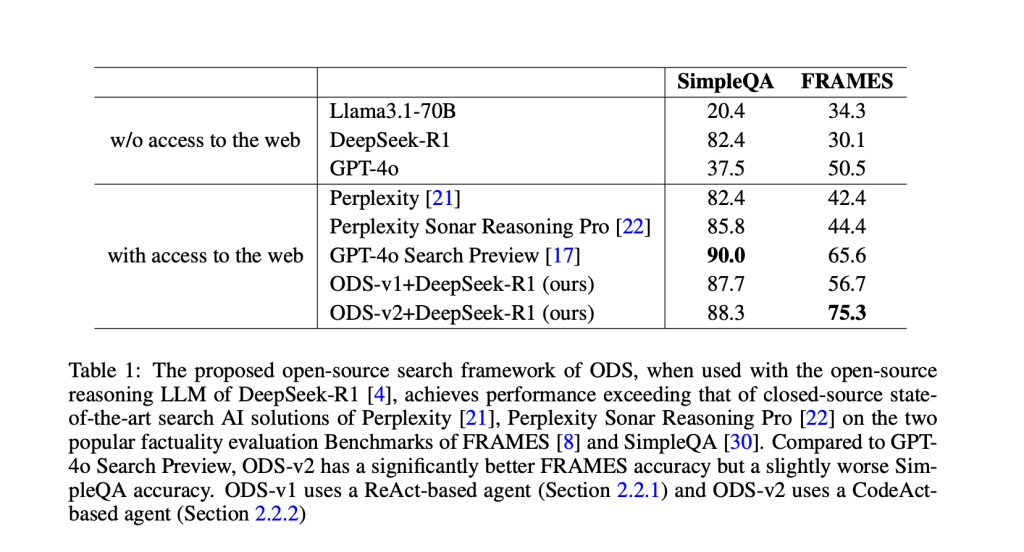
Introducing Open Deep Search (ODS): A Revolutionary Open-Source Framework for Enhanced Search
The landscape of search engine technology has evolved rapidly, primarily favoring proprietary solutions like Google and GPT-4. While these systems demonstrate strong performance, their closed-source nature raises concerns regarding transparency, innovation, and community collaboration. This exclusivity limits the potential for customization and restricts broader engagement from academic and entrepreneurial sectors in search-enhanced artificial intelligence (AI).
Understanding Open Deep Search (ODS)
In response to these challenges, a collaborative effort from researchers at the University of Washington, Princeton University, and UC Berkeley has led to the development of Open Deep Search (ODS). This open-source framework enables seamless integration with any chosen large language model (LLM) in a modular fashion. ODS consists of two key components:
- Open Search Tool: This tool features an advanced retrieval pipeline that includes intelligent query rephrasing to better capture user intent, resulting in more accurate and diverse search results. It also employs refined chunking and re-ranking techniques to filter results based on relevance.
- Open Reasoning Agent: This agent utilizes two methodologies: the Chain-of-thought ReAct agent and the Chain-of-code CodeAct agent. These agents interpret user queries, manage tool usage, and generate comprehensive, contextually accurate responses.
Performance Metrics and Case Studies
Empirical evaluations highlight the effectiveness of ODS. When integrated with DeepSeek-R1, an open-source reasoning model, ODS-v2 achieved impressive accuracy rates of 88.3% on the SimpleQA benchmark and 75.3% on the FRAMES benchmark. This performance surpasses proprietary alternatives like Perplexity and Sonar Reasoning Pro, which scored 85.8% and 44.4% respectively. Notably, ODS-v2 outperformed OpenAI’s GPT-4 on the FRAMES benchmark by 9.7% in accuracy.
Adaptive Resource Management
A standout feature of ODS is its adaptive use of tools, showcasing strategic decision-making in resource management. For straightforward queries, ODS minimizes additional searches, demonstrating efficient resource utilization. Conversely, for complex multi-hop queries, it intelligently increases web searches, reflecting a tailored approach to query complexity.
Practical Business Solutions
Organizations can leverage ODS to enhance their search capabilities through the following practical solutions:
- Automation of Processes: Identify areas where AI can automate repetitive tasks, improving efficiency and productivity.
- Enhancing Customer Interactions: Utilize AI to enrich customer engagement, ensuring timely and relevant responses.
- Tracking Key Performance Indicators (KPIs): Establish metrics to assess the impact of AI investments on business outcomes.
- Custom Tool Selection: Choose tools that align with your specific needs and allow for customization to meet your objectives.
- Gradual Implementation: Start with small AI projects, gather data on their effectiveness, and expand usage based on insights.
Conclusion
Open Deep Search represents a significant advancement in democratizing search-enhanced AI by providing an open-source framework compatible with various LLMs. It fosters innovation and transparency within the AI research community while encouraging broader participation in the development of advanced search and reasoning capabilities. By integrating sophisticated retrieval techniques with adaptive reasoning methodologies, ODS sets a robust standard for the future of search-integrated large language models.
For further inquiries or guidance on managing AI in your business, please contact us at hello@itinai.ru. You can also connect with us on Telegram, X, and LinkedIn.