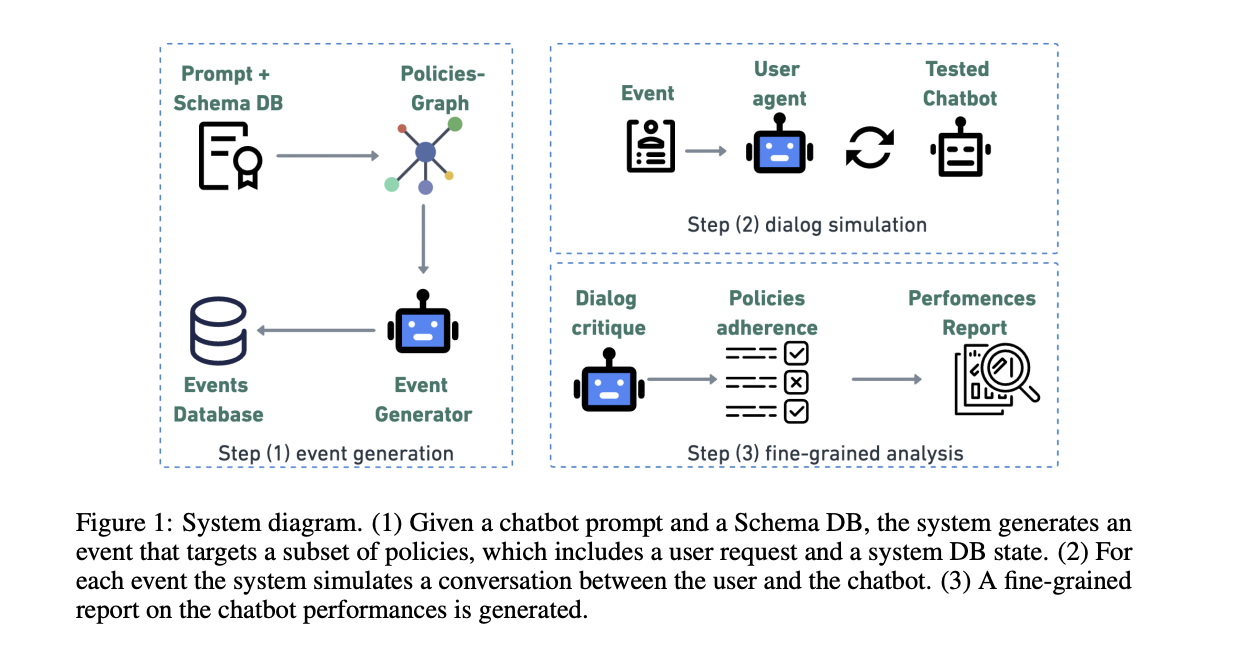
Evaluating Conversational AI Systems
Evaluating conversational AI systems that use large language models (LLMs) is a significant challenge. These systems need to manage ongoing dialogues, use specific tools, and follow complex rules. Traditional evaluation methods often fall short in these areas.
Current Evaluation Limitations
Existing benchmarks, like τ-bench and ALMITA, focus on narrow areas such as customer support and rely on small, static datasets. For instance, τ-bench assesses airline and retail chatbots but only uses 50-115 manually created examples per area. These benchmarks often miss important details like policy violations and the flow of conversation, making them inadequate for high-stakes environments like healthcare and finance.
Introducing IntellAgent
To overcome these challenges, Plurai researchers have developed IntellAgent, an open-source framework that automates the creation of diverse, policy-driven scenarios. IntellAgent uses advanced techniques like graph-based policy modeling and interactive simulations for comprehensive agent evaluation.
How IntellAgent Works
IntellAgent uses a policy graph to represent the relationships between different rules. Each node in the graph represents a specific policy, while edges show how policies might interact in a conversation. This allows IntellAgent to generate realistic user requests and database states through a weighted random walk.
Simulating Dialogues
After generating events, IntellAgent simulates conversations between a user agent and the chatbot. The user agent checks if the chatbot follows the rules. If a rule is broken, the interaction stops, and a critique component analyzes the conversation to identify policy violations. This provides detailed diagnostics, highlighting specific weaknesses in the chatbot’s performance.
Validation and Insights
Researchers validated IntellAgent by comparing its results with τ-bench using advanced LLMs like GPT-4o and Claude-3.5. Despite being fully automated, IntellAgent showed strong correlations with τ-bench results. It also revealed critical insights, such as all models struggling with user consent policies as complexity increased.
Benefits of IntellAgent
IntellAgent offers a dynamic and scalable approach to evaluating conversational AI. Its automated event generation and detailed critiques help identify areas for improvement. The framework is modular, allowing easy integration of new domains and policies.
Conclusion
IntellAgent addresses key issues in conversational AI evaluation by replacing outdated methods with a more effective, automated system. Future improvements could include using real user interactions to enhance its capabilities.
Get Involved
Check out the Paper and GitHub Page. Follow us on Twitter, join our Telegram Channel, and connect with our LinkedIn Group. Join our 70k+ ML SubReddit for more discussions.
Transform Your Business with AI
Stay competitive by leveraging AI solutions like IntellAgent. Here are some steps to get started:
- Identify Automation Opportunities: Find key customer interactions that can benefit from AI.
- Define KPIs: Ensure your AI initiatives have measurable impacts.
- Select an AI Solution: Choose tools that fit your needs and allow customization.
- Implement Gradually: Start with a pilot project, gather data, and expand carefully.
For AI KPI management advice, contact us at hello@itinai.com. For ongoing insights, follow us on Telegram or Twitter.
Explore AI Solutions
Discover how AI can enhance your sales processes and customer engagement at itinai.com.