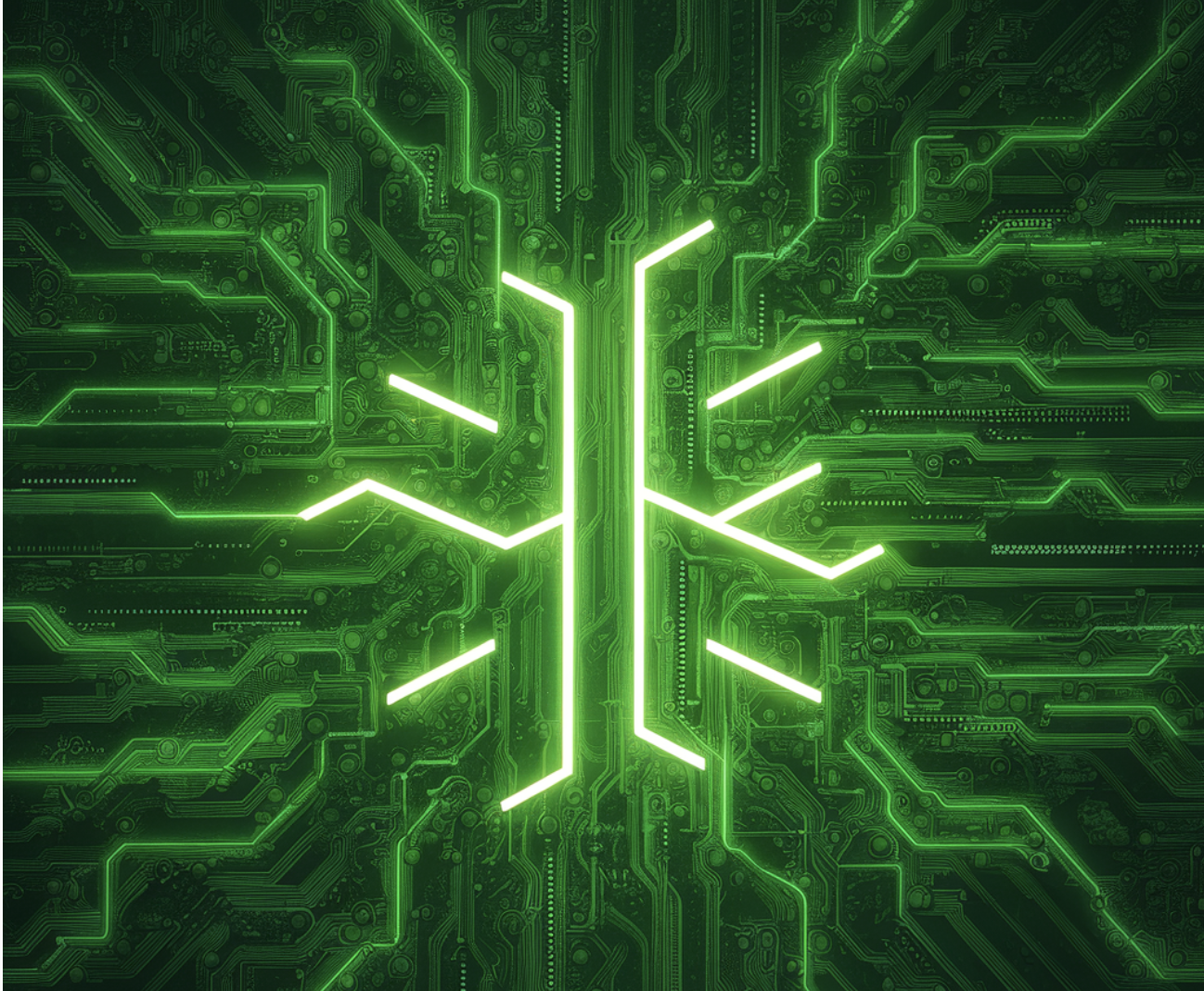
Accelerating Generative AI Inference Speed with NVIDIA TensorRT Model Optimizer
Generative AI, while powerful, faces challenges with slow inference speed in real-world applications. This impacts user experiences, turnaround times, and scalability. NVIDIA addresses these challenges with the TensorRT Model Optimizer, offering advanced techniques for model optimization and accelerated inference.
Model Optimization Techniques
NVIDIA’s TensorRT Model Optimizer introduces post-training quantization (PTQ) and sparsity techniques to reduce memory footprints and accelerate inference while maintaining accuracy. This includes methods like filter pruning, channel pruning, and advanced calibration algorithms for accurate quantization.
Practical Value
By leveraging the TensorRT Model Optimizer, developers can reduce model complexity, accelerate inference, and preserve accuracy. For example, INT4 AWQ can provide significant speedups, and Quantization Aware Training (QAT) enables 4-bit floating-point inference without compromising accuracy.
Performance Improvements
The Model Optimizer has been evaluated on benchmark models, demonstrating substantial speedups in inference. For instance, INT4 AWQ showed a 3.71x speedup compared to FP16 on a Llama 3 model, and INT8 and FP8 produced images with almost the same quality as FP16 while speeding up inference by 35 to 45 percent.
Practical AI Solution
For companies looking to leverage AI, the AI Sales Bot from itinai.com/aisalesbot offers practical automation for customer engagement across all stages of the customer journey, redefining sales processes and customer interactions.
AI Integration Guidance
For companies seeking to integrate AI solutions, it is essential to identify automation opportunities, define measurable KPIs, select suitable AI tools, and implement AI initiatives gradually. For AI KPI management advice and insights into leveraging AI, connect with us at hello@itinai.com or stay tuned on our Telegram t.me/itinainews or Twitter @itinaicom.