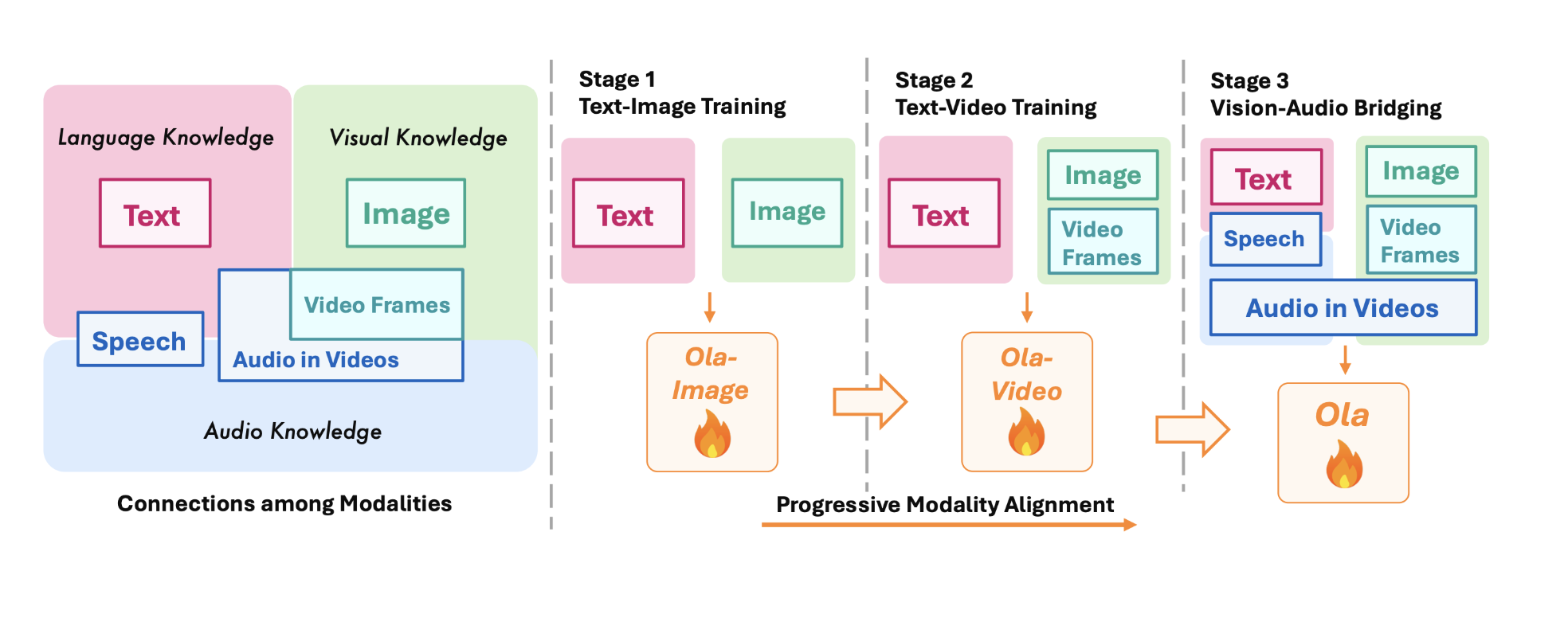
Understanding the Challenge of Omni-modal Data
Working with various types of data—like text, images, videos, and audio—within a single model is quite challenging. Current large language models often don’t perform as well when trying to handle all these types together compared to specialized models that focus on just one. This is mainly because each data type has unique patterns, making it difficult to ensure accuracy across different tasks. Many models struggle to align information from diverse inputs, leading to slow responses and requiring extensive data. These limitations hinder the development of effective models that can equally understand all data types.
Current Approaches to Data Processing
Most existing models focus on specific tasks, such as image recognition or audio processing, independently. While some models attempt to combine these tasks, their performance is still inferior to specialized ones. Vision-language models have made progress in handling videos and mixed inputs, but integrating audio effectively remains a significant challenge. Large audio-text models aim to link speech with language processing, but they still fall short in understanding complex audio like music and events. New omni-modal models are emerging, but they often face issues like poor performance and inefficient data handling.
Introducing Ola: The Omni-modal Solution
Researchers from Tsinghua University, Tencent Hunyuan Research, and S-Lab, NTU have developed Ola, an advanced omni-modal model designed to understand and generate various data types, including text, speech, images, videos, and audio. Ola uses a modular architecture where each data type has its own encoder to process information. This allows a central Large Language Model (LLM) to interpret and respond to inputs from all modalities seamlessly.
Key Features of Ola
- Dual Encoder for Audio: Ola processes speech and music features separately to enhance audio understanding.
- Efficient Vision Processing: OryxViT maintains the original aspect ratios of visual inputs to minimize distortion.
- Local-Global Attention Pooling: This feature compresses token length while keeping essential data, improving computational efficiency.
- Real-time Speech Synthesis: An external text-to-speech decoder enables quick output.
Proven Performance and Future Potential
Ola has been thoroughly evaluated against benchmarks for image, video, and audio understanding. It builds on the Qwen-2.5-7B model and integrates several specialized encoders, achieving superior results across multiple tests. For instance, Ola recorded impressive performance in audio benchmarks, surpassing previous omni-modal models and nearing specialized audio models.
By successfully combining various data types and implementing effective training methods, Ola sets a new standard for omni-modal learning. Its architecture and training techniques can serve as a foundational model for future developments in AI technology.
Leverage AI with Ola
To gain a competitive edge, consider incorporating Ola into your business processes. Here are practical steps:
- Identify Automation Opportunities: Find key customer interaction points suitable for AI enhancement.
- Define KPIs: Ensure your AI initiatives are measurable and impactful.
- Select an AI Solution: Choose customizable tools that meet your specific needs.
- Implement Gradually: Start small, gather insights, and expand AI usage wisely.
For AI KPI management advice, reach out at hello@itinai.com. Stay updated on AI trends via our Telegram or follow us on @itinaicom.
Explore how AI can revolutionize your sales processes and customer engagement at itinai.com.