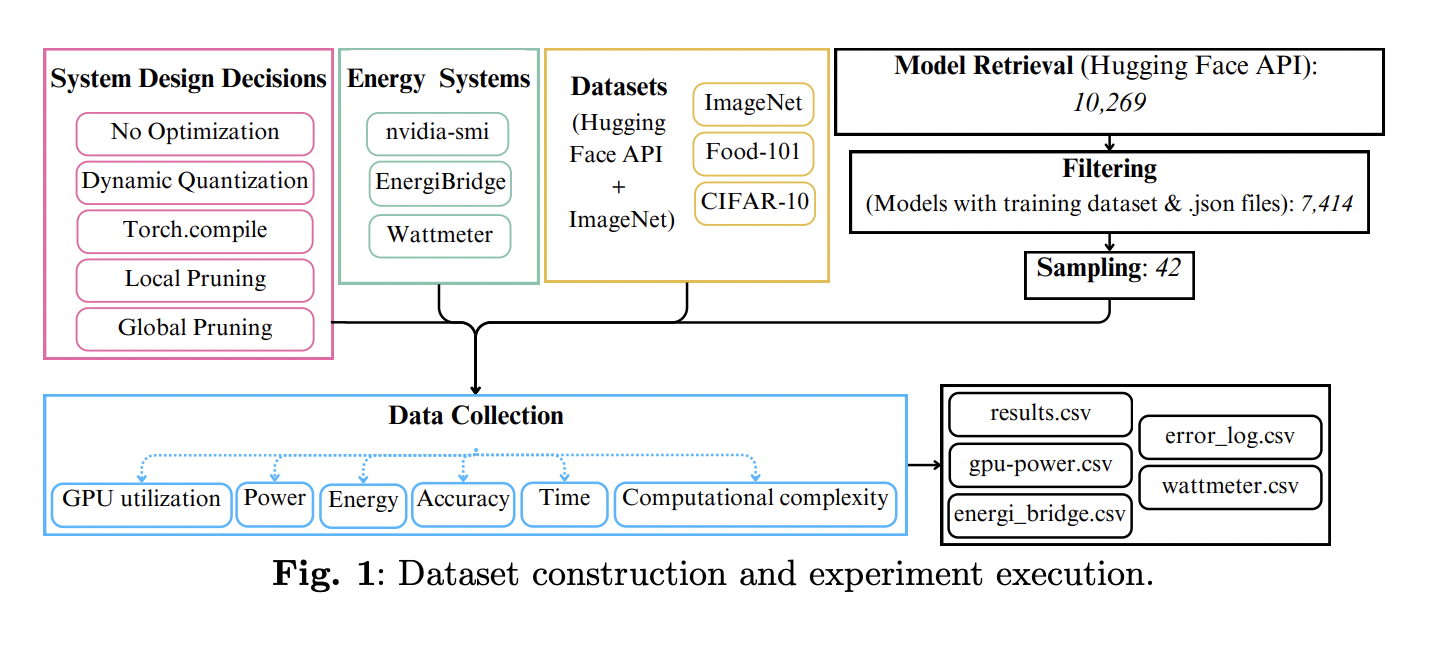
Practical Solutions for Optimizing Energy Efficiency in Machine Learning
Overview
With technology advancing rapidly, it is crucial to focus on the energy impact of Machine Learning (ML) projects. Green software engineering addresses the issue of energy consumption in ML by optimizing models for efficiency.
Research Findings
– Dynamic quantization in PyTorch reduces energy use and inference time.
– Torch. compile balances accuracy and energy efficiency.
– Local pruning does not improve efficiency, while global pruning increases costs.
– Techniques like pruning, quantization, and knowledge distillation aim to reduce resource consumption.
Key Metrics
– Inference time, accuracy, and economic costs are analyzed using the Green Software Measurement Model (GSMM).
– Optimization techniques impact GPU utilization, power consumption, and computational complexity.
– Results guide efficient ML model development.
Recommendations
– ML engineers can use a decision tree to select techniques based on priorities.
– Better documentation of model details is recommended for reliability.
– Implement pruning techniques that enhance efficiency.
– Future work includes NLP models, multimodal applications, and TensorFlow optimizations.
AI Implementation Tips
– Identify automation opportunities for AI integration.
– Define measurable KPIs for AI projects.
– Select AI solutions aligned with your needs.
– Implement AI gradually, starting with a pilot.
Contact Us
For AI KPI management advice, email us at hello@itinai.com. Stay updated on AI insights via Telegram t.me/itinainews or Twitter @itinaicom.
Discover More
Explore how AI can redefine your sales processes and customer engagement at itinai.com.