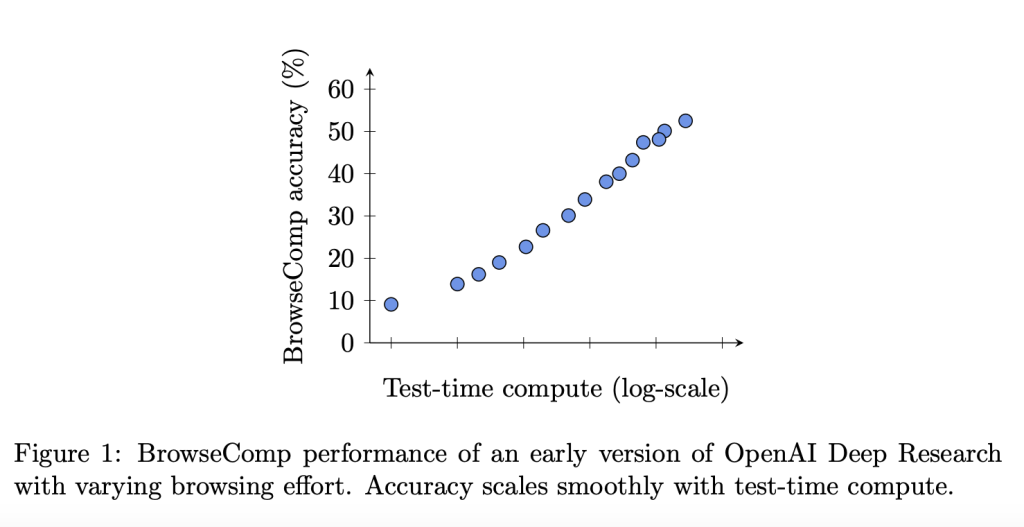
OpenAI’s BrowseComp: Enhancing AI Web Browsing Capabilities
Introduction
Despite significant advancements in large language models (LLMs), AI agents still struggle with complex web browsing tasks. Traditional benchmarks often evaluate models based on their ability to recall easily accessible information, which does not accurately reflect the challenges faced in real-world scenarios. AI agents need to demonstrate persistence, structured reasoning, and adaptability to effectively retrieve nuanced information from multiple sources.
Overview of BrowseComp
OpenAI has introduced BrowseComp, a comprehensive benchmark consisting of 1,266 information-seeking tasks aimed at assessing AI agents’ web browsing capabilities. Each task requires navigating various web pages to find precise answers, emphasizing the need for effective filtering and reasoning skills.
Benchmark Design
BrowseComp employs a reverse-question design methodology, where questions are crafted to obscure straightforward answers. This approach ensures that AI agents cannot rely on superficial searches, compelling them to engage in deeper reasoning and retrieval processes. The dataset covers diverse domains, including science, history, arts, sports, and entertainment, promoting topic diversity and complexity.
Model Evaluation and Insights
OpenAI evaluated several models, including GPT-4o and Deep Research, on the BrowseComp benchmark. The findings revealed significant performance disparities:
- GPT-4o without browsing: 0.6% accuracy
- GPT-4o with browsing: 1.9% accuracy
- OpenAI o1: 9.9% accuracy
- Deep Research: 51.5% accuracy
Deep Research’s success can be attributed to its architecture, which emphasizes iterative searching and evidence synthesis. The model’s performance improved with multiple trials and aggregation strategies, showcasing the importance of adaptive navigation in complex tasks.
Human Performance and Task Complexity
Human trainers attempted to solve the benchmark tasks without AI assistance. Out of 1,255 tasks, 71% were deemed unsolvable within a two-hour timeframe, highlighting the benchmark’s complexity. Only 29% of tasks were completed successfully, with an agreement rate of 86.4% with the reference answers. These results indicate that even human experts face challenges, underscoring the need for further advancements in AI adaptability and reasoning skills.
Conclusion
BrowseComp establishes a rigorous benchmark for evaluating AI web-browsing agents, shifting the focus from static recall to dynamic retrieval and multi-hop reasoning. While current models exhibit uneven performance, the success of the Deep Research agent illustrates the potential for specialized architectures to enhance AI capabilities. This benchmark not only provides insights into current AI limitations but also paves the way for future developments in AI technology.
Practical Business Solutions
Businesses can leverage insights from BrowseComp to improve their AI strategies:
- Identify Automation Opportunities: Explore tasks that can be automated, particularly in customer interactions, to enhance efficiency.
- Establish Key Performance Indicators (KPIs): Monitor the impact of AI investments on business outcomes to ensure positive returns.
- Select Tailored Tools: Choose AI tools that can be customized to meet specific business objectives.
- Start Small and Scale: Implement small-scale AI projects, analyze their effectiveness, and gradually expand their application.
For guidance on integrating AI into your business, please contact us at hello@itinai.ru or connect with us on Telegram, X, or LinkedIn.