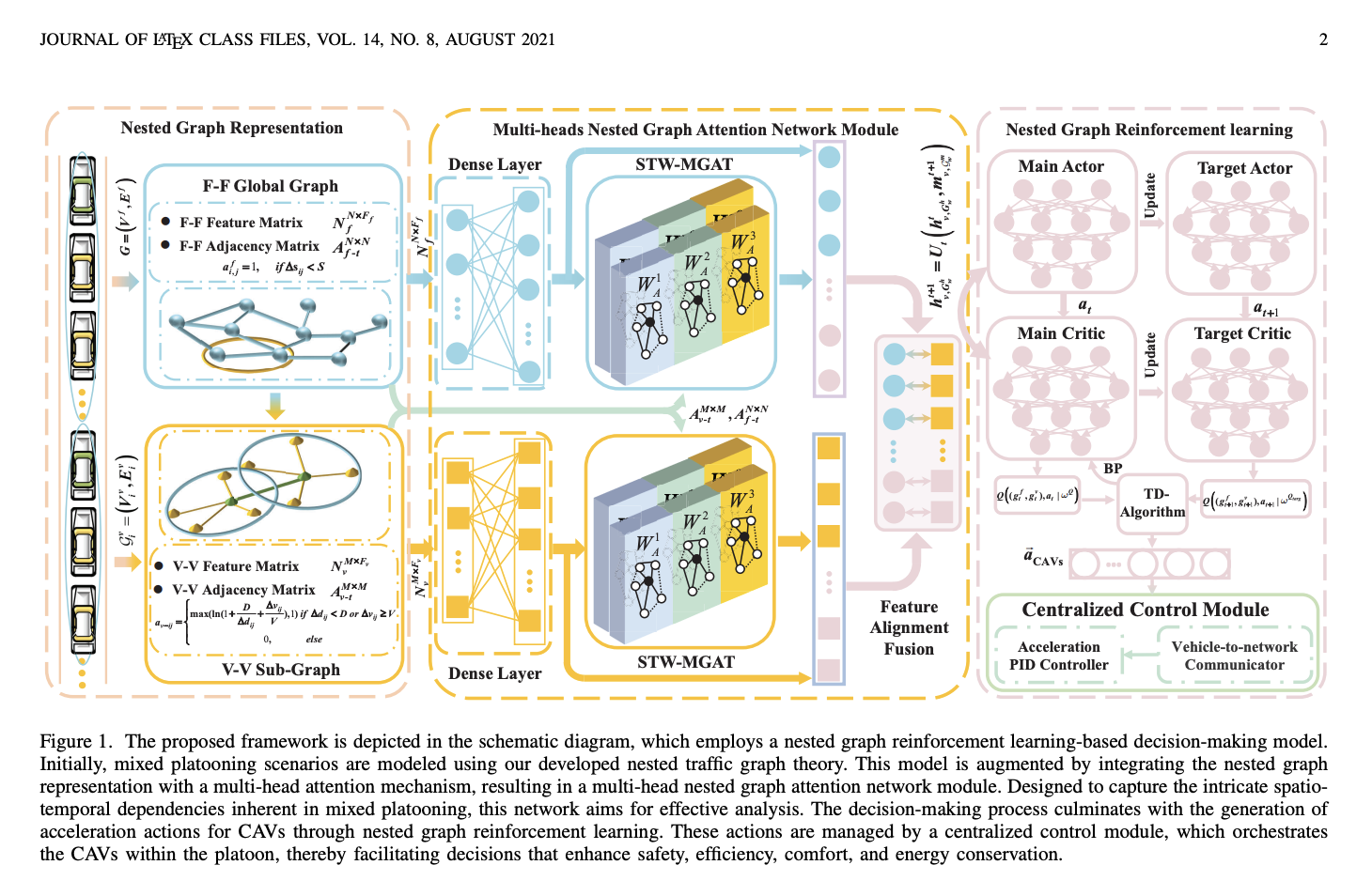
Practical Solutions for Optimizing Large-Scale Mixed Platoons
Addressing Traffic Flow Challenges
The platooning technology can optimize traffic flow, increase energy economy, and expand road capacity. However, issues arise in large-scale mixed platoons due to vehicle heterogeneity, leading to virtual bottlenecks in traffic flow.
Decision-Making Framework
A unique decision-making approach based on stacked graph reinforcement learning has been introduced to improve cooperative decision-making within the platoon. This method accurately reflects real-world traffic circumstances and improves the model’s capacity to handle local and global data, resulting in more efficient and stable platoon operations.
Enhanced Efficiency and Throughput
The nested graph reinforcement learning framework has demonstrated significant improvements in energy usage and traffic throughput. It has also shown the capacity for self-iterative learning, enabling the system to make better decisions over time and operate more effectively in dynamic traffic situations.
Validation and Results
Extensive simulation experiments have validated the framework, showing enhanced energy efficiency, traffic flow, and congestion management in large-scale mixed platoons. The approach has resulted in a 9% reduction in energy usage and a 10% increase in traffic throughput compared to baseline methods.
Future Implications
Nested graph reinforcement learning is a significant advancement in addressing the challenges of large-scale mixed platooning, leading to increased efficiency and sustainability in transportation systems.
AI Solutions for Business Evolution
Utilizing AI for Competitive Advantage
Discover how AI can redefine your company’s way of work and identify automation opportunities to stay competitive.
Implementing AI in Sales Processes
Explore AI solutions to redefine sales processes and customer engagement, and connect with us for AI KPI management advice and continuous insights into leveraging AI.