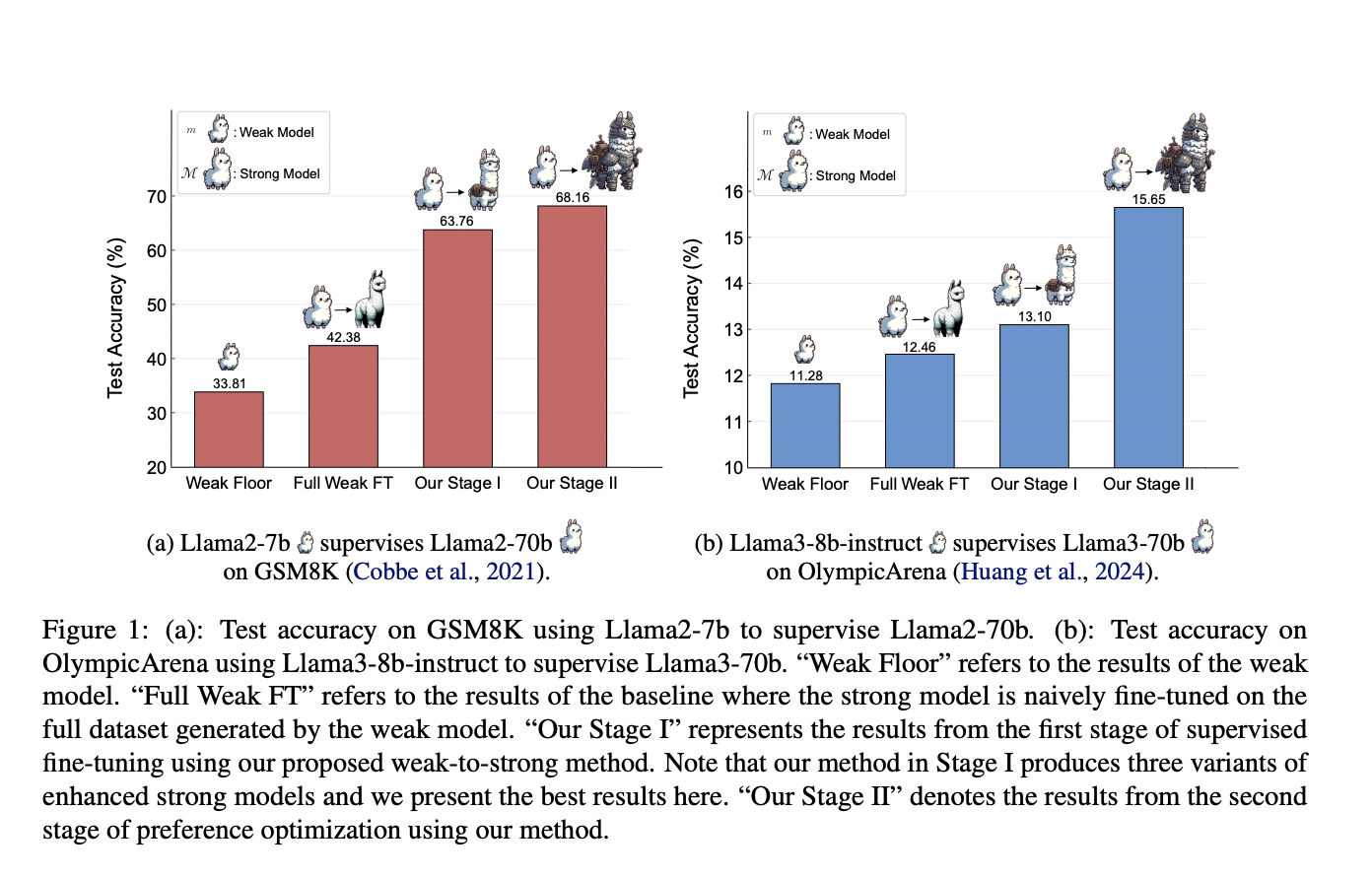
Progressive Learning Framework for Enhancing AI Reasoning through Weak-to-Strong Supervision
Practical Solutions and Value Highlights
As AI capabilities surpass human-level abilities, providing accurate supervision becomes challenging. Weak-to-strong learning offers potential benefits but needs testing for complex reasoning tasks.
Researchers have developed a progressive learning framework that allows strong models to refine their training data autonomously, leading to significant improvements in reasoning abilities. This framework paves the way for enhanced AI reasoning strategies and aligns with human values through supervised fine-tuning and preference optimization.
The weak-to-strong training method aims to maximize the use of weak data and enhance the strong model’s abilities. It refines the strong model by learning from the weak model’s mistakes, resulting in an improved model with better generalization on simpler problems and scalability in realistic scenarios.
This self-directed data curation is essential for advancing AI reasoning capabilities, promoting model independence and efficiency. The study highlights innovative model supervision’s role in AI development, particularly for Artificial General Intelligence (AGI).
If you want to evolve your company with AI and stay competitive, consider leveraging the Progressive Learning Framework for Enhancing AI Reasoning through Weak-to-Strong Supervision. It can redefine your work processes, automate key customer interactions, and redefine sales processes and customer engagement.
For AI KPI management advice and continuous insights into leveraging AI, connect with us at hello@itinai.com or stay tuned on our Telegram t.me/itinainews or Twitter @itinaicom.