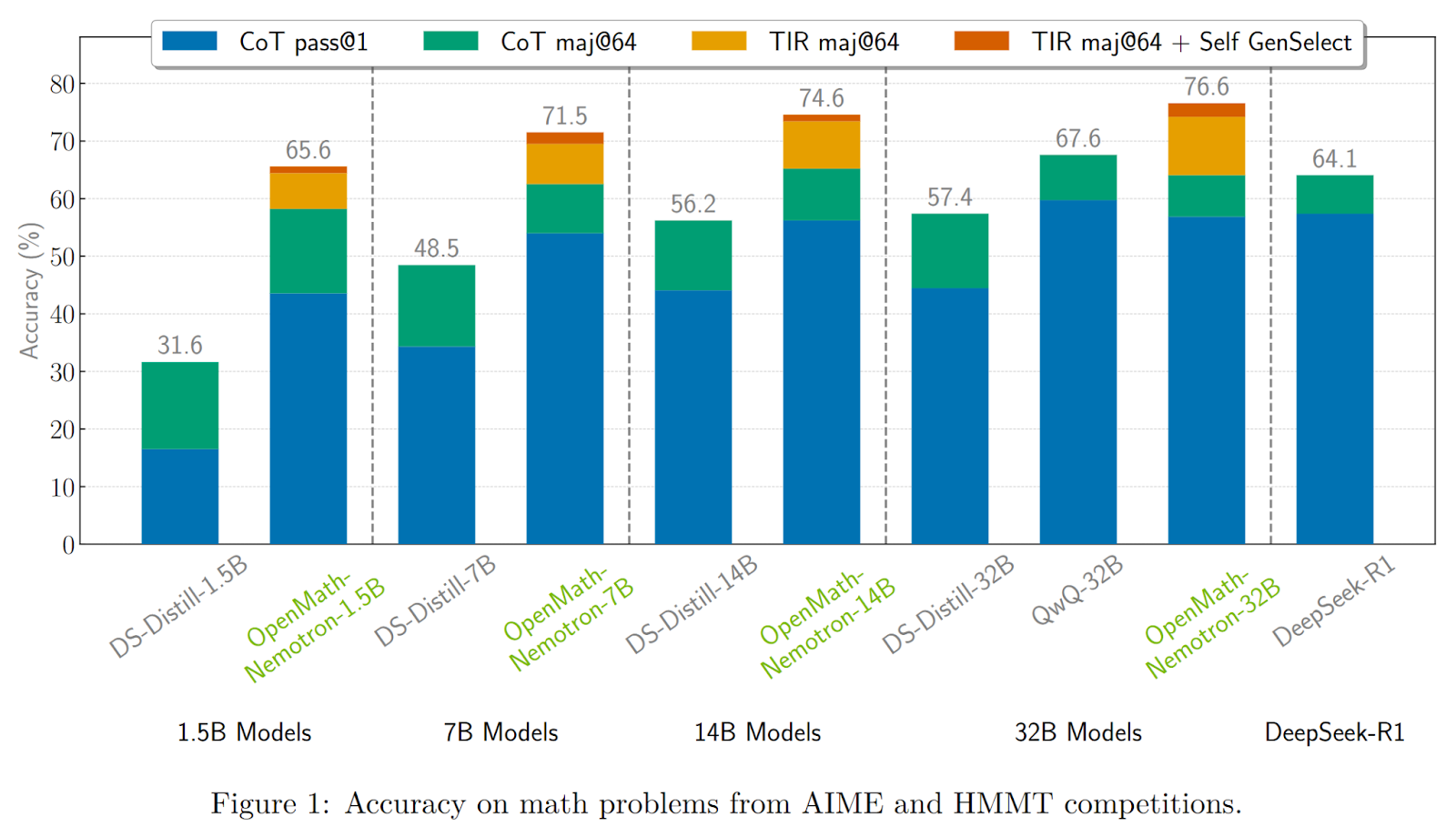
NVIDIA AI Launches OpenMath-Nemotron Models: Transforming Mathematical Reasoning
Introduction
NVIDIA has recently unveiled two advanced AI models, OpenMath-Nemotron-32B and OpenMath-Nemotron-14B-Kaggle, which excel in mathematical reasoning. These models have not only secured first place in the AIMO-2 competition but have also set new benchmarks in the field of AI-driven mathematical problem-solving.
The Challenge of Mathematical Reasoning in AI
Mathematical reasoning poses significant challenges for AI systems. Traditional language models often struggle with complex mathematical problems that require deep understanding and structured logical deductions. This gap has led researchers to develop specialized models that focus on enhancing mathematical capabilities through targeted training and fine-tuning.
Overview of OpenMath-Nemotron Models
OpenMath-Nemotron-32B
The flagship model, OpenMath-Nemotron-32B, features 32.8 billion parameters and is optimized for performance using BF16 tensor operations. It has been fine-tuned on the OpenMathReasoning dataset, which includes challenging problems from prestigious mathematical competitions. This model has achieved state-of-the-art results on various benchmarks, including:
- American Invitational Mathematics Examination (AIME) 2024 and 2025
- Harvard–MIT Mathematics Tournament (HMMT) 2024-25
- Harvard–London–Edinburgh Mathematics Exam (HLE-Math)
In its tool-integrated reasoning (TIR) configuration, it boasts an impressive average pass rate of 78.4% on AIME24.
OpenMath-Nemotron-14B-Kaggle
The OpenMath-Nemotron-14B-Kaggle model, with 14.8 billion parameters, was specifically fine-tuned for competitive performance and played a crucial role in NVIDIA’s success in the AIMO-2 Kaggle competition. This model demonstrated remarkable adaptability, achieving a pass rate of 73.7% on AIME24 in CoT mode and 86.7% under GenSelect protocols.
Inference Modes and Flexibility
Both models offer three distinct inference modes:
- Chain-of-Thought (CoT): Generates intermediate reasoning steps.
- Tool-Integrated Reasoning (TIR): Combines reasoning with external tools for enhanced accuracy.
- Generative Solution Selection (GenSelect): Produces multiple candidate solutions and selects the most consistent answer.
This flexibility allows users to choose the best approach based on their specific needs, whether for research or production environments.
Open-Source Accessibility and Integration
NVIDIA has made both models accessible through an open-source pipeline integrated into the NeMo-Skills framework. This allows developers to reproduce data generation, training procedures, and evaluation protocols easily. The models are optimized for NVIDIA GPU architectures, ensuring efficient performance across various hardware platforms.
Key Takeaways
- NVIDIA’s OpenMath-Nemotron series addresses the challenge of enhancing mathematical reasoning in AI.
- The 32B model achieves state-of-the-art accuracy on major benchmarks, offering three inference modes for flexibility.
- The 14B model, fine-tuned for competition, demonstrates high performance with a smaller parameter footprint.
- Both models are fully reproducible and optimized for NVIDIA GPUs, facilitating low-latency deployments.
Potential Applications
These models can be applied in various fields, including:
- AI-driven tutoring systems
- Academic competition preparation tools
- Scientific computing workflows requiring formal reasoning
Future Directions
Future developments may include expanding capabilities to advanced university-level mathematics, integrating multimodal inputs, and enhancing compatibility with symbolic computation engines.
Conclusion
NVIDIA’s OpenMath-Nemotron models represent a significant advancement in AI’s ability to tackle complex mathematical reasoning. By providing flexible, high-performance solutions, these models open new avenues for applications in education, research, and beyond. As businesses look to integrate AI into their operations, leveraging such advanced tools can lead to improved efficiency and innovation.