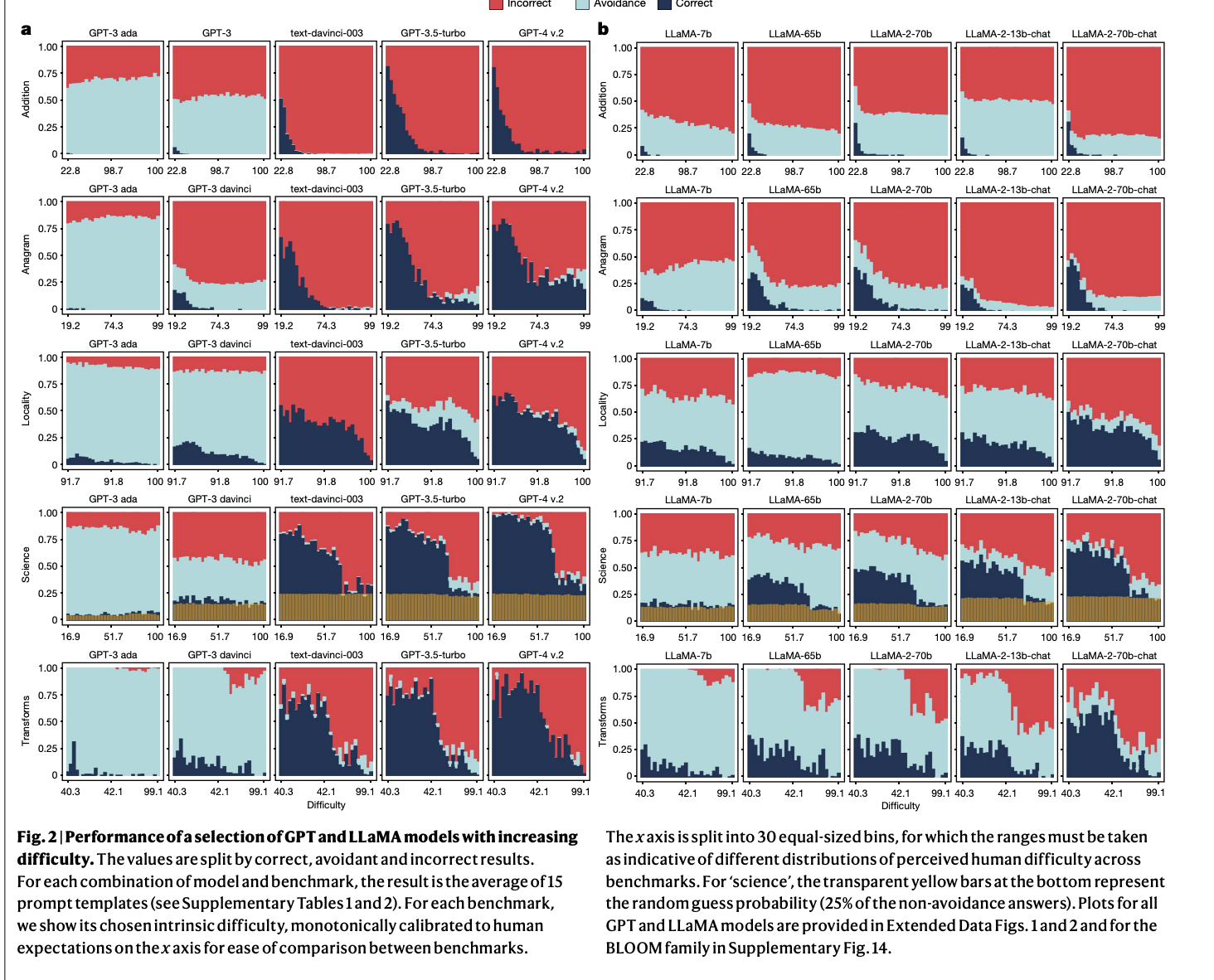
Practical Solutions and Value of Reliability in Large Language Models (LLMs)
Understanding Limitations and Improving Reliability
The research evaluates the reliability of large language models (LLMs) like GPT, LLaMA, and BLOOM across various domains such as education, medicine, science, and administration. As these models become more prevalent, it is crucial to understand their limitations to avoid misleading outputs.
Challenges of Scaling Up LLMs
As LLMs increase in size and complexity, their reliability may not necessarily improve. Existing methodologies to address reliability concerns include scaling up the models, which involves increasing parameters, training data, and computational resources.
Introducing the ReliabilityBench Framework
The researchers introduced the ReliabilityBench framework to systematically evaluate LLMs across five domains, revealing strengths and weaknesses. This approach offers a deeper understanding of the capabilities of LLMs.
Improving LLM Performance and Reliability
While strategies like scaling and shaping enhance LLM performance on complex questions, they often degrade reliability for simpler tasks. Shaped-up models are more prone to producing incorrect yet plausible answers, affecting user confidence in their outputs.
Paradigm Shift in Designing LLMs
The study highlights the need for a paradigm shift in designing LLMs. The proposed ReliabilityBench framework provides a robust evaluation methodology, emphasizing the importance of ensuring consistent model performance across all difficulty levels.
AI Solutions for Business Transformation
Discover how AI can redefine your way of work by identifying automation opportunities, defining KPIs, selecting suitable AI solutions, and implementing gradually. Connect with us for AI KPI management advice and continuous insights into leveraging AI.
Redefining Sales Processes with AI
Explore how AI can redefine your sales processes and customer engagement, and discover solutions at itinai.com for enhancing your business operations.