Determinism is a philosophical theory about the nature of the universe, suggesting that there is no randomness and that every event has a set of causes. This idea of determinism is relevant to various aspects of data science, including probability theory, irreducible error in machine learning models, the concept of a “god” model, causality and experiments, and the use of random numbers. Understanding determinism can provide deeper insights into the field of data science.
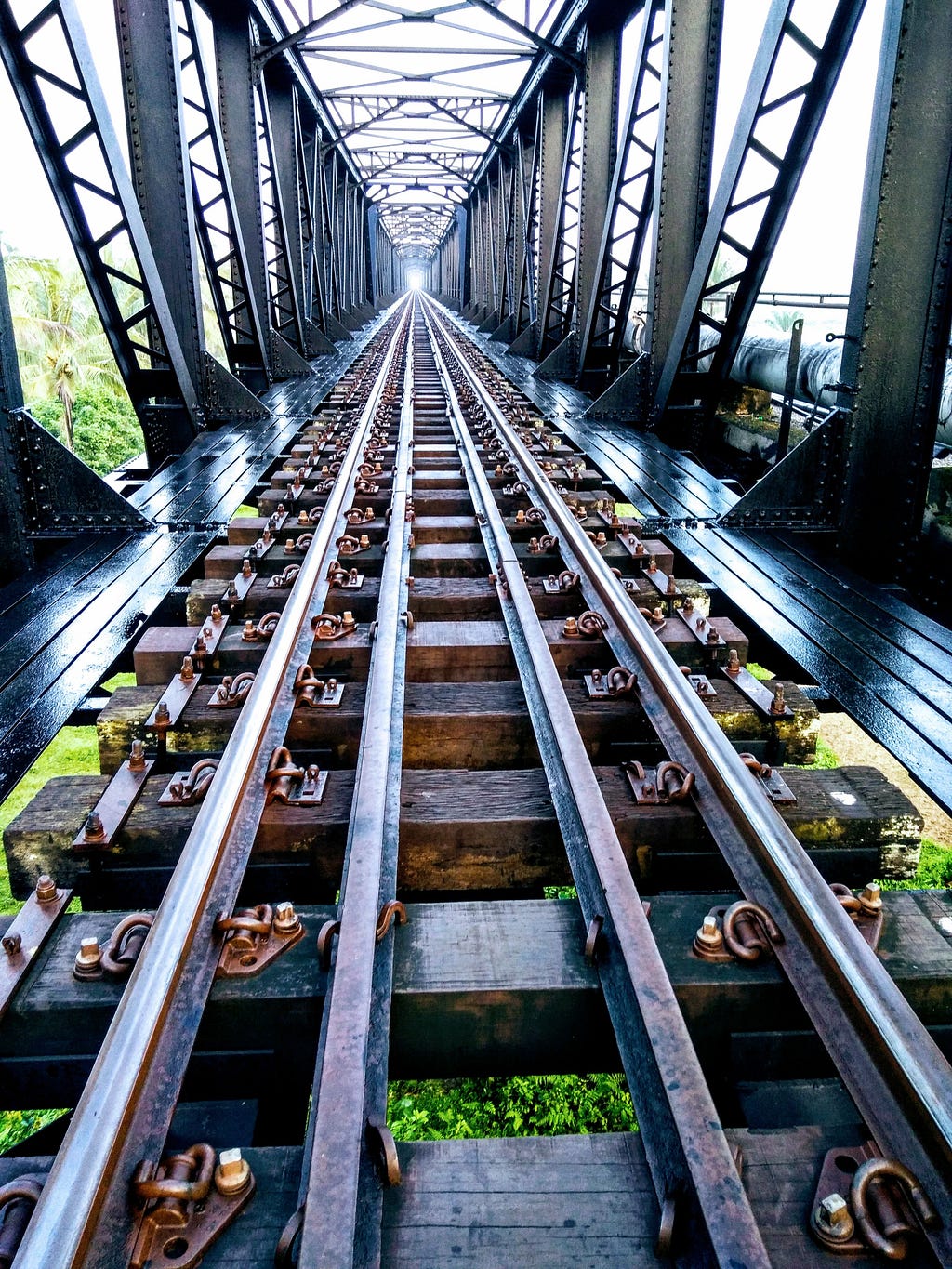
Determinism and its Implications for Data Science
Data science is a complex field that often requires deep technical knowledge. However, it can be valuable to step back and consider the bigger picture. Philosophy, particularly the study of determinism, can provide insights that are applicable to data science.
What is determinism?
Determinism is a philosophical theory that suggests there is no randomness in the universe. Every event has a set of causes that can explain it, and these causes themselves have their own causes. This chain of causes extends back to the beginning of the universe.
For example, the concept of probability theory, which is fundamental to data science, is based on the idea of randomness. However, determinism challenges this notion by suggesting that the apparent randomness is due to our limited understanding of the causes behind events.
Implications for data science
Determinism has practical implications for various aspects of data science:
- Probability theory: While probability theory deals with random variables, determinism suggests that randomness is an illusion caused by our limited knowledge. Probability remains a useful field of study, but it acknowledges the boundaries of our understanding.
- Model error: Machine learning models are imperfect and have errors. Determinism suggests that there are different sources of error, including model approximation, unavailable data, and random noise. Understanding these sources can help improve model accuracy.
- ‘God’ model: In a deterministic universe, a perfect model that accurately predicts outcomes would be possible. However, our current limitations prevent us from achieving this level of accuracy.
- Causality and experiments: Determinism assumes that everything is causally connected. This has implications for experimental design and the interpretation of results. It challenges the idea of randomness in experiments and suggests that variations can be attributed to our limited understanding.
- Random numbers: Determinism suggests that true randomness does not exist. Random number generators used in data science are actually pseudorandom numbers generated by deterministic algorithms.
Practical AI solutions for middle managers
If you want to leverage AI to evolve your company and stay competitive, consider the following steps:
- Identify Automation Opportunities: Locate key customer interaction points that can benefit from AI.
- Define KPIs: Ensure your AI endeavors have measurable impacts on business outcomes.
- Select an AI Solution: Choose tools that align with your needs and provide customization.
- Implement Gradually: Start with a pilot, gather data, and expand AI usage judiciously.
For AI KPI management advice and insights into leveraging AI, connect with us at hello@itinai.com or follow us on Telegram or Twitter.
Spotlight on a Practical AI Solution: AI Sales Bot
Consider using the AI Sales Bot from itinai.com/aisalesbot to automate customer engagement and manage interactions across all stages of the customer journey. This AI solution can redefine your sales processes and improve customer engagement.