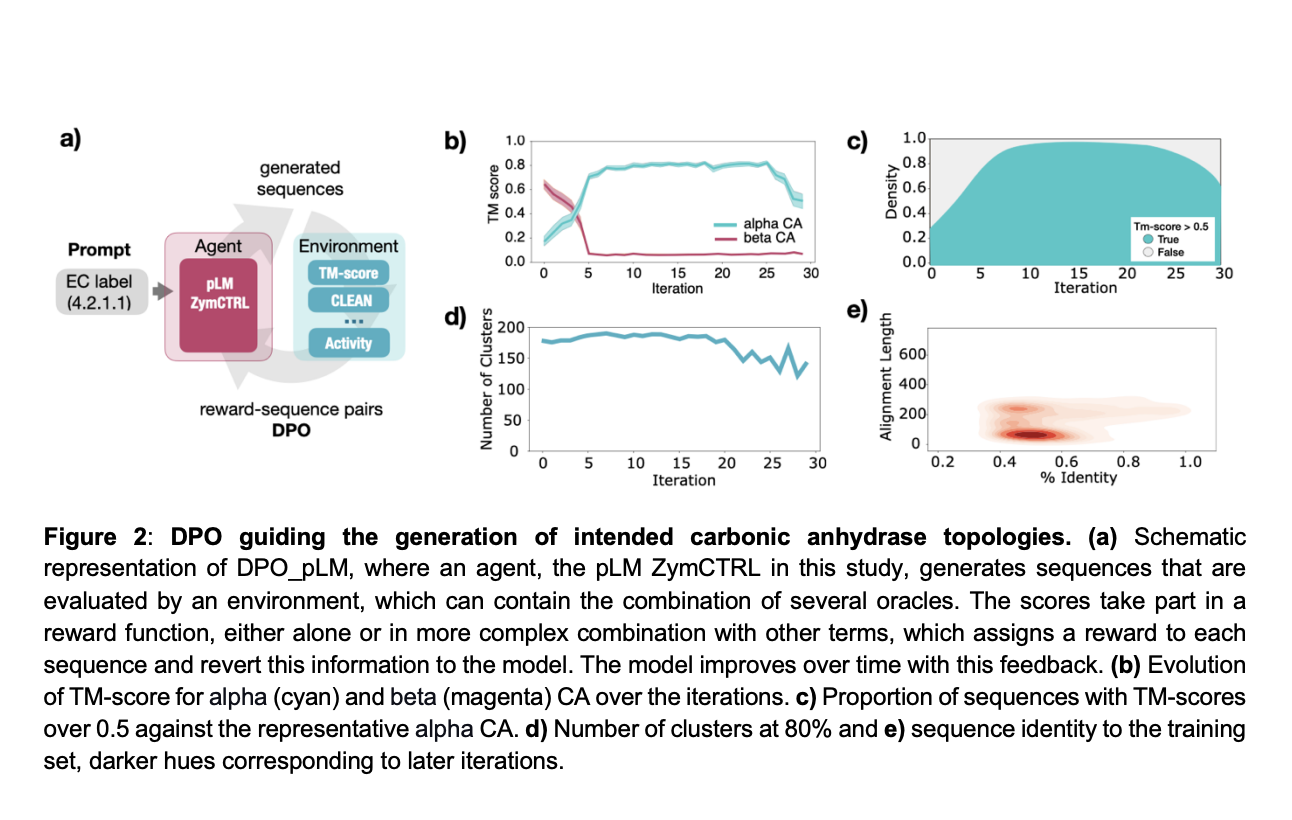
Revolutionizing Protein Design with AI Solutions
Transformative Tools in Protein Engineering
Autoregressive protein language models (pLMs) are changing how we design functional proteins. They can create diverse enzyme families, such as lysozymes and carbonic anhydrases, by analyzing patterns in training data. However, pLMs face challenges in targeting rare, valuable protein sequences, making tasks like engineering enzymatic activities difficult.
Addressing Optimization Challenges
Protein optimization is complicated due to the vast sequence space and costly lab validations. Traditional methods, like directed evolution, only explore local areas and lack long-term direction. This is where Reinforcement Learning (RL) comes in.
Introducing Reinforcement Learning for Protein Design
RL provides a powerful way to guide pLMs in optimizing specific protein properties. By receiving feedback from external sources, such as predictions on stability or binding affinities, pLMs can explore rare events more effectively. Techniques like Proximal Policy Optimization (PPO) and Direct Preference Optimization (DPO) show promising results in protein design.
Meet DPO_pLM: A New RL Framework
Researchers from top institutions have developed DPO_pLM, an innovative RL framework that fine-tunes pLMs using external rewards. This method optimizes various user-defined properties while keeping protein sequences diverse. DPO_pLM reduces computational needs and improves performance significantly, successfully designing high-affinity EGFR binders in just hours.
Key Features of DPO and Self-Fine-Tuning
DPO minimizes loss functions effectively, while self-fine-tuning (s-FT) refines protein sequences through multiple iterations. By utilizing Hugging Faceβs transformers API, the model efficiently evaluates and optimizes protein designs.
High Functionality with Diversity
pLMs can produce sequences similar to their training data, achieving high functionality despite variations. For example, ZymCTRL generated carbonic anhydrases with significant activity from sequences that only matched 39% of the original data. However, precise optimization for specific traits remains a challenge. With RL and DPO, fine-tuning can target desired properties while maintaining diversity.
Conclusion: The Future of Protein Optimization
DPO_pLM enhances the capabilities of pLMs, allowing for efficient, targeted sequence generation. With proven success in rapid design iterations, this framework is set to transform protein engineering. Future efforts will focus on integrating DPO_pLM into automated labs for innovative designs.
Get Involved and Learn More
For more details, check out the research paper. Follow us on Twitter, join our Telegram Channel, and be part of our LinkedIn Group. Join our community of over 60k on ML SubReddit!
Elevate Your Business with AI
Stay competitive by leveraging AI in protein design and beyond. Hereβs how:
- Identify Automation Opportunities: Find key areas for AI integration.
- Define KPIs: Measure the impact of AI on your business.
- Select an AI Solution: Choose tools that fit your needs.
- Implement Gradually: Start small, gather insights, and expand.
For AI KPI management advice, contact us at hello@itinai.com. Stay updated on AI insights on our Telegram t.me/itinainews or Twitter @itinaicom.
Discover how AI can transform your sales processes and customer engagement at itinai.com.