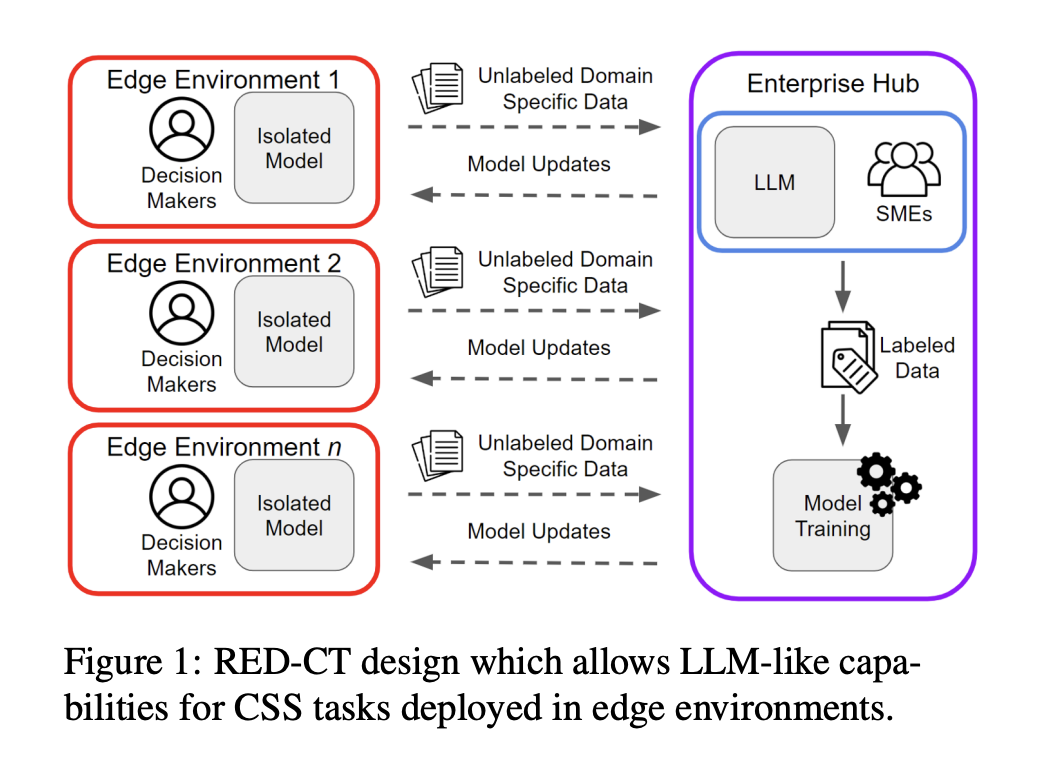
Practical Solutions for Computational Social Science (CSS) Tasks
Challenges in Deploying Large Language Models (LLMs)
Large language models (LLMs) have revolutionized CSS by enabling rapid and sophisticated text analysis, but their integration into practical applications remains complex due to high costs, data privacy concerns, and network infrastructure limitations.
Addressing LLM Deployment Challenges
The Rapid Edge Deployment for CSS Tasks (RED-CT) system offers an innovative solution to deploy edge classifiers using LLM-labeled data with minimal human annotation. This system is designed for resource-constrained environments, optimizing the use of LLMs while reducing their dependency.
Performance and Results
The RED-CT system demonstrated remarkable results across various CSS tasks, outperforming LLM-generated labels in seven of the eight tasks tested. It showed significant gains in tasks like stance detection and misinformation identification, highlighting its potential for real-world applications.
Value of RED-CT System
The RED-CT system offers a powerful and efficient solution for deploying edge classifiers in CSS tasks, reducing the dependency on LLMs and enhancing performance in key areas. It provides a practical and scalable solution for CSS applications in environments with limited resources.
Evolve Your Company with AI
Stay competitive and redefine your way of work by leveraging the Rapid Edge Deployment for CSS Tasks (RED-CT) system. Identify automation opportunities, define KPIs, select an AI solution, and implement gradually to evolve your business with AI.
AI KPI Management and Insights
For AI KPI management advice and continuous insights into leveraging AI, connect with us at hello@itinai.com or stay tuned on our Telegram t.me/itinainews or Twitter @itinaicom.
Redefined Sales Processes and Customer Engagement
Discover how AI can redefine your sales processes and customer engagement. Explore solutions at itinai.com.