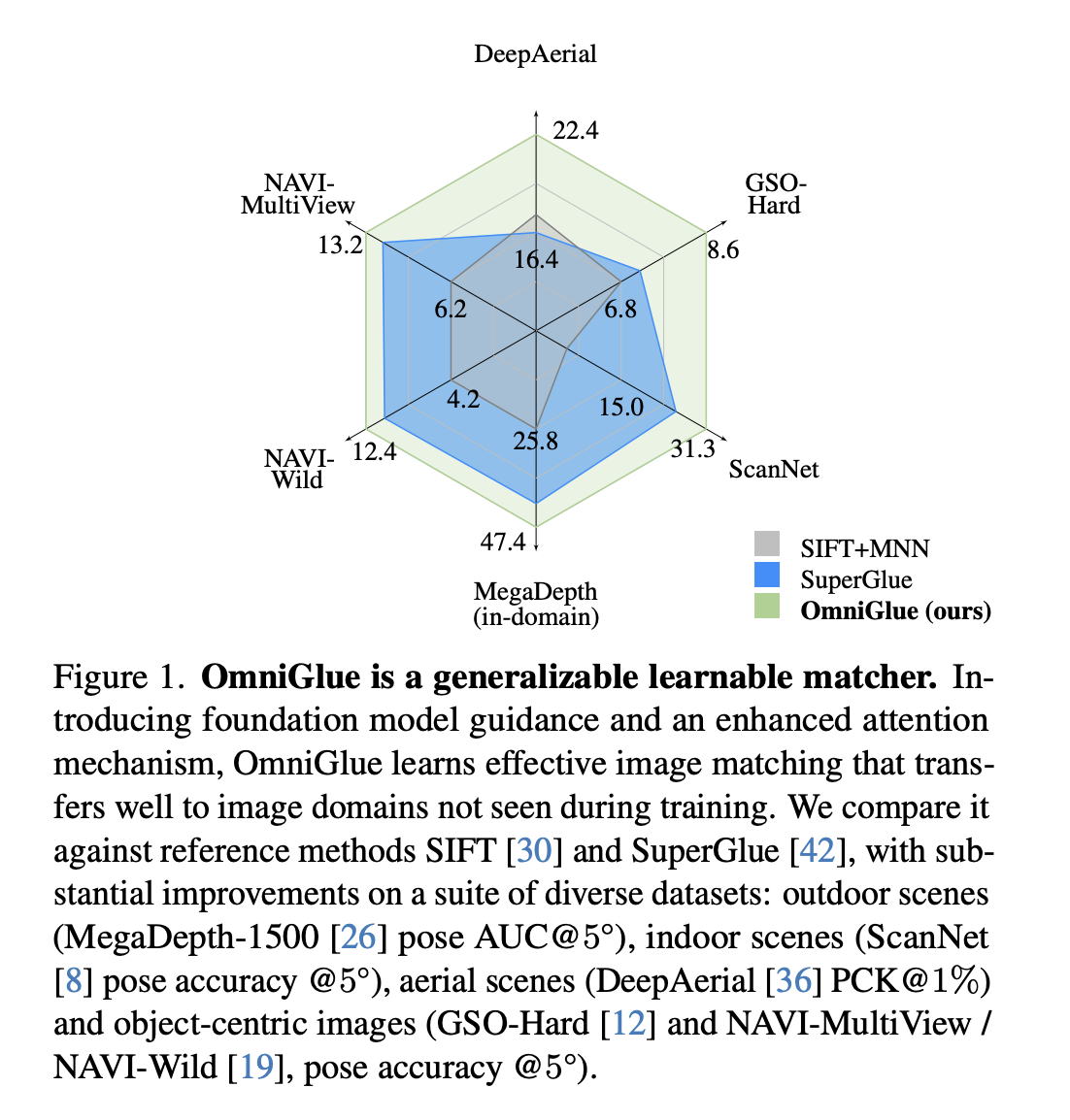
Local Image Feature Matching Techniques
Local image feature matching techniques help identify fine-grained visual similarities between two images. However, current advancements in this area often lack generalization capability, especially when dealing with out-of-domain data. The cost of collecting high-quality correspondence annotations is high, making it crucial to develop architectural improvements to generalize learnable matching methods.
OmniGlue: The First Learnable Image Matcher Designed with Generalization as a Core Principle
OmniGlue is a groundbreaking learnable image matcher designed to enhance generalization in out-of-distribution scenarios while maintaining strong performance in the source domain. It introduces techniques such as foundation model guidance and keypoint-position attention guidance to achieve better generalization without compromising performance.
Comparison and Results
Experimental comparisons with existing methods such as SIFT, SuperPoint, and SuperGlue demonstrate that OmniGlue outperforms these methods in in-domain data and exhibits superior generalization capabilities. It shows significant improvements in precision and recall, making it a promising solution for diverse image-matching tasks.
Practical AI Solutions
Identify Automation Opportunities
Locate key customer interaction points that can benefit from AI.
Define KPIs
Ensure your AI endeavors have measurable impacts on business outcomes.
Select an AI Solution
Choose tools that align with your needs and provide customization.
Implement Gradually
Start with a pilot, gather data, and expand AI usage judiciously.
For AI KPI management advice, connect with us at hello@itinai.com. Stay tuned on our Telegram channel or Twitter for continuous insights into leveraging AI.
Spotlight on a Practical AI Solution
Consider the AI Sales Bot from itinai.com/aisalesbot, designed to automate customer engagement 24/7 and manage interactions across all customer journey stages.