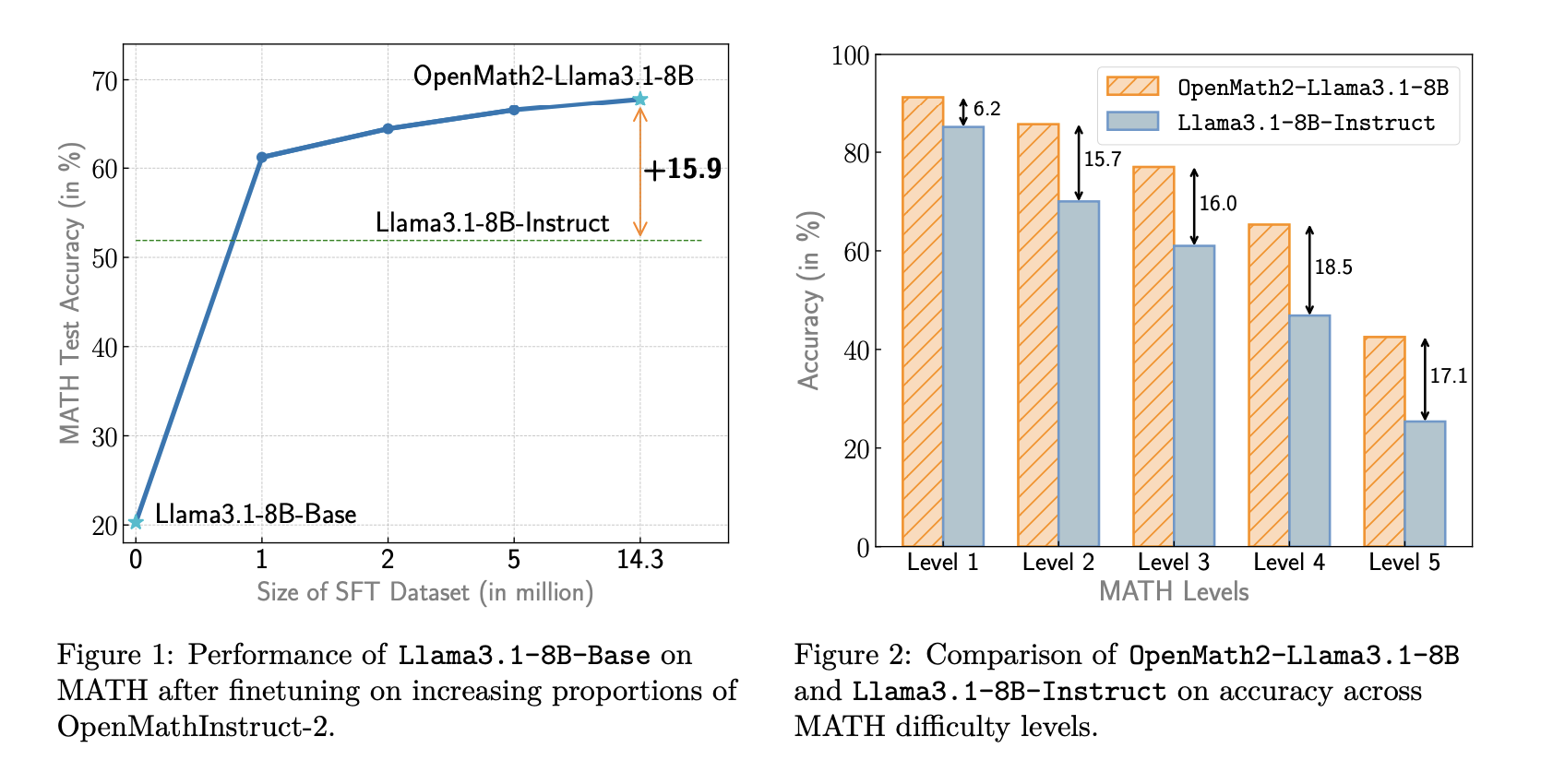
Practical Solutions and Value of AI in Mathematical Reasoning
Enhancing Mathematical Reasoning Abilities
Develop datasets like NuminaMath and Skywork-MathQA with competition-level problems and diverse augmentation techniques.
Focus on complicating and diversifying queries with datasets like MuggleMath and MetaMathQA.
Improve model accuracy by expanding existing datasets such as MATH and GSM8K.
Tool-Integrated Methods
Utilize approaches like Program of Thoughts (PoT) to combine text and programming language for problem-solving.
Create datasets like OpenMathInstruct-1 and InfinityMATH for code-interpreter solutions and programmatic mathematical reasoning.
Key Innovations in Dataset Creation
Employ open-weight models for dataset creation under a permissive license, enhancing accessibility and transparency.
Provide insights into critical aspects of dataset creation, including the impact of data quality and training effectiveness.
Ensure result accuracy through a decontamination process using an LLM-based pipeline.
Impact of OpenMathInstruct-2
Generate synthetic math instruction tuning data using Llama3.1 models for superior performance.
Thorough decontamination process ensures test set integrity in the dataset.
Demonstrates impressive results across various mathematical reasoning benchmarks.
Contributions to Mathematical Reasoning
Advance understanding of dataset construction through comprehensive dataset release and high-performing models.
Establish best practices for developing future mathematical reasoning datasets and models.
Ensure accurate benchmark evaluations through rigorous decontamination processes.
Evolve Your Company with AI
Identify automation opportunities and define KPIs for measurable impacts on business outcomes.
Select AI solutions that align with your needs and implement gradually starting with a pilot.
Connect with us for AI KPI management advice and stay tuned for continuous insights into leveraging AI.