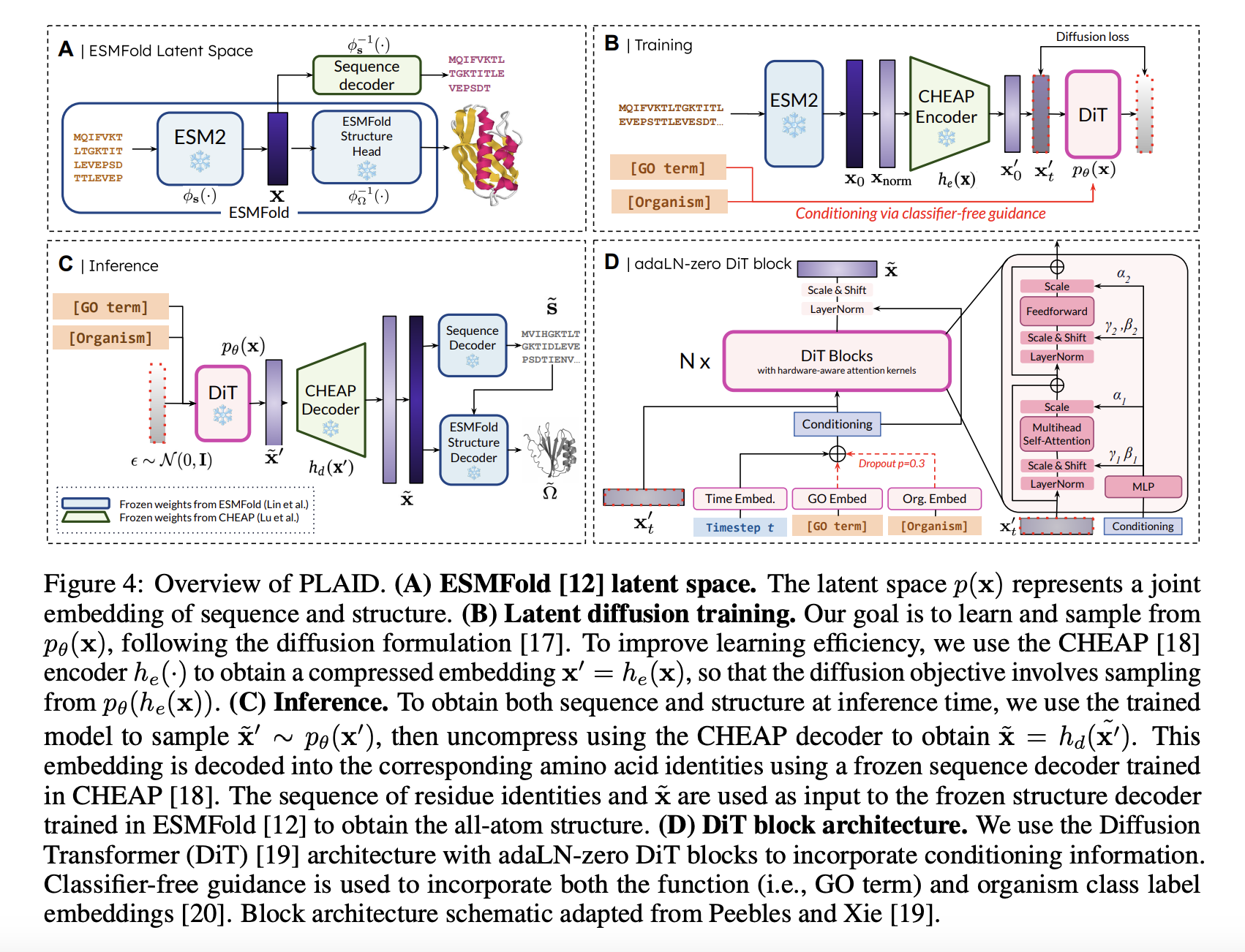
Introduction to Protein Structure Design
Designing precise all-atom protein structures is essential in bioengineering. It combines generating 3D structural information and 1D sequence data to determine the positions of side-chain atoms. Current methods often depend on limited experimental datasets, restricting our ability to explore the full variety of natural proteins. Moreover, these methods typically separate the processes of sequence and structure generation, leading to inefficiencies.
Challenges in Conventional Protein Design
Traditional protein design approaches rely on predicting backbone structures or deducing sequences from known structures. These methods treat sequence and structure as separate tasks, complicating their integration. The reliance on experimental datasets limits the ability to work with non-crystallizable proteins and slows down the process, increasing the risk of errors. Additionally, existing tools struggle to design large-scale, function-specific proteins due to their rigid structures and dataset limitations.
Introducing PLAID: A Breakthrough Solution
Researchers from UC Berkeley, Genentech, Microsoft Research, and New York University have developed a new framework called PLAID (Protein Latent Induced Diffusion). This approach synthesizes both protein sequences and all-atom structures by utilizing latent diffusion, significantly enhancing the integration of different data types.
Key Advantages of PLAID
- Enhanced Data Distribution: Increases the dataset size by 2–4 times, capturing natural protein diversity more effectively.
- Scalable Architecture: Designed for hardware efficiency, allowing for conditional multimodal protein generation.
- High Structural Fidelity: Produces samples with excellent structural quality and biophysical relevance.
How PLAID Works
PLAID maps protein sequences to a compact latent space, allowing for efficient generation and diffusion. It leverages pre-trained ESMFold weights, utilizing the extensive annotated sequence data available. The process systematically enhances features while ensuring the quality and relevance of generated proteins.
Performance Metrics
PLAID measures consistency across different data types and adherence to natural protein characteristics. This ensures that the proteins designed resemble real biological counterparts and meet design specifications.
Impact on Protein Design
This innovative framework has made significant progress in creating proteins that are not only accurate but also biologically relevant. PLAID improves the alignment between generated sequences and structures, yielding proteins with robust structural fidelity and functional specificity.
Broader Applications
PLAID’s approach is poised to enhance advancements in molecular engineering, drug development, and synthetic biology. By addressing key challenges in protein design, PLAID opens new avenues for tackling complex biological problems.
Get Involved!
Explore the full research paper and visit our GitHub page for more insights. Stay connected with us on Twitter, join our Telegram channel, and participate in our LinkedIn group. Don’t forget to join our thriving ML SubReddit community!
Leverage AI for Your Business
If you want to enhance your company with AI, consider adopting PLAID’s approach. Here’s how:
- Identify Automation Opportunities: Find areas in customer interactions that could benefit from AI.
- Define KPIs: Ensure measurable impacts from AI initiatives.
- Select AI Solutions: Choose tools that fit your needs and can be customized.
- Implement Gradually: Begin with a pilot project, analyze data, and expand thoughtfully.
Contact Us
For AI KPI management advice, reach out to us at hello@itinai.com. For ongoing insights on leveraging AI, follow us on Telegram or Twitter @itinaicom.
Transforming Sales and Engagement
Discover how AI can revolutionize your sales processes and customer engagement by exploring solutions at itinai.com.