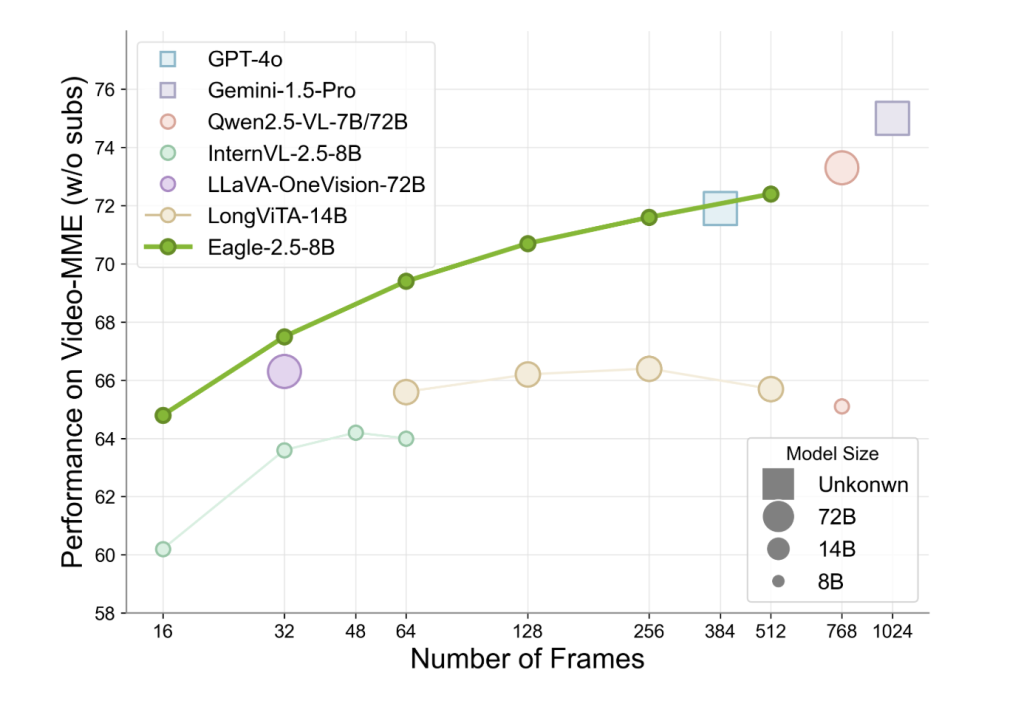
NVIDIA AI’s Eagle 2.5: Advancing Long-Context Multimodal Understanding
Introduction to Long-Context Multimodal Models
Recent advancements in vision-language models (VLMs) have significantly improved the integration of image, video, and text data. However, many existing models struggle to handle long-context multimodal information, such as high-resolution images or lengthy video sequences. These challenges often lead to performance issues, inefficient memory use, and a decline in the quality of semantic details. To overcome these limitations, innovative strategies in data sampling, training, and evaluation are essential.
Introducing Eagle 2.5
NVIDIA’s Eagle 2.5 represents a breakthrough in the field of long-context multimodal learning. This model not only accommodates longer input sequences but also demonstrates consistent performance improvements as input size increases. Designed for comprehensive image and video understanding, Eagle 2.5 targets applications where the complexity of long-form content is vital.
Performance and Efficiency
With just 8 billion parameters, Eagle 2.5 achieves impressive results on established benchmarks. For instance, it scores 72.4% on Video-MME with a 512-frame input, competing closely with larger models like Qwen2.5-VL-72B and InternVL2.5-78B. This success is notable as it does not rely on task-specific compression methods, endorsing a generalist design approach.
Training Strategy: Context-Aware Optimization
The success of Eagle 2.5 is driven by two key training strategies: information-first sampling and progressive post-training.
Information-First Sampling
This strategy emphasizes the retention of essential visual and semantic content. It employs an innovative Image Area Preservation (IAP) technique, which maintains over 60% of the original image area while minimizing distortions. Additionally, Automatic Degradation Sampling (ADS) adjusts the proportion of visual and textual inputs based on context length, ensuring a balanced representation of data.
Progressive Post-Training
This method gradually increases the model’s context window, allowing for a smooth transition through stages of varying token lengths (32K, 64K, and 128K). By doing so, the model is less likely to overfit to a specific context range, resulting in stable performance across diverse scenarios.
Innovative Training Data: Eagle-Video-110K
A pivotal aspect of Eagle 2.5’s effectiveness lies in its training data pipeline, which combines open-source materials with a custom dataset known as Eagle-Video-110K. This dataset supports comprehensive video comprehension through a dual annotation strategy.
Top-Down and Bottom-Up Approaches
The top-down method utilizes human-annotated chapter metadata and GPT-4-generated captions. The bottom-up approach generates question-answer pairs for short clips, incorporating temporal and textual anchors to enhance spatial-temporal awareness. This diverse dataset promotes narrative coherence and provides granular annotations, enriching the model’s understanding of complex temporal data.
Performance Metrics and Benchmarking
Eagle 2.5-8B has shown solid performance across various video and image tasks, achieving scores like 74.8 on MVBench and 94.1 on DocVQA. These metrics illustrate the model’s robust capabilities in both domains. Studies indicate that the model’s sampling strategies significantly influence its performance, particularly in high-resolution tasks.
Conclusion
NVIDIA’s Eagle 2.5 exemplifies a sophisticated approach to long-context vision-language modeling. By focusing on preserving contextual integrity and employing innovative training strategies, Eagle 2.5 achieves competitive performance without the need for extensive model scaling. This positions it as a vital advancement for developing AI systems capable of complex multimodal understanding in real-world applications.
Next Steps for Businesses
- Explore how AI can transform your business operations by identifying tasks suitable for automation.
- Monitor key performance indicators (KPIs) to assess the impact of AI on your business.
- Select adaptable AI tools that align with your specific objectives.
- Start with a pilot project, analyze its results, and scale AI deployment accordingly.
For guidance on integrating AI into your business, feel free to contact us at hello@itinai.ru. Connect with us on Telegram, X, and LinkedIn for more insights.