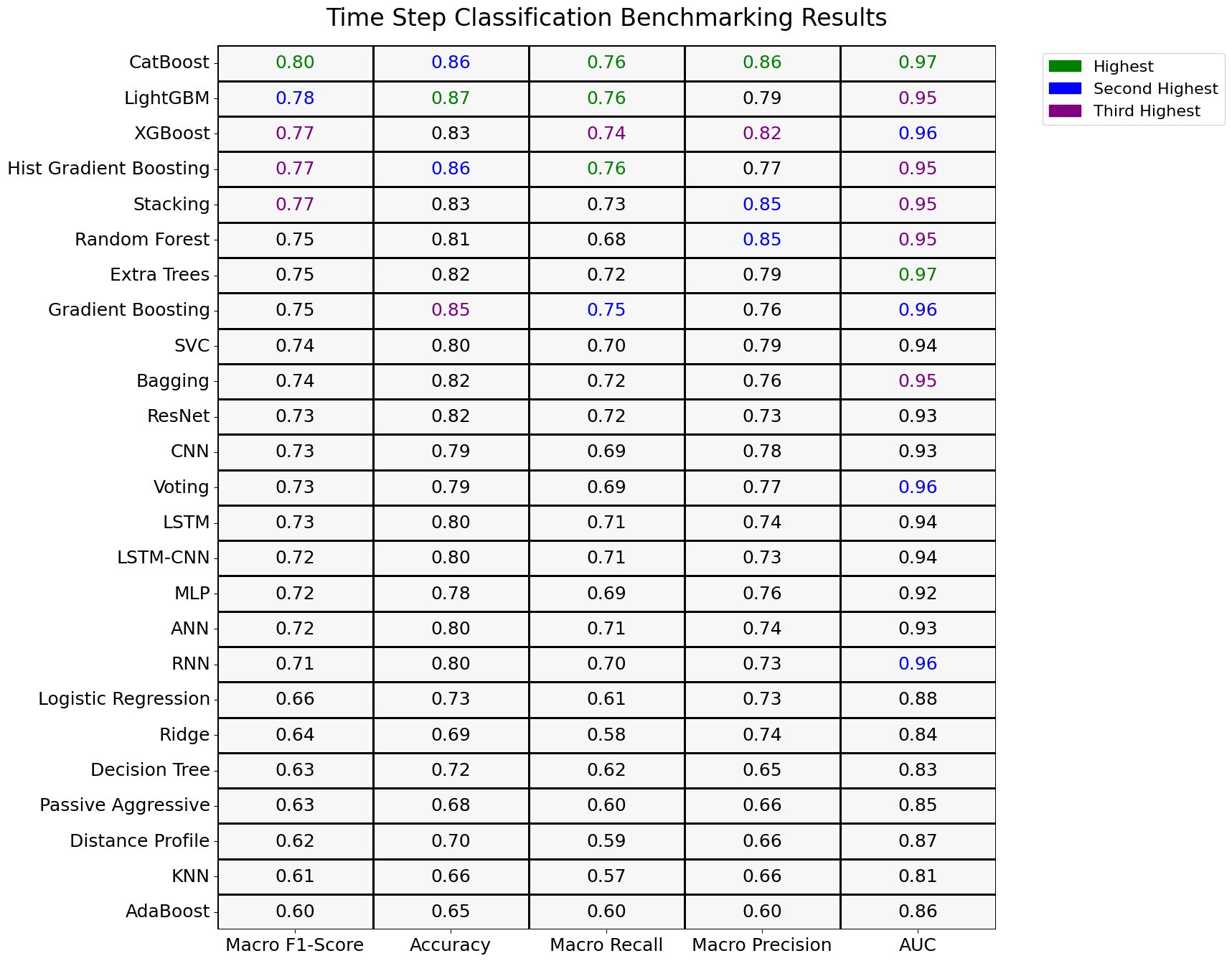
Practical Solutions for Time Series Step Classification
Overview of Study
Ready Tensor conducted a study to improve time series step classification accuracy by evaluating 25 machine learning models across diverse datasets.
Datasets Summary
The study used real-world and synthetic datasets with varying time frequencies and series lengths to represent different time series classification tasks.
Evaluated Models
The 25 models were categorized into Machine Learning models, Neural Network models, and a unique Distance Profile model, each offering distinct approaches to time series classification.
Results and Insights
The study highlighted top-performing models like CatBoost, LightGBM, and XGBoost, as well as strong contenders and baseline performers, providing valuable insights for model selection based on dataset characteristics.
Conclusion
Ready Tensor’s benchmarking study offers a detailed comparison of models, emphasizing the effectiveness of boosting algorithms for managing time series data and guiding researchers and practitioners in selecting appropriate models.
For AI KPI management advice and insights into leveraging AI, contact us at hello@itinai.com or follow us on Telegram and Twitter for continuous updates.