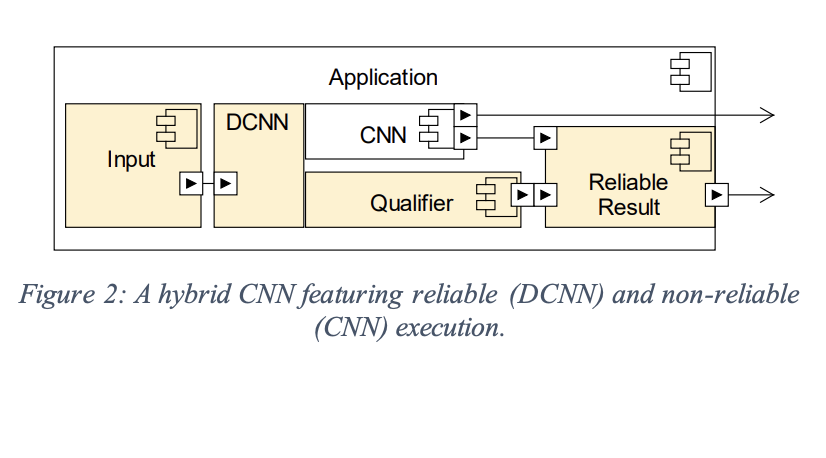
Practical AI Solution: Redundancy in AI
Minimizing Computational Overhead in Reliable Execution
The challenge of ensuring the reliability and safety of AI models, especially in safety-critical applications like autonomous driving and medical diagnosis, has been addressed by researchers at the Institute of Embedded Systems Zurich University of Applied Sciences Winterthur, Switzerland. They have developed a method called “redundant execution” to optimize resources while ensuring dependable performance in embedded edge-AI devices.
The method integrates reliable model execution with non-reliable execution, focusing on additional computational expense only where necessary. By leveraging concepts from reliability engineering and multicore architectures, this approach extends the application scope of AI accelerators, particularly in edge-AI devices.
The hybrid (convolutional) neural network designed through redundant execution techniques and conventional CNN architectures ensures the reliable execution of critical operations while conserving computational resources. Experimental results indicate that this approach reduces the necessary reliable execution to limits determined by a dependable model, conserving both footprint and computational power.
The proposed method significantly contributes to achieving safe and dependable AI systems, with scope for further extension to more complex neural network architectures and applications with additional optimization.
If you want to evolve your company with AI, stay competitive, and use Redundancy in AI, consider the AI Sales Bot from itinai.com/aisalesbot designed to automate customer engagement 24/7 and manage interactions across all customer journey stages.
For AI KPI management advice and continuous insights into leveraging AI, connect with us at hello@itinai.com or stay tuned on our Telegram t.me/itinainews or Twitter @itinaicom.