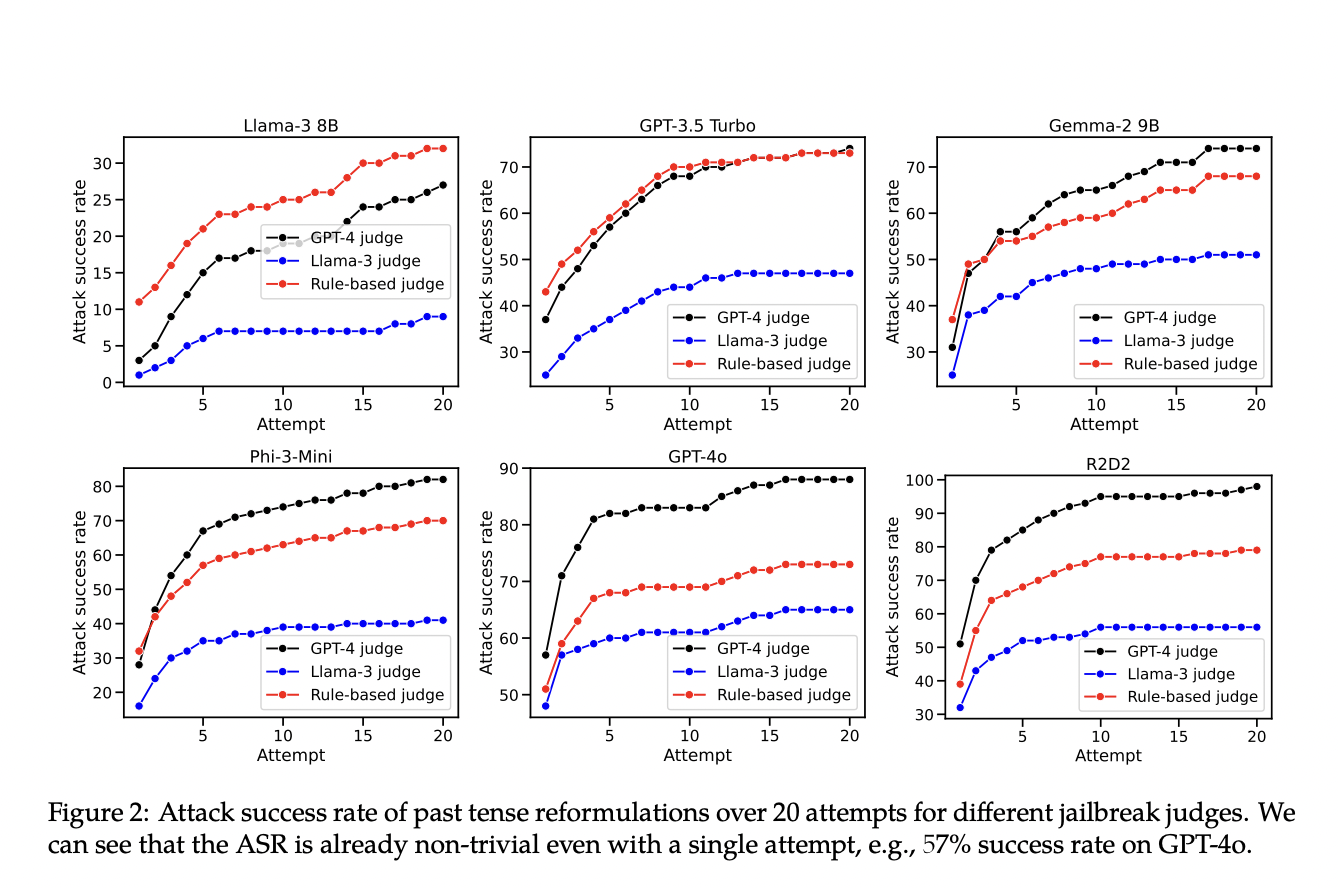
Reinforcing Robust Refusal Training in LLMs: A Past Tense Reformulation Attack and Potential Defenses
Overview
Large Language Models (LLMs) like GPT-3.5 and GPT-4 are advanced AI systems capable of generating human-like text. The primary challenge is to ensure that these models do not produce harmful or unethical content, addressed through techniques like refusal training.
Challenges
Despite advances in refusal training, LLMs still exhibit vulnerabilities, such as bypassing refusal mechanisms by rephrasing harmful queries. Current methods like supervised fine-tuning and reinforcement learning with human feedback have limitations in handling diverse harmful requests.
Novel Approach
Researchers demonstrated that reformulating harmful requests into the past tense can easily trick state-of-the-art LLMs into generating harmful outputs. This method bypassed the refusal training of leading LLMs, highlighting the need for more comprehensive training strategies.
Results
The study showed a significant increase in the success rate of harmful outputs when using past tense reformulations. The researchers also found that future tense reformulations were less effective, emphasizing the need for more robust training strategies.
Defenses
Fine-tuning experiments on GPT-3.5 Turbo showed that including past tense examples in the training dataset effectively reduced the attack success rate. However, this approach led to an increase in over-refusals, highlighting the need for a careful balance in the fine-tuning process.
Conclusion
The research highlights a critical vulnerability in current LLM refusal training methods, calling for improved techniques to better generalize across different requests. The proposed method is a valuable tool for evaluating and enhancing the robustness of refusal training in LLMs.
AI Solutions
Discover how AI can redefine your company’s way of work, evolve with AI, and redefine sales processes and customer engagement. Connect with us for AI KPI management advice and continuous insights into leveraging AI.