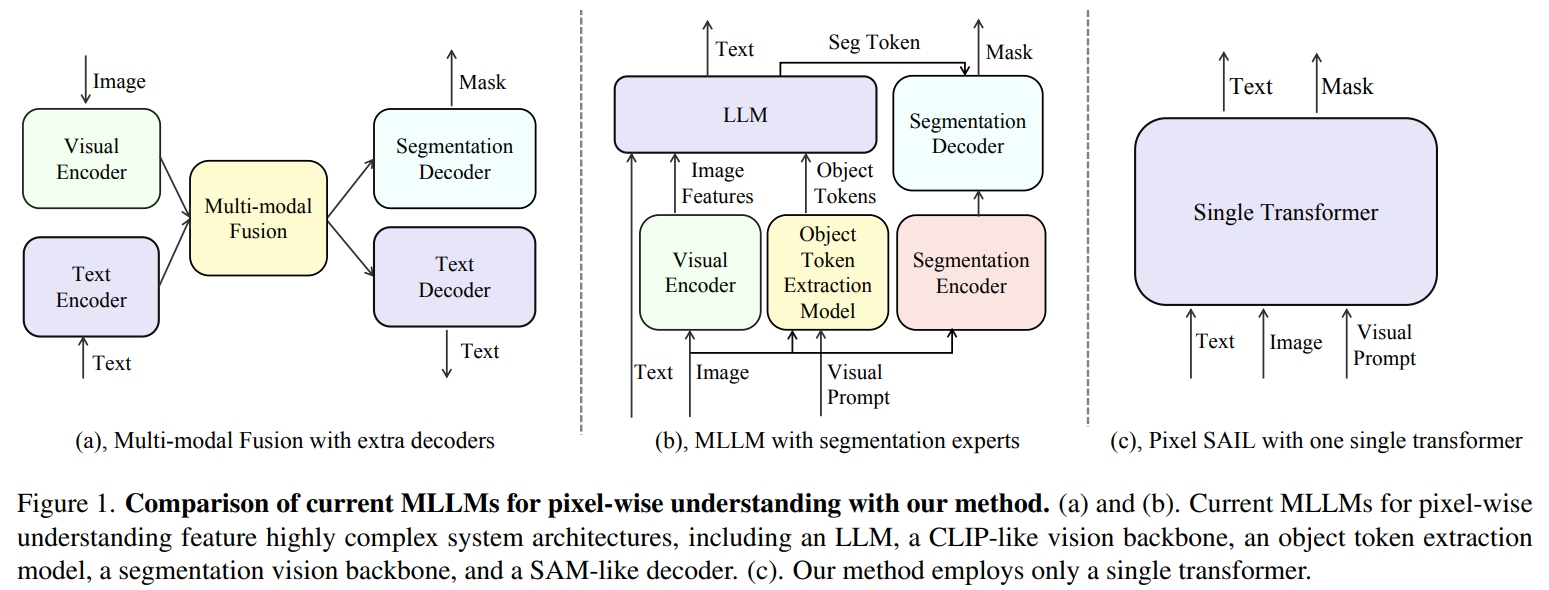
The Future of Vision-Language Models: A Professional Overview
Introduction to Pixel-SAIL
Recent advancements in Artificial Intelligence (AI) have led to the development of Pixel-SAIL, a cutting-edge model introduced by researchers from ByteDance and WHU. This innovative single-transformer model is designed to enhance pixel-level understanding, effectively outperforming larger multimodal language models (MLLMs) with a simpler architecture.
The Evolution of Vision-Language Models
Historically, vision-language models have transitioned from complex systems relying on multiple components, such as vision encoders and segmentation networks, to more unified approaches. Traditional methods like CLIP and ALIGN have necessitated intricate engineering and depend on the performance of separate modules, which can complicate scalability and adaptability.
Challenges with Modular Systems
The reliance on modular architectures often leads to inefficiencies, particularly when adapting to new tasks. For example, large-scale models that mix visual and language features face challenges in maintaining performance across various applications. Recent research has indicated a shift towards encoder-free designs, which facilitate more efficient training and inference.
Introducing Pixel-SAIL: Key Innovations
Pixel-SAIL emerges as a solution to the complexities of modular systems, with three significant innovations:
- Learnable Upsampling Module: This enhancement refines visual features for improved detail recovery.
- Visual Prompt Injection: A technique that integrates visual prompts directly into text tokens for better interaction.
- Vision Expert Distillation: This method improves mask quality by leveraging expertise from advanced models.
Performance and Benchmarking
In extensive evaluations, Pixel-SAIL outperformed larger models such as GLaMM and OMG-LLaVA across five benchmarks, including the newly proposed PerBench, which assesses tasks like referring segmentation and visual prompt understanding.
Case Studies and Results
Tests using the modified SOLO and EVEv2 architectures confirmed Pixel-SAIL’s superior segmentation capabilities with higher scores on datasets like RefCOCO and gRefCOCO. Furthermore, scaling the model size from 0.5 billion to 3 billion parameters yielded notable performance enhancements.
Practical Business Applications
Organizations can leverage Pixel-SAIL’s capabilities in various sectors:
- Customer Interactions: Automate routine inquiries and enhance service quality using AI-driven visual prompts.
- Data Analysis: Use advanced segmentation models to gain deeper insights from visual data.
- Product Development: Accelerate the design process through automated visual manipulation and editing.
Conclusion
In summary, Pixel-SAIL represents a significant advancement in the field of vision-language models by simplifying architecture while maintaining robust performance. Its innovations in upsampling, prompt injection, and expert distillation mark a new era in pixel-grounded tasks. By adopting such technologies, businesses can streamline their operations and enhance their AI strategies.
For more insights on how AI can transform your business, explore potential automation opportunities and identify key performance indicators to evaluate your AI investments. Start small, measure effectiveness, and scale your AI initiatives efficiently.
For guidance on managing AI in business, contact us at hello@itinai.ru. Connect with us on Telegram, X, and LinkedIn.