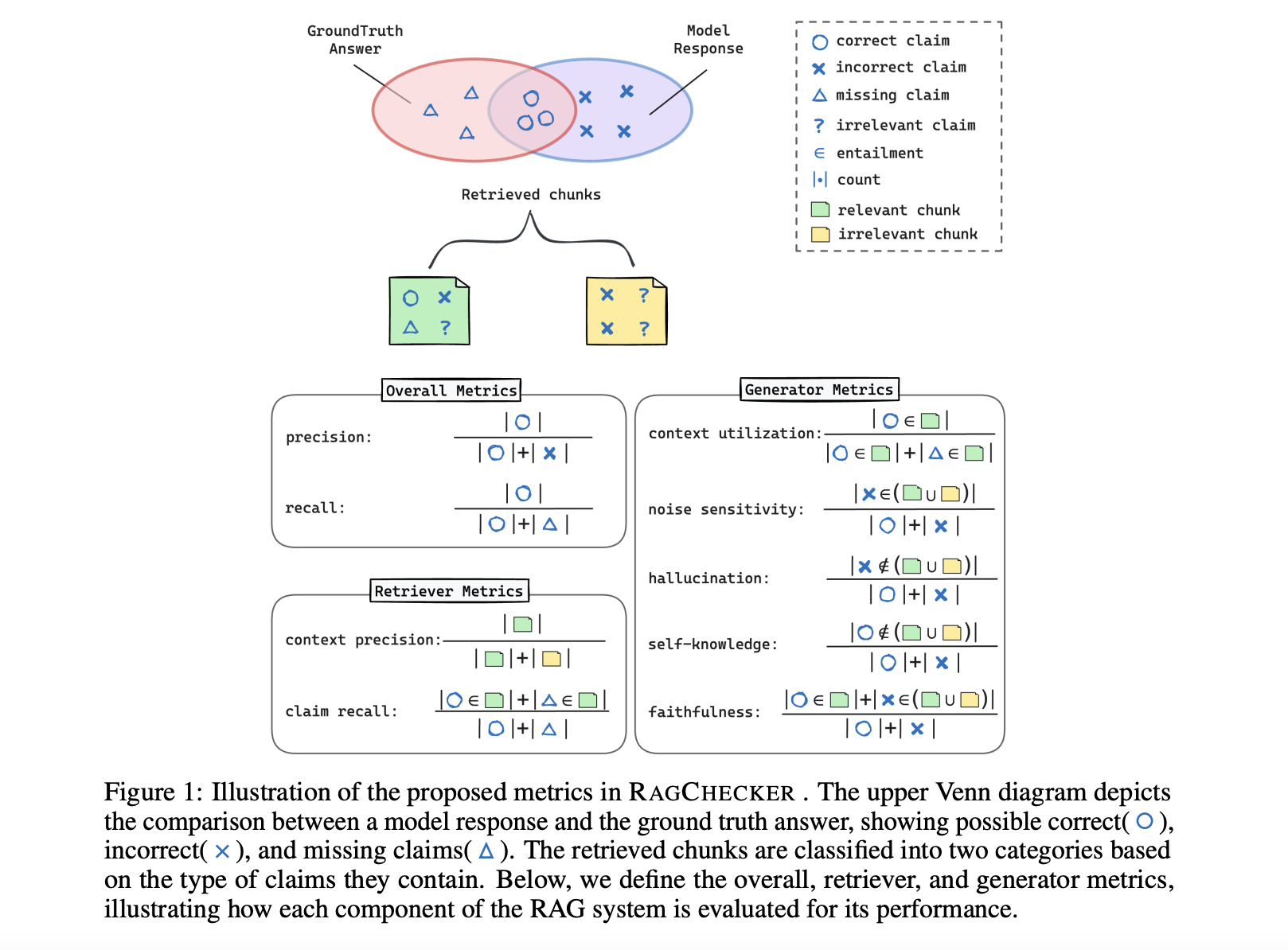
Practical Solutions and Value of RAGChecker for AI Evolution
Enhancing RAG Systems with RAGChecker
Retrieval-Augmented Generation (RAG) is a cutting-edge approach in natural language processing (NLP) that significantly enhances the capabilities of Large Language Models (LLMs) by incorporating external knowledge bases. RAG systems address challenges in precision and reliability, particularly in critical domains like legal, medical, and financial.
Challenges in Evaluating RAG Systems
Evaluating RAG systems poses significant challenges due to their modular nature and the need for more granularity in assessment metrics. Existing methods often fail to capture the complex interactions between the retriever and generator components, resulting in incomplete and inaccurate evaluations.
Introducing RAGChecker for Comprehensive Evaluation
RAGChecker is a novel evaluation framework designed to comprehensively analyze RAG systems. It incorporates diagnostic metrics to evaluate the retrieval and generation processes at a fine-grained level, offering actionable insights for the development of more effective RAG systems.
Key Insights and Practical Recommendations
RAGChecker’s analysis of RAG systems has revealed key insights, such as the impact of retriever quality and generator size on overall performance. It also provides practical recommendations for optimizing the retriever and generator components to enhance system performance and reliability.
Advancing AI Evolution with RAGChecker
RAGChecker represents a significant advancement in evaluating Retrieval-Augmented Generation systems, offering detailed and reliable assessments of the retriever and generator components. It provides critical guidance for developing more effective RAG systems, driving future improvements in the design and application of these systems.