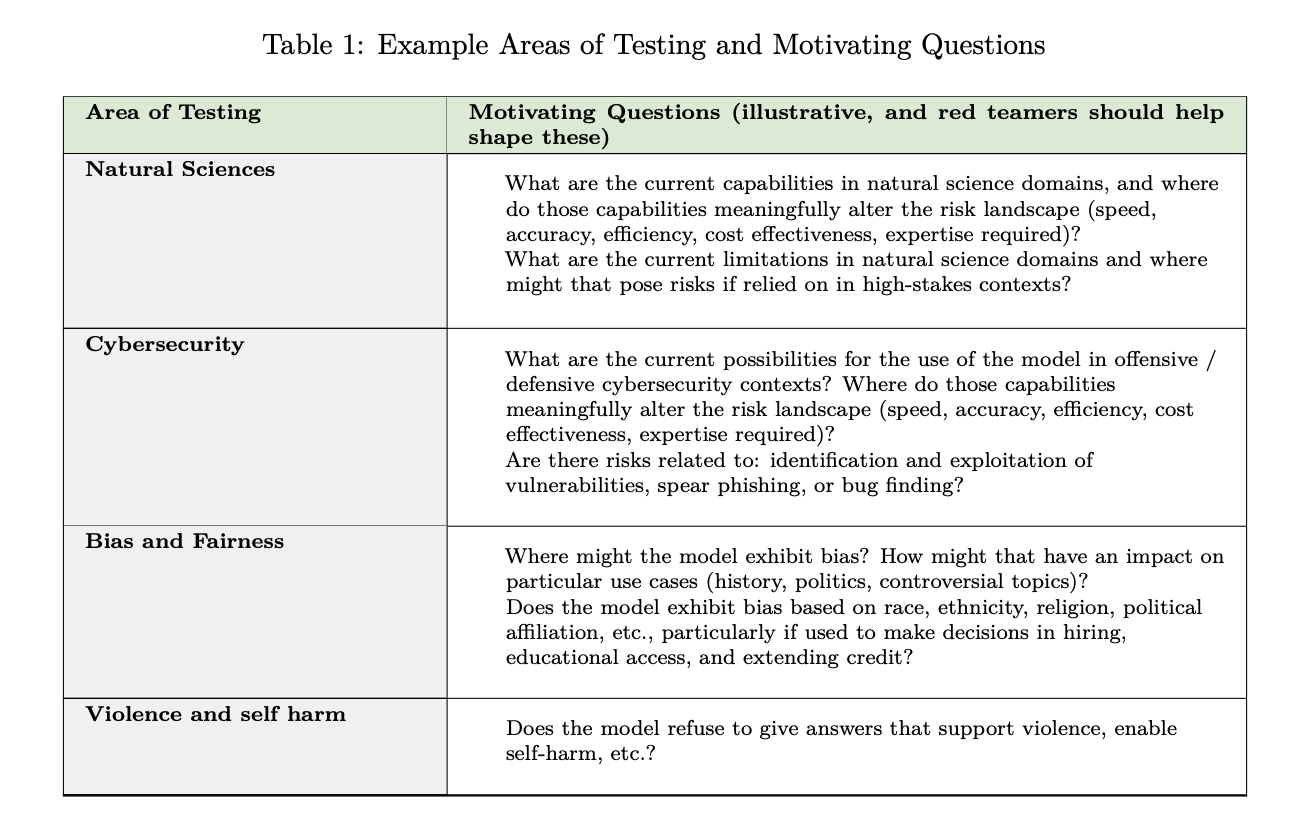
Understanding Red Teaming in AI
Red teaming is crucial for evaluating AI risks. It helps find new threats, spot weaknesses in safety measures, and improve safety metrics. This process builds public trust and enhances the credibility of AI risk assessments.
OpenAI’s Red Teaming Approach
This paper explains how OpenAI uses external red teaming to assess AI model risks. By working with experts, they gain insights into both strengths and weaknesses of their models. While focusing on OpenAI, the principles discussed can guide other organizations in incorporating human red teaming into their AI evaluations.
A Foundation for AI Safety Practices
Red teaming has become essential in AI safety, with OpenAI adopting it since the launch of DALL-E 2 in 2022. It systematically tests AI systems for vulnerabilities and risks, informing safety practices in AI labs, and aligning with global policy initiatives on AI safety.
Key Benefits of External Red Teaming
External red teaming provides immense value for AI safety assessments. It identifies new risks from advancements like GPT-4o’s ability to mimic voices. It also stress-tests existing defenses, uncovering vulnerabilities such as bypassing safeguards in DALL-E. By bringing in expert knowledge, red teaming enhances risk assessments and ensures objective evaluations.
Diverse Testing Methods
Red teaming methods vary, adapting to the complexity of AI systems. Developers outline the scope and criteria for testing, using both manual and automated techniques. OpenAI combines these methods in System Cards to improve evaluations for frontier models.
Steps for Effective Red Teaming
Creating a successful red teaming campaign involves strategic planning. Key steps include:
- Defining the team based on testing goals.
- Determining which model versions are accessible.
- Synthesizing the data gathered to create thorough evaluations.
Comprehensive Testing and Evolving Strategies
A thorough red teaming process tests various scenarios and use cases to address different AI risks. Prioritizing areas based on anticipated capabilities and context ensures a structured approach. External teams provide fresh perspectives that enhance the overall testing process.
Shifting to Automated Evaluations
Transitioning from human red teaming to automated evaluations is vital for scalable AI safety. Post-campaign analyses help identify whether new guidelines are required, while insights inform future assessments and enhance understanding of user interactions with AI models.
Challenges and Considerations
Despite its value, red teaming has limitations. Findings can quickly become outdated as models evolve. Additionally, the process can be resource-intensive and may expose participants to harmful content. Fairness issues can arise if red teamers gain early access to models, and rising model complexity requires advanced expertise for effective evaluations.
Conclusion
This paper emphasizes the role of external red teaming in AI risk assessment and the importance of ongoing evaluations to enhance safety. Engaging diverse domain experts is crucial for identifying risks proactively. However, integrating public perspectives and accountability measures is essential for comprehensive AI assessments.
Explore more: Check out the full paper. Follow us on Twitter, join our Telegram Channel, and LinkedIn Group for updates. If you appreciate our work, subscribe to our newsletter and join our 55k+ ML SubReddit.
To advance your organization with AI and stay competitive, consider the benefits of Red Teaming for AI. Learn how AI can transform your work processes:
- Identify Automation Opportunities.
- Define KPIs for measurable impacts.
- Select the right AI solutions tailored to your needs.
- Implement gradually with pilot projects.
For AI KPI management advice, connect with us at hello@itinai.com. Stay updated on AI insights through our Telegram at t.me/itinainews or Twitter @itinaicom.
Discover how AI can enhance your sales and customer engagement! Visit itinai.com for more solutions.