Decision trees are often replaced with random forests, but this prioritizes a “black box” algorithm. Decision trees provide intuitive results and allow for trade-off comparisons and process improvement. To improve decision tree performance, principal component analysis (PCA) can be applied to optimize feature data and reduce the feature space. This improves performance and generalizability.
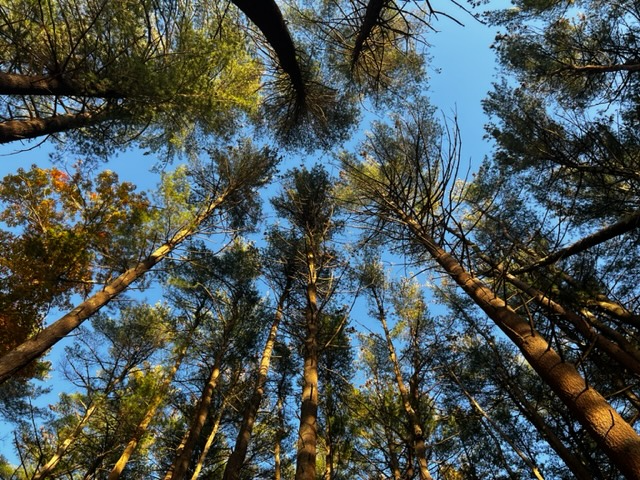
One Step to Make Decision Trees Produce Better Results
Background, implementation, and model improvement
Decision trees (DT) are often replaced with Random Forests, but this prioritizes a “black box” algorithm. Decision trees offer intuitive results, allow comparison of trade-offs, and involve business leaders in process improvement.
How Decision Trees make decisions
Under the hood, decision trees separate classes by creating orthogonal decision boundaries between classes among the data provided. Feature data with clear orthogonal decision boundaries are ideal for decision tree models.
Implementing the Process
Principal Component Analysis (PCA) can be applied to improve decision tree performance and generalizability. PCA orients key features together and reduces the feature space. This process surfaces the most predictive variables for decision tree inclusion.
Conclusion
Instead of reaching for more advanced algorithms, optimal data preparation through thoughtful feature engineering and PCA can give decision trees the best chance of revealing their intuitive decision-making capabilities.
If you want to evolve your company with AI and stay competitive, consider using “One Step to Make Decision Trees Produce Better Results” to redefine your work. Connect with us at hello@itinai.com for AI KPI management advice and explore AI solutions at itinai.com.