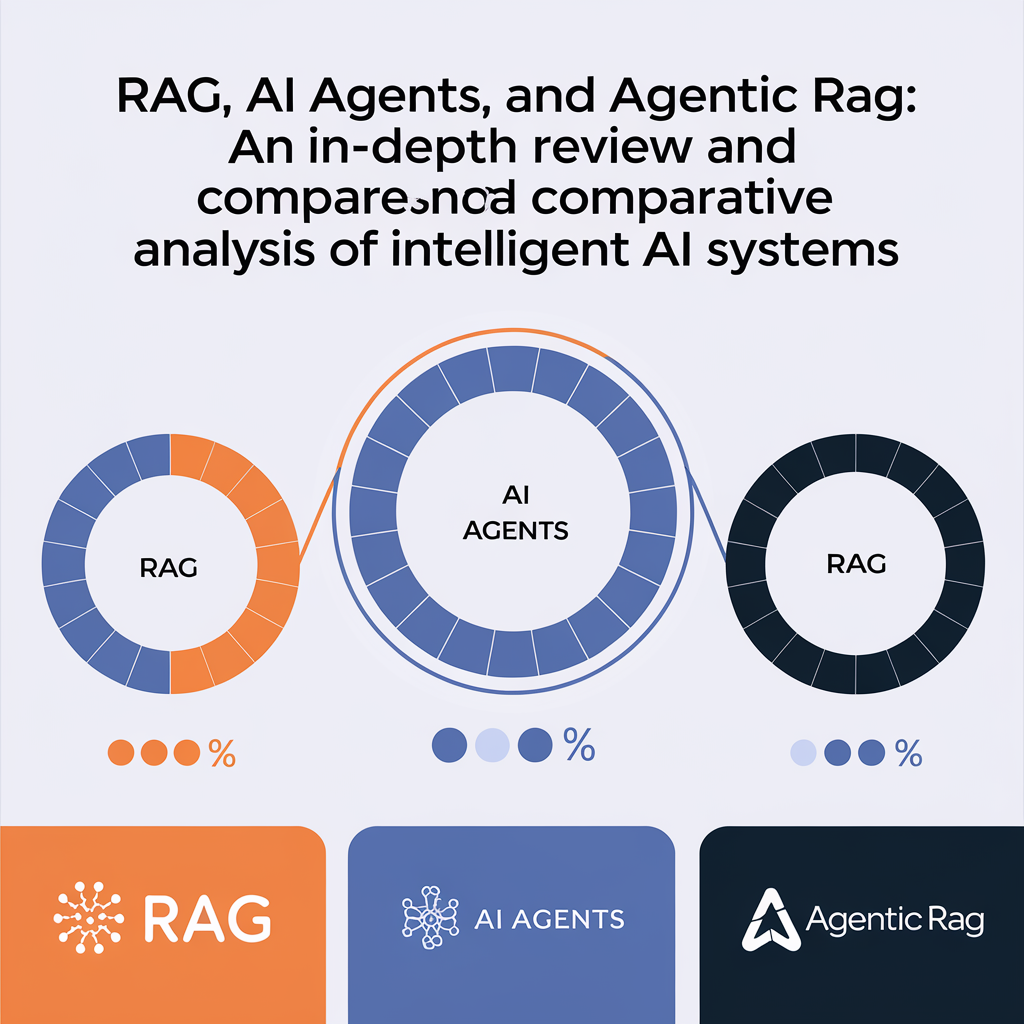
What is Retrieval-Augmented Generation (RAG)?
RAG enhances text generation by retrieving real-time information from external sources, improving accuracy and relevance.
RAG Architecture and Workflow
RAG combines a retriever that searches external knowledge bases with a generator that processes retrieved data to produce responses.
Understanding Agents in AI
Agents are autonomous entities in AI that perform actions based on inputs, ranging from simple rule-based systems to complex decision-making models.
Role of Agents in AI Systems
AI agents automate tasks, optimize processes, and make decisions, with types including reactive, cognitive, and collaborative agents.
Agentic RAG: A Hybrid Approach
Agentic RAG merges Retrieval-Augmented Generation with AI Agents, combining dynamic retrieval with autonomous decision-making.
Concept of Agentic RAG
Agentic RAG uses intelligent agents to control real-time retrieval tasks, enhancing generation and decision-making processes.
Applications of Agentic RAG
Agentic RAG enables dynamic content generation, real-time decision-making, and multi-agent collaborative systems for diverse applications.
Comparative Analysis: RAG, Agents, and Agentic RAG
Agentic RAG combines the strengths of RAG and agents, providing real-time decisions and dynamic content generation.
Conclusion
RAG, Agents, and Agentic RAG offer unique advancements in AI, with Agentic RAG pushing the boundaries of real-time decision-making and dynamic content generation.