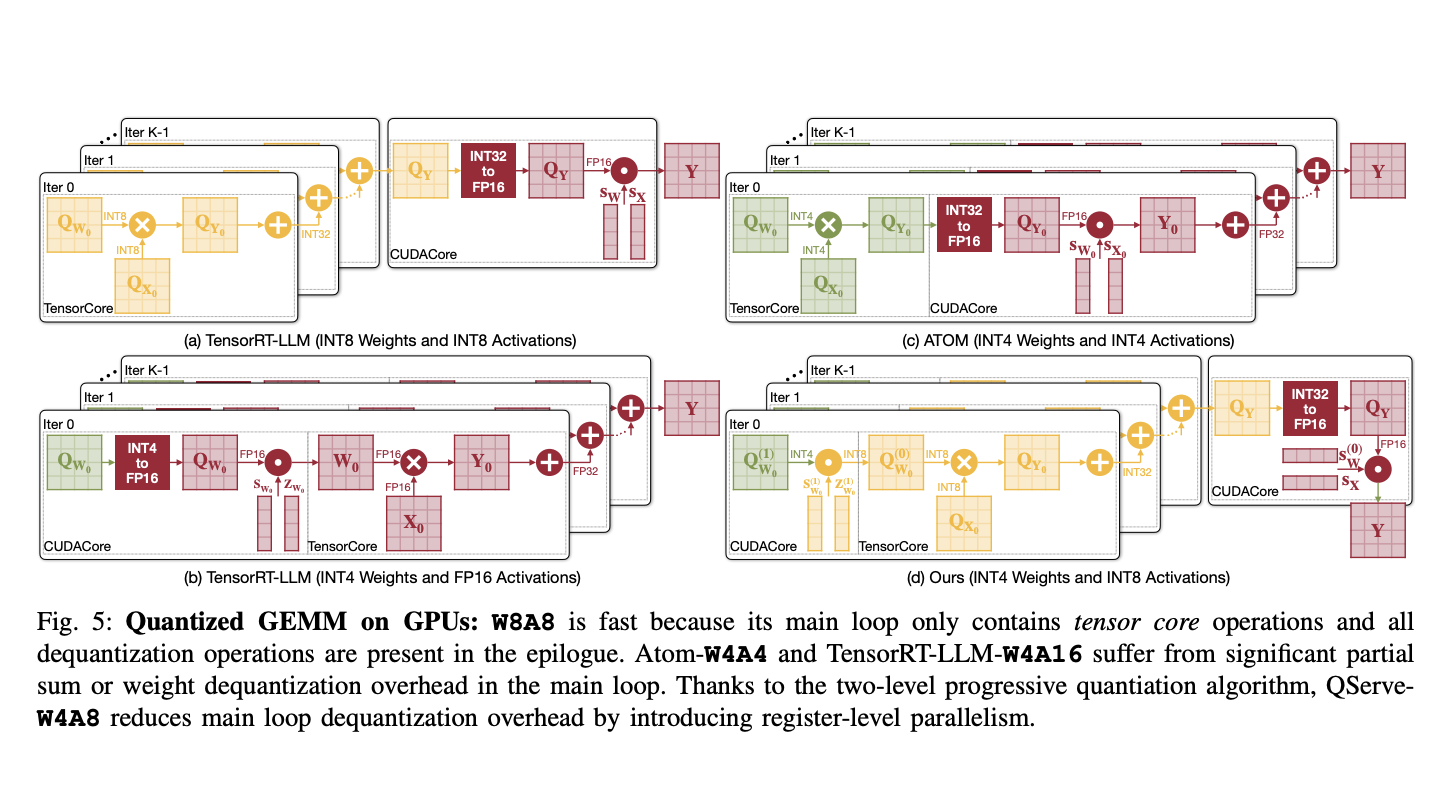
Practical Solutions for Large Language Model Deployment
Quantization and Model Performance
Quantization simplifies data for quicker computations and more efficient model performance. However, deploying large language models (LLMs) is complex due to their size and computational intensity.
Introducing the QoQ Algorithm
The Quattuor-Octo-Quattuor (QoQ) algorithm by researchers from MIT, NVIDIA, UMass Amherst, and MIT-IBM Watson AI Lab refines quantization using progressive group quantization, mitigating accuracy losses. This ensures computations are adapted to current-generation GPUs.
Two-Stage Quantization Process
The QoQ algorithm utilizes a two-stage quantization process, enabling operations on INT8 tensor cores and incorporating SmoothAttention to optimize performance further.
QServe System for Efficient Deployment
The QServe system maximizes the efficiency of LLMs, integrating seamlessly with GPU architectures and reducing quantization overhead by focusing on compute-aware weight reordering and fused attention mechanisms.
Performance and Results
Performance evaluations of the QoQ algorithm show substantial improvements, with throughput enhancements of up to 3.5 times compared to previous methods. QoQ and QServe significantly reduce the cost of LLM serving.
Evolve Your Company with AI
Use QoQ and QServe to redefine your way of work. Identify automation opportunities, define KPIs, choose AI solutions that align with your needs, and implement gradually. Connect with us at hello@itinai.com for AI KPI management advice and continuous insights into leveraging AI.
Spotlight on a Practical AI Solution: AI Sales Bot
The AI Sales Bot from itinai.com/aisalesbot automates customer engagement 24/7 and manages interactions across all customer journey stages, redefining sales processes and customer engagement.