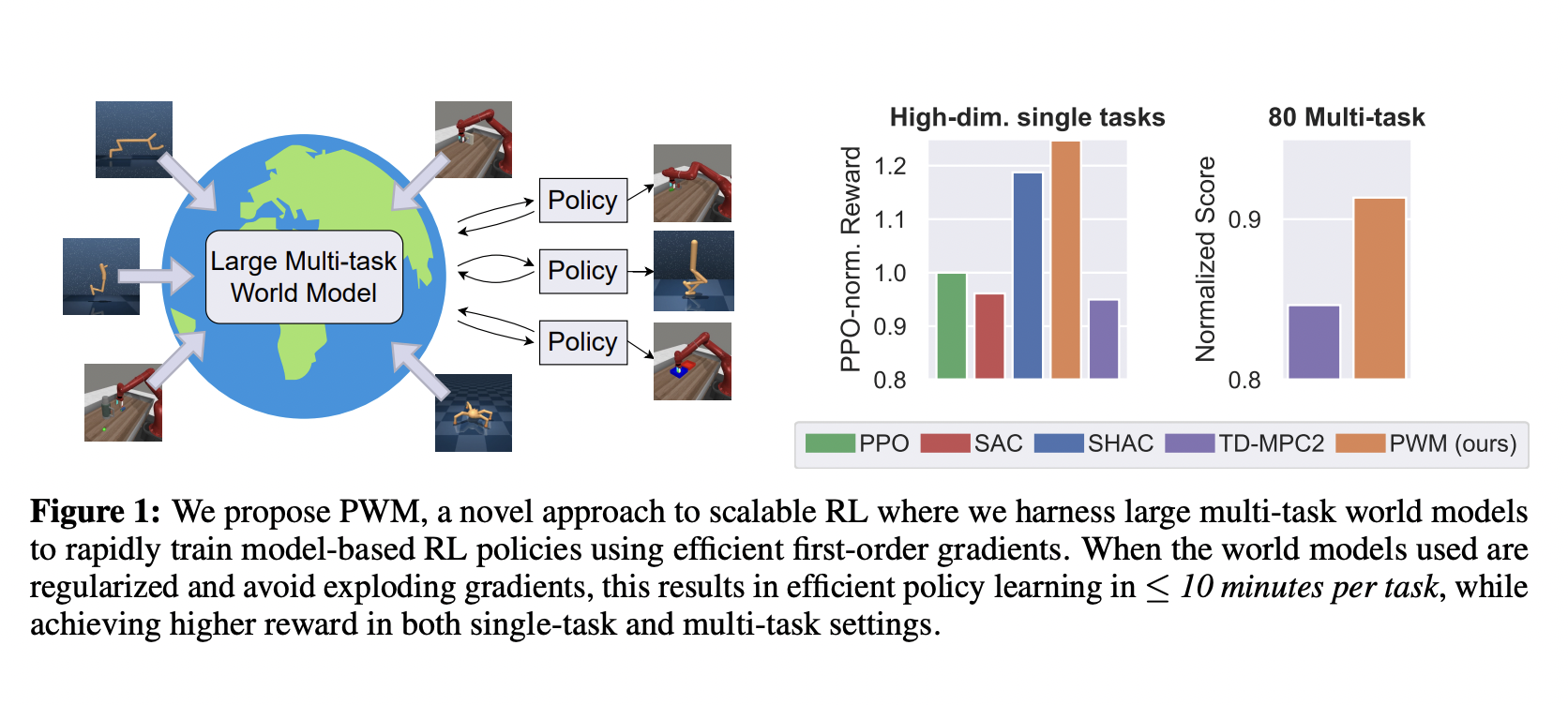
Advancing Multi-Task Reinforcement Learning Efficiency and Performance
Practical Solutions and Value
Model-Based Reinforcement Learning (MBRL) Innovation
– Policy Learning with Large World Models (PWM) offers scalable solutions for multitasking in robotics.
– Pretrains world models on offline data for efficient first-order gradient policy learning, achieving up to 27% higher rewards without costly online planning.
– Focus on smooth, stable gradients over long horizons for better policies and faster training.
Model-Free and Model-Based Approaches
– Model-free methods like PPO and SAC dominate real-world applications and employ actor-critic architectures.
– MBRL methods like DreamerV3 and TD-MPC2 leverage large world models for efficient policy training.
Evaluating PWM Performance
– PWM outperforms existing methods, achieving higher rewards and smoother optimization landscapes in complex environments.
– Superior reward performance and faster inference time than model-free methods in multi-task environments.
– Robustness to stiff contact models and higher sample efficiency highlights PWM’s strengths.
Application and Future Research
– PWM utilizes large multi-task world models for efficient policy training but relies on extensive pre-existing data for world model training.
– Challenges include re-training for each new task and limitations in low-data scenarios.
– Future research could explore enhancements in world model training and extending PWM to image-based environments and real-world applications.
For more insights on AI and how it can redefine your processes, visit our website.
For AI KPI management advice, connect with us at hello@itinai.com. For continuous insights into leveraging AI, follow us on Telegram or Twitter.