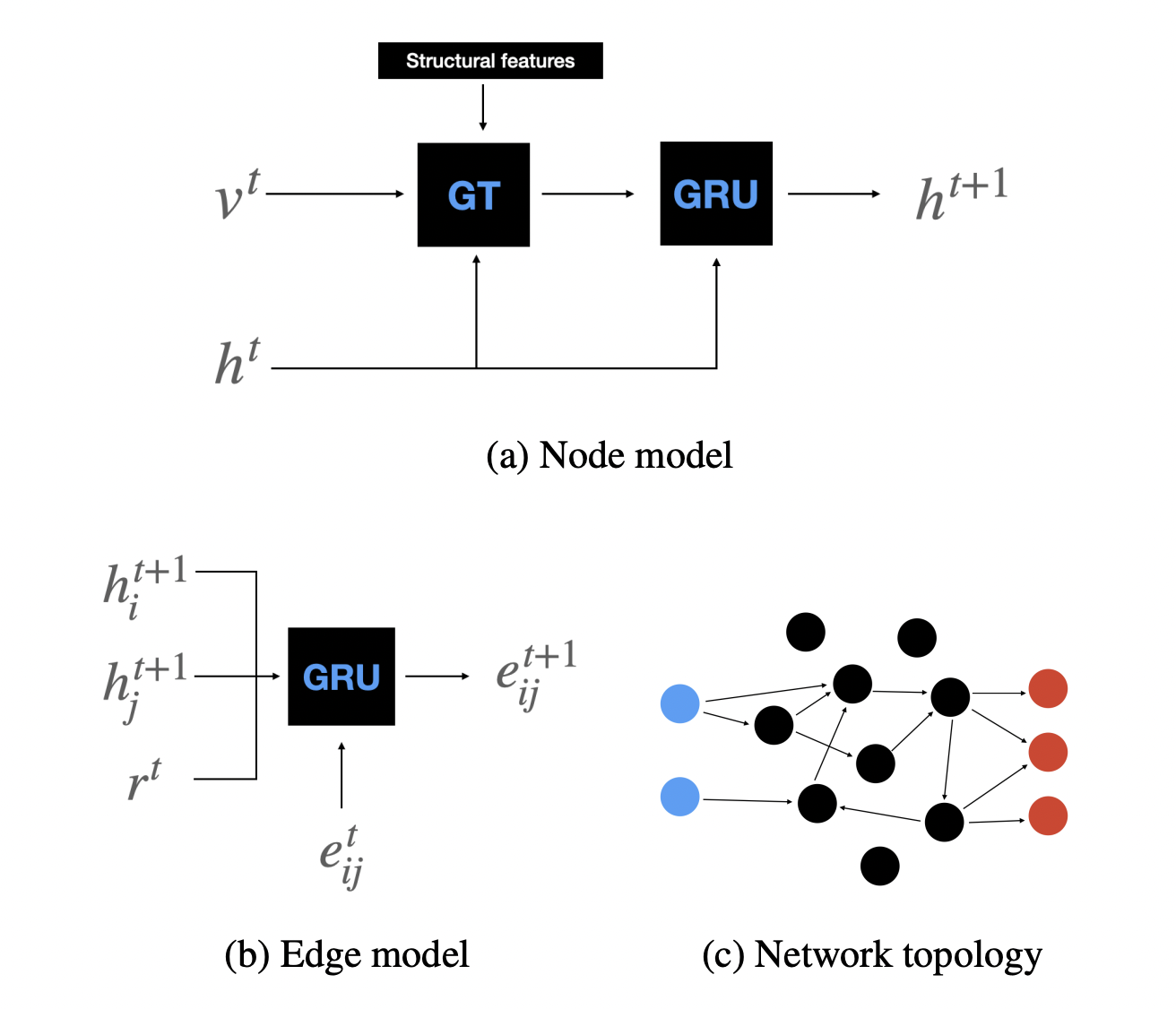
Enhancing Adaptability of Artificial Neural Networks
Addressing Limitations
Artificial neural networks (ANNs) traditionally struggle with adaptability and plasticity in dynamic environments, hindering their effectiveness in real-time applications like robotics and adaptive systems.
Practical Solutions
Researchers have introduced Lifelong Neural Developmental Programs (LNDPs), a novel approach that enables ANNs to self-organize, learn from experiences, and adapt throughout their lifetime. LNDPs utilize a graph transformer architecture combined with Gated Recurrent Units (GRUs) to enable neurons to dynamically adapt their structure and connectivity, addressing the limitations of static and pre-defined developmental phases.
Key Components
LNDPs involve node and edge models, synaptogenesis, and pruning functions integrated into a graph transformer layer. This allows for dynamic adaptation of the network’s structure and connectivity.
Proven Effectiveness
LNDPs have demonstrated significant improvements in adaptation speed and learning efficiency across various reinforcement learning tasks, showcasing their potential for developing adaptable and self-organizing AI systems.
Practical Implementation
For companies looking to leverage AI, the adoption of LNDPs can redefine work processes, enhance customer engagement, and provide automation opportunities, ultimately redefining business outcomes and sales processes.
Get in Touch
For AI KPI management advice and continuous insights into leveraging AI, connect with us at hello@itinai.com or stay tuned on our Telegram t.me/itinainews and Twitter @itinaicom.