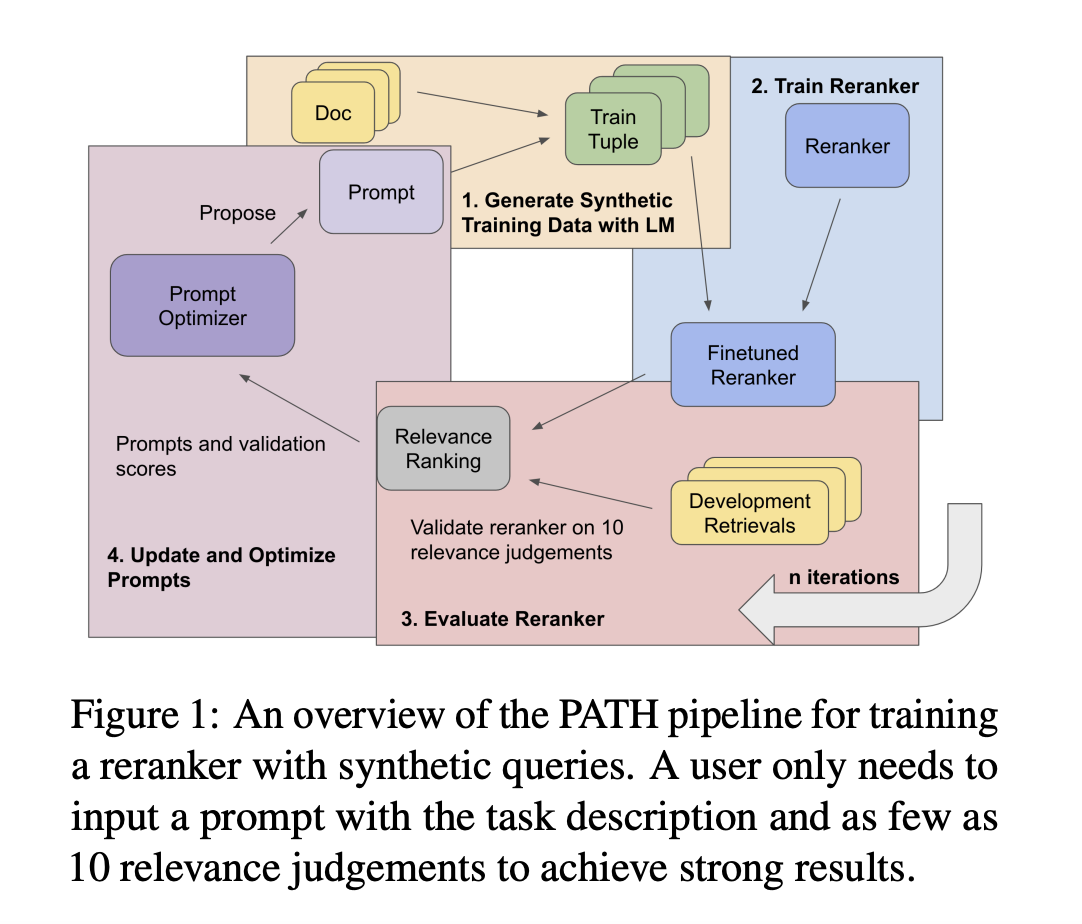
The Value of PATH: A Machine Learning Method for Training Small-Scale Neural Information Retrieval Models
Improving Information Retrieval Quality
The use of pretrained language models has significantly improved the quality of information retrieval (IR) by training models on large datasets. However, the necessity of such large-scale data for language model optimization has been questioned, leading to scientific and engineering challenges.
Practical Solution
A team of researchers has introduced PATH, a technique for training small-scale neural information retrieval models using minimal labeled data and fewer parameters. This approach, named Prompts as Auto-optimized Training Hyperparameters, leverages a language model to create fictitious document queries and automatically optimize the prompt for training data creation.
Performance Improvement
Trials using the BIRCO benchmark have shown that PATH greatly enhances the performance of trained models, outperforming larger models trained with more labeled data. This demonstrates the effectiveness of automatic rapid optimization in producing high-quality artificial datasets and the potential for smaller models to outperform larger ones with the right adjustments to the data creation process.
AI Integration and Evolution
Companies can leverage PATH to evolve with AI, stay competitive, and redefine their work processes. By identifying automation opportunities, defining KPIs, selecting suitable AI solutions, and implementing AI gradually, organizations can benefit from AI-driven improvements in customer interaction and sales processes.
Connect with Us
For AI KPI management advice and continuous insights into leveraging AI, connect with us at hello@itinai.com. Explore AI solutions for sales processes and customer engagement at itinai.com.